1. Introduction
The novel coronavirus 2019 (COVID-19) has spread worldwide (
World Health Organization (WHO), 2020). COVID-19 is a disease with the core feature of acute respiratory distress syndrome (ARDS), and the commonest symptoms of fatigue, cough, and fever, as well as headache, hemoptysis, and dyspnea as other manifestations (
Singhal, 2020).
COVID-19 can even spread to the central nervous system (CNS) through the respiratory tract concerning its neuroinvasive potential (
Zhou et al., 2020). Although the routes of COVID-19 entering the brain are still unclear, it seems that the virus can induce an intracranial cytokine storm leading to depression of the blood-brain barrier by inducing direct viral invasion (
Karuppan et al., 2021). COVID-19 can also enter the cerebral blood flow by passing through the circulation (
Al‐Sarraj et al., 2021). Another route for the virus to enter the brain may be through the olfactory bulb (neuronal pathways) (
Wu et al., 2020) or directly enter the brain via olfactory vessels or cervical lymphatic nodes (lymphatic pathway) (
Bostancıklıoğlu, 2020). According to the results, human ocular tissue can provide a potent pathway to pass the severe acute respiratory syndrome coronavirus 2 (SARS-CoV-2) and cause infection (
Ma et al., 2020). Coronavirus infection is associated with neurological symptoms categorized as peripheral nervous system (i.e. neuralgia, hyposmia, hypogeusia, and hypoplasia), CNS (i.e. ataxia, dizziness, acute cerebrovascular disease, epilepsy, headache, and impaired consciousness), and musculoskeletal symptoms (
Matías-Guiu et al., 2020). A study conducted on 214 adult patients affected by COVID-19 reported that 36.4% had neurological symptoms indicating potential neurological symptoms of COVID-19 (
Mao et al., 2020).
The evidence shows that organs with increased expression of angiotensin-converting enzyme II (ACE 2) at the cell level should potentially be considered at high risk for COVID-19 infection; e.g. lung, kidney, and heart. Severe acute respiratory syndrome coronavirus 2 (SARS-CoV-2) binds to a G-protein coupled receptor, ACE 2, to enter the cells (
Zhang et al., 2020). ACE 2 and its receptors are also found in the brain, especially in CNS neurons, including excitatory and inhibitory ones and glial cells, mainly astrocytes and oligodendrocytes (
Chen et al., 2021). The role of ACE 2 in the CNS is beyond the regulation of cardiovascular function; ACE2 is involved in cognition, behavior, and locomotion in the adult brain. Wang et al. reported that the knockout of the ACE 2 caused a significant cognitive impairment in mice. Alterations in the activity of ACE 2, as a viral receptor, lead to cognitive impairment and neuronal dysfunction (
Wang et al., 2016). In particular, ACE 2 contributes to different brain functions, such as depression, stress, memory consolidation, and cerebroprotection (
Guimond & Gallo-Payet, 2012). In humans, ACE2 is expressed in the posterior cingulate cortex, olfactory bulb areas, and middle temporal gyrus, although the number of cells expressing ACE 2 in the prefrontal cortex and the hippocampus was zero and very few, respectively (
Chen et al., 2020). Due to the spread of the ACE 2 receptor through neural networks of the brain, useful information was obtained by a whole-brain scan about the COVID-19 cognitive impairment mechanism.
The brain networks’ intrinsic connectivity can be studied by the resting-state functional magnetic resonance imaging (rsfMRI) that maps blood oxygen fluctuations depending on signals that are temporally synchronous, spatially distributed, and have a spontaneous low frequency (<0.08 Hz) (
Mantini et al., 2007). More information can be obtained using rfMRI regarding the intrinsic functional organization of the brain (
Fox & Greicius, 2010).
The present study was conducted to evaluate the whole-brain neuronal circuits in patients with COVID-19 using rfMRI and to find its correlation with cognitive impairment using the Montreal cognitive assessment (MoCA), which is a reliable brief test to detect mild cognitive impairments. This instrument includes the main cognitive domains, i.e. visuospatial ability, language, episodic memory, executive functions, orientation, and attention. This is the first study to investigate cognitive impairments in patients with COVID-19 in terms of rsfMRI characteristics. The study examines the alteration of the neural circuits of the brain in the group of patients with COVID-19 compared to the healthy controls.
2. Methods and Methods
Participants
The current study was conducted with a cross-sectional design in a teaching hospital in Shahroud City, Iran, on subjects selected by the convenience sampling method. Informed consent was obtained from all the subjects to participate in the study, then they underwent an interview and MoCA test by a psychologist, and fMRI scanning by a radiologic technologist.
The study was conducted on 29 patients with COVID-19 (the COV group) and 20 healthy controls (the CON group). The groups underwent similar cognitive assessment and fMRI procedures. Both groups were matched by demographic characteristics, including age, gender, socioeconomic status, smoking habit, body mass index (BMI), and underlying systemic disease, i.e. diabetes, hypertension, blood diseases, such as any type of anemia, and chronic pulmonary and cardiovascular (coronary and cyanotic heart conditions) diseases. The inclusion criteria included a positive reverse transcription polymerase chain reaction (RT-PCR) test for COVID-19 and native Persian speaking. The exclusion criteria included contraindication for MRI (pacemakers, metal implants, etc.), history of neurological diseases, including neurocognitive impairment or dementia, psychological problems, use of neuropsychological drugs, and other brain pathology. The baseline characteristics, including demographics, educational level, etc. were collected by a questionnaire.
Montreal cognitive assessment (MoCA)
In the current study, the Persian version of MoCA was employed to evaluate cognitive function. This questionnaire assessing more cognitive domains is composed of more complex skills and thus is more sensitive to mild cognitive impairments compared to the mini-mental state examination. The MoCA, including executive functions (following the numbers, letters, words, and abstracts), attention, concentration, delayed recalling, language (naming and sentence repetition), orientation, visuoconstructional skills (drawing a cube or watch), calculations, and conceptual thinking, was used to assess cognitive domains in the subjects. The overall instrument score is 30; scores ≥26 are considered normal. One point is added to the overall score of the ones with the education level below the high school diploma (
Kang et al., 2018). Emsaki et al. provided the Persian version of MoCA by translating the original version and testing it psychometrically (Cronbach’s α: 0.77, concurrent validity: 0.79, sensitivity: 0.85, and specificity: 0.90) (
Emsaki et al., 2011). The MoCA was performed by a research assistant (clinical psychologist) in the same environment as participants in both COVID-19 (COV) and control (CON) groups.
Paraclinical assessments
The results of laboratory tests, including hematologic and serologic tests, were extracted from patients’ medical profiles. All the routine blood tests, such as complete blood cell count, platelet count, hemoglobin (Hb), hematocrit, blood urea nitrogen, creatinine, erythrocyte sedimentation rate (ESR), C-reactive protein (CRP), alanine aminotransferase, aspartate aminotransferase, alkaline phosphatase, sodium, potassium, calcium, phosphorus, blood sugar levels, prothrombin time, partial thromboplastin time, and bilirubin tests, as well as the result of chest x-ray and chest computed tomography (CT) scan, were recorded.
Image acquisition
A 1.5T Avanto MRI scanner (Siemens, Germany) with an 18-channel parallel imaging head coil was used to obtain MR images. Resting-state fMRI data and oblique axial sections (time for echo (TE): 45 ms; Time for repetition (TR): 3300 ms; in-plane resolution=3×3 mm2, slice thickness: 3.5 mm; flip angle: 90°) were acquired for 10 minutes using gradient-echo echo planar imaging sequence, containing 300 imaging volumes with time point=200, field of view (FOV)=157×15.7 cm, and matrix=64×64. The subjects closed their eyes while they were awake during scanning. T1 structural data were acquired using three-dimensional magnetization-prepared rapid-gradient-echo in sagittal view. Data acquisition parameters for T1 imaging were as follows: TR=2000 ms, TE=2.9 ms, matrix size=256×256, and flip angle=8°.
Functional magnetic resonance imaging (fMRI) data preprocessing
All steps of data preprocessing were performed using FMRIB software library tools, version 6. The correction of head movements was performed rigidly using a motion correction tool and using FLIRT commands. Separation of the brain from the skull was performed on the volumes of motion-corrected blood oxygenation level-dependent (BOLD) images as implemented in the brain extraction tool algorithm. Confirmation of this process was done by visual inspection of the obtained results. Also, the affine algorithm in FMRIB’s linear image registration tool (FLIRT) format was used to register different volumes of fMRI images on 3D T1 images of each person and also to transfer 3D T1 images to the standard space of MNI152. Images were converted to 2 mm voxels using a softener filter with a 5 mm full width at half maximum. Parameters related to the head movements of the two groups, both absolute and relative, were not significantly different from each other. Also, the absolute and relative amount of head movement in all subjects was less than the image voxel size (
Jenkinson et al., 2002).
Analysis and processing of rest-fMRI
Independent components (IC) analysis was used to process rs-fMRI images (
Abou Elseoud et al., 2011). For IC analysis, we used the multivariate exploratory linear optimized decomposition into IC (MELODIC) tool implemented in the FMRIB software library (
Beckmann & Smith, 2004). For this purpose, the multi-session temporal concatenation tool in multivariate exploratory linear optimized decomposition into independent components (MELODIC) and the preprocessing and steps required for group data analysis in this tool were used. Spatial independent components analysis using 60 independent component maps was used to detect resting-state networks from the control group. The reason for choosing the control group is that experience shows that using a group analysis consisting of both control and patient groups provides maps of the average of the two groups, which reduces the sensitivity of differences between the two groups in the next step, dual regression analysis (
Abou Elseoud et al., 2011). Data were also normalized to variance (variance normalization). IC mappings were then thresholded using a hypothesis test based on the fit of a Gaussian mixed model on the intensity distribution of voxels on spatial mappings and false-discovery rate control at P<0.5 (
Abou‐Elseoud et al., 2010;
Beckmann & Smith, 2004). Twenty-three rest networks were identified as classical anatomical and functional networks based on ocular examination by an experienced neuroradiologist using criteria previously described (
Abou‐Elseoud et al., 2010;
Kiviniemi et al., 2009;
Smith et al., 2009). Supplementary
Table 1 shows the 23 resting-state networks mentioned above.
The analysis was performed to investigate the intergroup differences using the dual regression technique in the FMRIB software library, which allows the voxel-like comparison of resting-state fMRI images (
Abou‐Elseoud et al., 2010;
Filippini et al., 2009;
Littow et al., 2010). This step includes using group-independent component analysis spatial maps to fit a linear model on various fMRI datasets, leading to matrices (time change matrices) that describe temporal dynamics, and using these time course matrices to estimate the spatial mappings of each individual. Independent components analysis patterns were selected for dual regression from sound control data. Dual regression analysis was performed with variance normalization and non-normalization (dual regression command with des norm-option variable equal to 1 or 0, respectively) because the results have a different emphasis on the location and amplitude of the BOLD signal depending on the normalization (
Allen et al., 2014;
Allen et al., 2012). With variance normalization, dual regression shows differences in both resting-state network activity and spatial dispersion. Without normalization, only spatial changes are reflected.
As a statistical analysis, the maps of different components on different people are integrated into 4D files (one file for each original Independent components analysis mapping) and tested in a box-by-box manner to examine statistically significant differences between groups. This is done using the FMRIB software library of a non-parametric random permutation test with 5000 permutations using an enhanced free threshold clustering technique to control multiple comparisons (
Nichols & Holmes, 2002). The difference hypothesis between the two groups was calculated using a threshold of P<0.05 by changing the vaccine to vaccine in the randomized FMRIB software library tool. To control the comparison of several detected differences between different components of IC analysis, the inter-IC concatenation technique was used (
Abou Elseoud et al., 2014). After 5000 times permutation, the resulting datasets were separated using the fslroi command and the threshold of P<0.05 to correct type 1 error for the selected independent components. As a new step, a gray matter regressor image was introduced into the model in the same way so that it was concatenated in the y-direction to control the gray matter differences using the vxl and vxf options in the randomize FMRIB software library.
The Juelish histological atlas is embedded in the FMRIB software library, and the Harvard-Oxford cortical and subcortical atlases were used in the FMRIB software library to identify the anatomical features of the probabilistic independent components analysis maps obtained. Finally, fslstats and fslmaths tools were used to calculate the number of non-zero voxels in the selected difference maps and their t-score values.
Figure 1 shows the applied pipeline for rest-fMRI data processing and analysis.
Statistical analysis
Data from quantitative variables were expressed as Mean±SD and categorical variables as frequency and percentage. Data with normal distribution were compared using a t-test, and data with non-normal distribution with the Mann-Whitney U test to compare continuous variables. The correlation between quantitative variables was examined by the Pearson correlation coefficient or non-parametric Spearman test. The relationship of cognitive indices with MRI characteristics was assessed using a multiple linear regression model. Data were analyzed in SPSS software, version 20. The significance level was<0.05.
3. Results
Demographics, neuropsychological tests, and fMRI
Table 1 presents the demographic, MoCA, and fMRI measures of patients with COVID-19 and healthy controls.
Suplememtary
Table 2 presents more detailed characteristics of COVID-19 patients. The two groups were matched by age, gender, and education level. According to the results of MoCA, the neurocognitive performance was significantly lower in the COV group than in healthy controls (P<0.001). The Mean±SD MoCA score of the patients was 20.41±6.07. Among patients, 5(17.2%) were normal and the remaining 24(82.8%) had mild cognitive impairment. Moreover, patients with COVID-19 had impairments in visual acuity, memory, attention, speech, abstraction, information, and names.
The whole-brain functional connectivity was detected by rs-fMRI in all the 29 patients using data obtained from functional MRI. Then, the 23 brain regions of each patient were compared to obtain functional connectivity. The normal resting-state networks of the classical resting-state networks (RNS) groups identified anatomically and functionally are shown in a red-yellow color FMRIB Software Library (FSL), based on the 2.5< z-score <8 thresholds (
Figure 2).
Table 2 presents a significant increase in the functional connectivity of patients with COVID-19 compared to controls in terms of default mode network (DMN) and dorsal attention network (DAN), based on IC results.
Table 3 presents the results of ICs with a significant decrease in functional connectivity in V1 dorsal L and BA25 of patients with COVID-19 (
Figure 3).
Correlations between functional connectivity, neuropsychological variables, and paraclinical assessments
Tables 4,
5, and
6 present detailed information about the comparison of paraclinical data of participants with normal and abnormal MoCA scores, such as their association with rs-fMRI data, as well as Hb, ESR, CRP, and blood oxygen levels.
According to the obtained results, a significant correlation was found between the rs-fMRI data and MoCA subdomains, except memory and speech. Regarding the correlation between functional brain regions,
Table 7 presents alternations in functional connectivity and scores of MoCA subdomains.
4. Discussion
To our knowledge, this study was conducted to assess cognitive impairments and investigate the whole-brain functional connectivity using rs-fMRI in patients with COVID-19 compared to healthy controls. The COV group obtained a lower score in the MoCA test, increased functional connectivity in the DMN (IC00) and DAN (IC08), and decreased functional connectivity in the V1 dorsal L (IC14) and BA25 (IC38) at the baseline compared to healthy controls (
Figure 4). Cognitive impairment was mainly manifested in visual acuity, attention, abstraction, information, and name domains, while speech and memory had no deficits based on the MoCA results. Finally, the functional connectivity of these brain regions showed a correlation with the total score of MoCA. It should be noted specific factors that may affect the final analysis results, including the mental status of the subject, the physiology of the BOLD signal, and the methodology of the analysis approach. A study on the pattern of cognitive deficits during the post-critical acute stage of severe COVID-19 reported mild to severe deficits at MoCA with extensive cognitive impairment in memory, attention, executive, and visuospatial functions, but relatively preserved language and orientation dysfunction (
Beaud et al., 2021). Blazhenets et al. assessed MoCA and PET scans in 8 COVID-19 patients at the subacute and chronic stages and found a residual impairment still measurable in some patients six months after the infection of COVID-19 (
Blazhenets et al., 2021).
DMN contributes to internal psychological processing. A correlation is observed between external environmental monitoring and internally directed cognition (
Andrews-Hanna et al., 2010;
Buckner et al., 2008); its activity decreases during a variety of cognitive tasks requiring external perceptual attention (
Greicius et al., 2003). In contrast, DAN contributes to controls that are goal- and attention-oriented (
Scalf et al., 2014). It has a significant correlation with externally directed cognition (
Corbetta & Shulman, 2002), and its activity increases during cognitive tasks focusing on external visuospatial attention (
Miller & Buschman, 2013). DMN and DAN show an anticorrelated activity pattern in resting-state and task studies (internal and external cues). In addition, when the system works properly, they show a competitive relationship that represents a cerebral mechanismsupporting cognitive functions, switching focus between internal (supported by DMN) and external cues (supported by DAN) (
Esposito et al., 2018b;
Franzmeier et al., 2017). The details of how the DMN and DAN interact remain unknown. However, the current study results showed that both DMN and DAN increased functional connectivity. Therefore, no anticorrelation was observed between them. It seems that a decrease in DMN-DAN anticorrelation is part of the normal process of aging (
Esposito et al., 2018a); hence, any abnormality in this process can be associated with cognitive dysfunction and increase the risk of Alzheimer’s disease (
Zhu et al., 2016) and mild cognitive impairment (
Franzmeier et al., 2017;
Zhu et al., 2016). As previously mentioned, in patients with COVID-19, the activity of the DAN network increased while it was expected to decrease due to the cognitive deficit based on MoCA results. The patterns of DAN and DMN activity may be related to a compensatory mechanism against cognitive impairment in patients with COVID-19.
Both CRP and ESR, as markers of inflammation, increased in patients with COVID-19. Also, evidence of the excessive immune response following the induction of cytokine storm by the virus has been reported (
Nile et al., 2020). These patterns were also observed in ARDS as a systemic inflammatory disease with some symptoms similar to those of COVID-19.
As several studies reported (
Li et al., 2020;
Singhal, 2020), in the current study, the MoCA score was also negatively correlated with ESR and CRP levels. Marsland et al. showed a relationship between interleukin-6, a systemic inflammation indicator, and alterations in DMN activity (
Marsland et al., 2017). Moreover, the DMN may contribute to the incidence of psychiatric and neurological disorders correlating with systemic inflammations, e.g. anxiety, and mood disorders, characterized by emotion regulation impairments and raised systemic inflammation (
Labrenz et al., 2019;
Rabany et al., 2017). Cognitive impairments are reported in survivors of ARDS even several years after discharge (
Hopkins et al., 2005). Patients with ARDS may experience prolonged hypotension, metabolic abnormalities, and hypoxemia, predisposing them to injuries in the peripheral and central nervous systems, followed by neurologic and cognitive impairments (
Hopkins et al., 2006;
Hopkins et al., 2004). In contrast, no significant relationship was found between blood oxygen levels, MoCA scores, and rs-fMRI data in the current study. One possible explanation for inconsistent findings is our small sample size. On the other hand, ARDS survivors experience prolonged psychiatric symptoms after discharge. Systematic reviews reported 17% to 43% depression, 23% to 48% anxiety, and 8% to 35% post-traumatic stress disorder (PTSD) symptoms in ARDS survivors. Studies examine the impact of stress related to COVID-19. Patients with COVID-19 experience prolonged stress due to fear of death. Therefore, COVID-19 has already led to various stress-related disorders, including anxiety and posttraumatic stress disorder (
Tucker & Czapla, 2020). Our data consistent with previous studies showed that DMN activity may contribute to the incidence of psychiatric disorders correlating with anxiety (
Vicentini et al., 2017).
Based on the obtained results, a decrease of functional connectivity in patients with COVID-19 was observed in the occipital cortex, including bilateral lingual gyri and left middle occipital gyrus located in the visual network, which contributes to visual memory and visuospatial function processing (
Cai et al., 2017). The potential brain bases for the impaired multiple cognitive domains were determined based on the results of COVID-19, which were consistent with the results of a study reporting neurological symptoms in patients with COVID-19 (
Li et al., 2020). A significant correlation was observed between the occipital lobe activities and functional connectivity, visual hallucination, visual memory, and visuospatial function (
Yao et al., 2016). Hence, the occipital cortex functional impairments may play a role in damage to multiple cognitive domains, especially in terms of visual cognition, in patients with COVID-19.
In terms of the study limitations, first, the sample size was small. Second, due to the high infectivity and fatality of COVID-19 and its psychosocial impacts, both the patients and researchers experienced a huge amount of stress. Third, brain function impairments and recovery should be investigated in patients with COVID-19 via longitudinal studies.
5. Conclusion
In conclusion, the study results indicated significant clinical changes in the resting-state functional connectivity in patients with COVID-19. According to MoCA results, in such patients, functional connectivity within different cognitive networks decreased, and cognitive impairments occurred. Further longitudinal studies with larger sample sizes are required to obtain more conclusive results and understand the rsfMRI of cognitive networks and changes in neural circuits.
Ethical Considerations
Compliance with ethical guidelines
This study was completed concerning the Declaration of Helsinki and the ethical guidelines for medical and health research established by the Ministry of Health and Medical Education and Ministry of Science, Research and Technology, Iran. Also the Ethics Committee of Shahroud University of Medical Sciences approved this study (Code: IR.SHMU.REC.1398.174). All subjects provided their informed written consent to participate in the present study.
Funding
This research was financially supported by Shahroud University of Medical Sciences (Grant No.: 98133).
Authors' contributions
Conceptualization: Mohammad Niroumand Sarvandani and Javad Sheikhi Koohsar; Methodology: Raheleh Rafaiee, and Maryam Saeedi; Data analysis: Raheleh Rafaiee and Javad Sheikhi Koohsar; Investigation: Hamed Ghazvini and Seyedeh Masoumeh Seyedhosseini Tamijani; Writing the original draft: Raheleh Rafaiee and Mohammad Niroumand Sarvandani; Writing, review, editing and resources: Hossein Sheibani and Maryam Saeedi; Funding acquisition and supervision: Hossein Sheibani.
Conflict of interest
The authors declared no conflict of interest.
Acknowledgments
The authors thank all the participants in this project. Also, they thank the manager of Imam Hossein Hospital of Shahroud City for their cooperation.
References
Abou-Elseoud, A., Starck, T., Remes, J., Nikkinen, J., Tervonen, O., & Kiviniemi, V. (2010). The effect of model order selection in group PICA. Human Brain Mapping, 31(8), 1207–1216. [DOI:10.1002/hbm.20929] [PMID] [PMCID]
Abou Elseoud, A., Littow, H., Remes, J., Starck, T., Nikkinen, J., & Nissilä, J., et al. (2011). Group-ICA model order highlights patterns of functional brain connectivity. Frontiers in Systems Neuroscience, 5, 37. [DOI:10.3389/fnsys.2011.00037] [PMID] [PMCID]
Abou Elseoud, A., Nissilä, J., Liettu, A., Remes, J., Jokelainen, J., & Takala, T., et al. (2014). Altered resting-state activity in seasonal affective disorder. Human Brain Mapping, 35(1), 161–172. [DOI:10.1002/hbm.22164] [PMID] [PMCID]
Al-Sarraj, S., Troakes, C., Hanley, B., Osborn, M., Richardson, M. P., & Hotopf, M., et al. (2021). Invited review: The spectrum of neuropathology in COVID-19. Neuropathology and Applied Neurobiology, 47(1), 3–16.[DOI:10.1111/nan.12667] [PMID]
Allen, E. A., Damaraju, E., Plis, S. M., Erhardt, E. B., Eichele, T., & Calhoun, V. D. (2014). Tracking whole-brain connectivity dynamics in the resting state. Cerebral Cortex, 24(3), 663–676. [DOI:10.1093/cercor/bhs352] [PMID] [PMCID]
Allen, E. A., Erhardt, E. B., Wei, Y., Eichele, T., & Calhoun, V. D. (2012). Capturing inter-subject variability with group independent component analysis of fMRI data: A simulation study. NeuroImage, 59(4), 4141–4159. [DOI:10.1016/j.neuroimage.2011.10.010] [PMID] [PMCID]
Andrews-Hanna, J. R., Reidler, J. S., Sepulcre, J., Poulin, R., & Buckner, R. L. (2010). Functional-anatomic fractionation of the brain's default network. Neuron, 65(4), 550–562.[DOI:10.1016/j.neuron.2010.02.005] [PMID] [PMCID]
Beaud, V., Crottaz-Herbette, S., Dunet, V., Vaucher, J., Bernard-Valnet, R., & Du Pasquier, R., et al. (2021). Pattern of cognitive deficits in severe COVID-19. Journal of Neurology, Neurosurgery, and Psychiatry, 92(5), 567–568. [DOI:10.1136/jnnp-2020-325173] [PMID] [PMCID]
Beckmann, C. F., & Smith, S. M. (2004). Probabilistic independent component analysis for functional magnetic resonance imaging. IEEE Transactions on Medical Imaging, 23(2), 137–152. [DOI:10.1109/TMI.2003.822821] [PMID]
Blazhenets, G., Schroeter, N., Bormann, T., Thurow, J., Wagner, D., & Frings, L., et al. (2021). Slow but evident recovery from neocortical dysfunction and cognitive impairment in a series of chronic COVID-19 patients. Journal of Nuclear Medicine, 62(7), 910-915. [DOI:10.2967/jnumed.121.262128]
Bostancıklıoğlu M. (2020). SARS-CoV2 entry and spread in the lymphatic drainage system of the brain. Brain, Behavior, and Immunity, 87, 122–123. [DOI:10.1016/j.bbi.2020.04.080] [PMID] [PMCID]
Buckner, R. L., Andrews-Hanna, J. R., & Schacter, D. L. (2008). The brain's default network: Anatomy, function, and relevance to disease. Annals of the New York Academy of Sciences, 1124, 1–38. [DOI:10.1196/annals.1440.011] [PMID]
Cai, S., Chong, T., Peng, Y., Shen, W., Li, J., & von Deneen, K. M., et al. (2017). Altered functional brain networks in amnestic mild cognitive impairment: A resting-state fMRI study. Brain Imaging and Behavior, 11(3), 619–631. [DOI:10.1007/s11682-016-9539-0] [PMID]
Chen, R., Wang, K., Yu, J., Howard, D., French, L., & Chen, Z., et al. (2021). The spatial and cell-type distribution of SARS-CoV-2 receptor ACE2 in the human and mouse brains. Frontiers in Neurology, 11, 573095. [DOI:10.3389/fneur.2020.573095] [PMID] [PMCID]
Chen, R., Yu, Jie, Wang, K., Howard, D., French, L., & Chen, Z., et al. (2020). The spatial and cell-type distribution of SARS-CoV-2 receptor ACE2 in human and mouse brain. Applied Neuroimaging, 11; 573095. [DOI:10.3389/fneur.2020.573095]
Corbetta, M., & Shulman, G. L. (2002). Control of goal-directed and stimulus-driven attention in the brain. Nature reviews. Neuroscience, 3(3), 201–215. [DOI:10.1038/nrn755] [PMID]
Emsaki, G., Molavi, H., Chitsaz, A., Abtahi, M, M., & Asgari, K. (2011). [Psychometric properties of the montreal cognitive assessment (MoCA) in parkinson’s disease patients in Isfahan (Persian)]. Journal of Isfahan Medical School, 29(158), 1606-15. [Link]
Esposito, R., Cieri, F., Chiacchiaretta, P., Cera, N., Lauriola, M., Di Giannantonio, M., et al. (2018). Modifications in resting state functional anticorrelation between default mode network and dorsal attention network: Comparison among young adults, healthy elders and mild cognitive impairment patients. Brain Imaging and Behavior, 12(1), 127–141. [DOI:10.1007/s11682-017-9686-y] [PMID]
Filippini, N., MacIntosh, B. J., Hough, M. G., Goodwin, G. M., Frisoni, G. B., & Smith, S. M., et al (2009). Distinct patterns of brain activity in young carriers of the APOE-epsilon4 allele. Proceedings of the National Academy of Sciences of the United States of America, 106(17), 7209–7214. [DOI:10.1073/pnas.0811879106] [PMID] [PMCID]
Fox, M. D., & Greicius, M. (2010). Clinical applications of resting state functional connectivity. Frontiers in Systems Neuroscience, 4, 19. [DOI:10.3389/fnsys.2010.00019] [PMID] [PMCID]
Franzmeier, N., Buerger, K., Teipel, S., Stern, Y., Dichgans, M., & Ewers, M., et al. (2017). Cognitive reserve moderates the association between functional network anti-correlations and memory in MCI. Neurobiology of Aging, 50, 152–162. [DOI:10.1016/j.neurobiolaging.2016.11.013] [PMID]
Franzmeier, N., Göttler, J., Grimmer, T., Drzezga, A., Áraque-Caballero, M. A., & Simon-Vermot, L., et al. (2017). Resting-state connectivity of the left frontal cortex to the default mode and dorsal attention network supports reserve in mild cognitive impairment. Frontiers in Aging Neuroscience, 9, 264. [DOI:10.3389/fnagi.2017.00264] [PMID] [PMCID]
Greicius, M. D., Krasnow, B., Reiss, A. L., & Menon, V. (2003). Functional connectivity in the resting brain: A network analysis of the default mode hypothesis. Proceedings of the National Academy of Sciences of the United States of America, 100(1), 253–258. [DOI:10.1073/pnas.0135058100] [PMID] [PMCID]
Guimond, M. O., & Gallo-Payet, N. (2012). The Angiotensin II Type 2 Receptor in Brain Functions: An update. International Journal of hypertension, 2012, 351758. [DOI:10.1155/2012/351758] [PMID] [PMCID]
Hopkins, R. O., Gale, S. D., & Weaver, L. K. (2006). Brain atrophy and cognitive impairment in survivors of acute respiratory distress syndrome. Brain Injury, 20(3), 263–271. [DOI:10.1080/02699050500488199] [PMID]
Hopkins, R. O., Weaver, L. K., Chan, K. J., & Orme, J. F., Jr (2004). Quality of life, emotional, and cognitive function following acute respiratory distress syndrome. Journal of the International Neuropsychological Society, 10(7), 1005–1017. [DOI:10.1017/S135561770410711X] [PMID]
Hopkins, R. O., Weaver, L. K., Collingridge, D., Parkinson, R. B., Chan, K. J., & Orme, J. F., Jr (2005). Two-year cognitive, emotional, and quality-of-life outcomes in acute respiratory distress syndrome. American Journal of Respiratory and Critical Care Medicine, 171(4), 340–347. [DOI:10.1164/rccm.200406-763OC] [PMID]
Jenkinson, M., Bannister, P., Brady, M., & Smith, S. (2002). Improved optimization for the robust and accurate linear registration and motion correction of brain images. NeuroImage, 17(2), 825–841. [DOI:10.1016/S1053-8119(02)91132-8] [PMID]
Kang, J. M., Cho, Y. S., Park, S., Lee, B. H., Sohn, B. K., & Choi, C. H., et al. (2018). Montreal cognitive assessment reflects cognitive reserve. BMC Geriatrics, 18(1), 261. [DOI:10.1186/s12877-018-0951-8] [PMID] [PMCID]
Karuppan, M. K. M., Devadoss, D., Nair, M., Chand, H. S., & Lakshmana, M. K. (2021). SARS-CoV-2 infection in the central and peripheral nervous system-associated morbidities and their potential mechanism. Molecular Neurobiology, 58(6), 2465–2480. [DOI:10.1007/s12035-020-02245-1] [PMID] [PMCID]
Kiviniemi, V., Starck, T., Remes, J., Long, X., Nikkinen, J., & Haapea, M., et al. (2009). Functional segmentation of the brain cortex using high model order group PICA. Human Brain Mapping, 30(12), 3865–3886. [DOI:10.1002/hbm.20813] [PMID] PMCID]
Labrenz, F., Ferri, F., Wrede, K., Forsting, M., Schedlowski, M., & Engler, H., et al. (2019). Altered temporal variance and functional connectivity of BOLD signal is associated with state anxiety during acute systemic inflammation. NeuroImage, 184, 916–924. [DOI:10.1016/j.neuroimage.2018.09.056] [PMID]
Li, Z., Liu, T., Yang, N., Han, D., Mi, X., Li, Y., Liu, K., Vuylsteke, A., Xiang, H., & Guo, X. (2020). Neurological manifestations of patients with COVID-19: Potential routes of SARS-CoV-2 neuroinvasion from the periphery to the brain. Frontiers of Medicine, 14(5), 533–541. [DOI:10.1007/s11684-020-0786-5] [PMID] [PMCID]
Littow, H., Elseoud, A. A., Haapea, M., Isohanni, M., Moilanen, I., & Mankinen, K., et al. (2010). Age-related differences in functional nodes of the brain cortex - a high model order group ICA study. Frontiers in Systems Neuroscience, 4, 32. [DOI:10.3389/fnsys.2010.00032] [PMID] [PMCID]
Ma, D., Chen, C. B., Jhanji, V., Xu, C., Yuan, X. L., & Liang, J. J., et al. (2020). Expression of SARS-CoV-2 receptor ACE2 and TMPRSS2 in human primary conjunctival and pterygium cell lines and in mouse cornea. Eye, 34(7), 1212–1219. [DOI:10.1038/s41433-020-0939-4] [PMID] [PMCID]
Mantini, D., Perrucci, M. G., Del Gratta, C., Romani, G. L., & Corbetta, M. (2007). Electrophysiological signatures of resting state networks in the human brain. Proceedings of the National Academy of Sciences of the United States of America, 104(32), 13170–13175. [DOI:10.1073/pnas.0700668104] [PMID] [PMCID]
Mao, L., Wang, M., Chen, S., He, Q., Chang, J., & Hong, C., et al. (2020). Neurological manifestations of hospitalized patients with COVID-19 in Wuhan, China: A retrospective case series study. Medrxiv, [Unpublished]. [DOI:10.1101/2020.02.22.20026500]
Marsland, A. L., Kuan, D. C. H., Sheu, L. K., Krajina, K., Kraynak, T. E., Manuck, S. B., & Gianaros, P. J. (2017). Systemic inflammation and resting state connectivity of the default mode network. Brain, Behavior, and Immunity, 62, 162-170. [DOI: 10.1016/j.bbi.2017.01.013] [PMID]
Matías-Guiu, J., Gomez-Pinedo, U., Montero-Escribano, P., Gomez-Iglesias, P., Porta-Etessam, J., & Matias-Guiu, J. A. (2020). Should we expect neurological symptoms in the SARS-CoV-2 epidemic? Neurología, 35(3), 170-175. [DOI:10.1016/j.nrleng.2020.03.002]
Miller, E. K., & Buschman, T. J. (2013). Cortical circuits for the control of attention. Current Opinion in Neurobiology, 23(2), 216–222. [DOI:10.1016/j.conb.2012.11.011] [PMID] [PMCID]
Nichols, T. E., & Holmes, A. P. (2002). Nonparametric permutation tests for functional neuroimaging: A primer with examples. Human Brain Mapping, 15(1), 1–25. [DOI:10.1002/hbm.1058] [PMID] [PMCID]
Nile, S. H., Nile, A., Qiu, J., Li, L., Jia, X., & Kai, G. (2020). COVID-19: Pathogenesis, cytokine storm and therapeutic potential of interferons. Cytokine & Growth Factor Reviews, 53, 66–70. [DOI:10.1016/j.cytogfr.2020.05.002] [PMID] [PMCID]
World Health Organization (WHO). (2020). Novel Coronavirus (2019-nCoV): Situation report-3. Geneva: World Health Organization. [Link]
Rabany, L., Diefenbach, G. J., Bragdon, L. B., Pittman, B. P., Zertuche, L., & Tolin, D. F., et al. (2017). Resting-state functional connectivity in generalized anxiety disorder and social anxiety disorder: Evidence for a dimensional approach. Brain Connectivity, 7(5), 289–298. [DOI:10.1089/brain.2017.0497] [PMID]
Scalf, P. E., Ahn, J., Beck, D. M., & Lleras, A. (2014). Trial history effects in the ventral attentional network. Journal of Cognitive Neuroscience, 26(12), 2789–2797. [DOI:10.1162/jocn_a_00678] [PMID]
Singhal T. (2020). A review of coronavirus disease-2019 (COVID-19). Indian Journal of Pediatrics, 87(4), 281–286. [DOI:10.1007/s12098-020-03263-6] [PMID] [PMCID]
Smith, S. M., Fox, P. T., Miller, K. L., Glahn, D. C., Fox, P. M., Mackay, C. E., et al. (2009). Correspondence of the brain's functional architecture during activation and rest. Proceedings of the National Academy of Sciences of the United States of America, 106(31), 13040–13045. [DOI:10.1073/pnas.0905267106] [PMID] [PMCID]
Tucker, P, & Czapla, CS. (2020). Post-COVID stress disorder: Another emerging consequence of the global pandemic. Psychiatric Times, 38(1), 9-11. [Link]
Vicentini, J. E., Weiler, M., Almeida, S. R. M., de Campos, B. M., Valler, L., & Li, L. M. (2017). Depression and anxiety symptoms are associated to disruption of default mode network in subacute ischemic stroke. Brain Imaging and Behavior, 11(6), 1571–1580. [DOI:10.1007/s11682-016-9605-7] [PMID]
Wang, X. L., Iwanami, J., Min, L. J., Tsukuda, K., Nakaoka, H., & Bai, H. Y., et al. (2016). Deficiency of angiotensin-converting enzyme 2 causes deterioration of cognitive function. NPJ Aging and Mechanisms of Disease, 2, 16024. [DOI:10.1038/npjamd.2016.24] [PMID] [PMCID]
Wu, Y., Xu, X., Chen, Z., Duan, J., Hashimoto, K., & Yang, L., et al. (2020). Nervous system involvement after infection with COVID-19 and other coronaviruses. Brain, Behavior, and Immunity, 87, 18–22. [DOI:10.1016/j.bbi.2020.03.031] [PMID] [PMCID]
Yao, N., Cheung, C., Pang, S., Shek-kwan Chang, R., Lau, K. K., & Suckling, J., et al. (2016). Multimodal MRI of the hippocampus in Parkinson's disease with visual hallucinations. Brain Structure & Function, 221(1), 287–300. [DOI:10.1007/s00429-014-0907-5] [PMID] [PMCID]
Zhang, H., Penninger, J. M., Li, Y., Zhong, N., & Slutsky, A. S. (2020). Angiotensin-converting enzyme 2 (ACE2) as a SARS-CoV-2 receptor: Molecular mechanisms and potential therapeutic target. Intensive Care Medicine, 46(4), 586–590. [DOI:10.1007/s00134-020-05985-9] [PMID] [PMCID]
Zhou, F., Yu, T., Du, R., Fan, G., Liu, Y., & Liu, Z., et al. (2020). Clinical course and risk factors for mortality of adult inpatients with COVID-19 in Wuhan, China: A retrospective cohort study. Lancet, 395(10229), 1054–1062. [DOI:10.1016/S0140-6736(20)30566-3] [PMID] [PMCID]
Zhu, H., Zhou, P., Alcauter, S., Chen, Y., Cao, H., & Tian, M., et al. (2016). Changes of intranetwork and internetwork functional connectivity in Alzheimer's disease and mild cognitive impairment. Journal of Neural Engineering, 13(4), 046008. [DOI:10.1088/1741-2560/13/4/046008] [PMID]
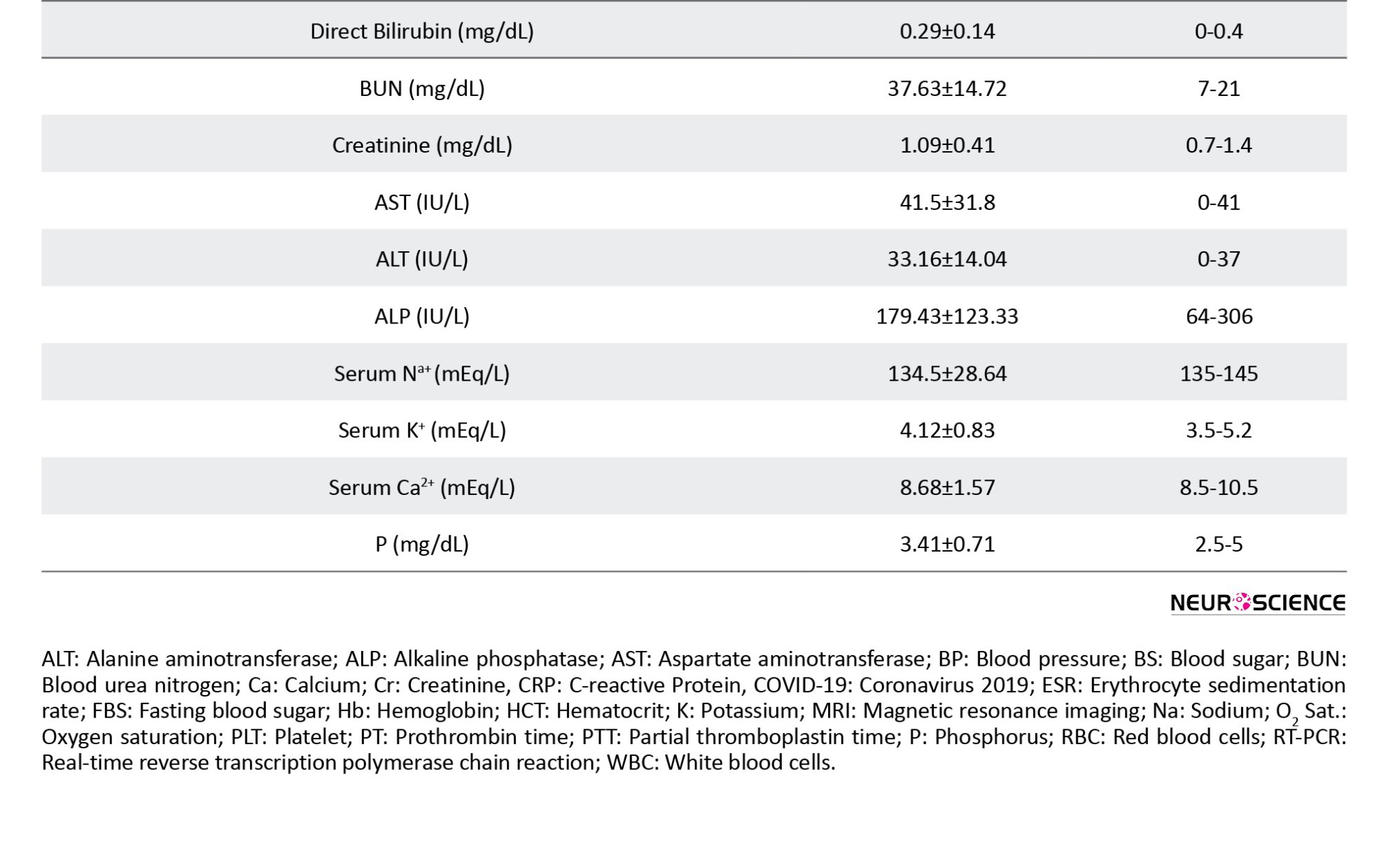