1. Introduction
Attention-deficit/hyperactivity disorder (ADHD) is one of the most prevalent chronic childhood mental illnesses, affecting 4%-12% of all school-aged children and persisting in roughly 66%-85% of cases (Getahun et al., 2013). The disease affects individuals across the lifespan (Sonuga-Barke et al., 2013). It is characterized by age-inappropriate, chronic, and pervasive symptoms of inattention, hyperactivity, and or impulsivity. These symptoms are associated with a high risk of scholastic failure, interpersonal difficulties, and mental illnesses (Sonuga-Barke et al., 2013).
Electroencephalography (EEG) measurements show the relationship between intracranial electrical currents and the scalp’s resultant voltages, representing specific aspects of brain electrical activity and processing. Many pieces of research have been conducted to compare brain activity in children with ADHD to normal controls, particularly utilizing EEG, to shed more light on the underlying neurophysiology of ADHD and to study subtypes of ADHD with varied responses to treatment (Arns et al., 2013). The most commonly reported EEG characteristics associated with ADHD are increased slow wave power (delta, theta) and or decreased fast wave power (beta), which are occasionally combined and indexed as theta-to-beta ratio (TBR): The ratio of theta band (4-7 Hz) power divided by beta band (13-30 Hz) power (Arns et al., 2013; Lenartowicz & Loo, 2014; Barry et al., 2003). Previous studies that employed TBR to distinguish an ADHD group from a control group found a sensitivity of 87% and a specificity of 94% (Snyder & Hall, 2006).
A significant increase in power in the theta band might be used to establish an ADHD diagnosis. In ADHD, elevated theta might be regarded as a sign of inattention and executive dysfunction (Ogrim et al., 2012). However, the diagnostic value of the TBR biomarker increases when it can make a differentiation between ADHD and other disorders (especially other neurodevelopmental dysfunctions). Nevertheless, very little research has been done on the discriminative power of the TBR index for psychological disorders. Cooldige et al. (2007) investigated the differential power of TBR among children with different psychological problems and found its lack of power both in terms of sensitivity and specificity to diagnose children with ADHD.
This issue is especially critical in children with learning disabilities (LD). Previous research has indicated high comorbidity for ADHD and LD in children. That is, attention deficit hyperactivity disorder has the highest comorbidity rate with learning disorder, co-occurs 33% to 45% of the time with reading disability and 11% of the time with mathematical disability (Mayes et al., 2000; DuPaul et al., 2013; Butterworth & Kova, 2013). The rate is so high that it has led Hendrickson et al. (2007) to consider attention impairments as one of the subgroups of learning disorders, along with verbal and non-verbal types.
On the other hand, a wide range of EEG studies have shown greater theta and less beta power in frontal areas in children with LD compared to the control group (Jäncke et al., 2019). In their research on LD children, Jäncke and Alahamadi (2016) found that theta/beta and theta/alpha ratios were much higher in LD children than in healthy children, indicating a significant slowdown of EEG oscillations, particularly for frontal scalp positions (involved in the control of executive functions, attention, planning, and language). Moreover, in a review of the studies that considered or disregarded ADHD comorbidity, Chabot et al. (2001) indicated that in addition to alpha and theta deviations, an elevated ratio of theta to beta has been shown in children with learning disabilities. Chabot concluded that due to the overlap of behavioral symptoms and common neural infrastructure, attention deficit might be considered one of the LD subgroups (Chabot et al., 2001).
In the present study, the power of the TBR biomarker to differentiate between ADHD and LD was investigated during the cognitive task condition.
2. Materials and Methods
Study subjects
In this descriptive causal-comparative study, we examined 2 different groups of children: 15 children who met the criteria for specific learning disorder (SLD) according to DSM-5 (4 girls and 11 boys, Mean±SD age: 8.8±1.14 y) and 15 children who met the criteria for ADHD according to DSM-5 (3 girls and 12 boys, Mean±SD age: 8.9±SD y). The sample was collected from students with complaints of difficulty in academic achievements who were referred to the Atieh Clinical Neuroscience Center (Tehran City, Iran) from July to November 2020.
A clinical psychologist, an educational psychologist, and a psychiatrist checked the criteria with parents during psychological interviews and observed the child in separate sessions. Subjects who received a joint diagnosis from all three specialists were included in the study. The subjects were matched in two groups based on age. Normal intelligence ability was tested earlier in children with SLD diagnosis by the revised version of the Tehran-Stanford-Binet intelligence scale (Mahvashe et al., 2014). The exclusion criteria were a history of neurological and or psychiatric problems, severe sociocultural disadvantages, abnormal psychomotor development, and visual and auditory deficits. Excluded children with anxiety, mood, or other psychiatric disorders received the diagnosis through the interview process. Furthermore, all three presentations of ADHD were included in the study.
Visual continuous performance task (VCPT)
This study used a VCPT. This task was a cued go/no-go task and primarily assessed the executive function of suppressing an action. The 400 trials were divided into four categories, each consisting of a pair of sequentially presented visual stimuli. In go trials, a picture of an animal is followed by a picture of an animal, and the participant is asked to press a button as fast as possible. In no-go trials, the participant is asked to refrain from clicking the button since a picture of an animal is followed by a picture of a plant. No action is needed in ignore trials. In this condition, a picture of a plant is followed by a picture of a plant or a human (in the novelty condition, a picture of a plant is followed by a picture of a human being, the latter being presented along with a novel sound) (Mueller et al., 2010). VCPT was presented via open-source Python software and synchronized with EEG Studio software to record electroencephalograph data.
Electroencephalographic Recording
Electroencephalograms were recorded using the 19-channel Mitsar-EEG system (Mitsar, Russia) in an active (VCPT task) state. We used 19 silver-chloride electrodes fixed to the scalp according to the international 10-20 system using Electro-Cap (ElectroCap, Inc, OH) with its electrodes placed on Fp1/Fp2, F3/F4, C3/C4, P3/P4, O1/O2, F7/F8, T3/T4, T5, T6, Fz, Cz, and Pz. The EEG input signals were referenced to linked ears, filtered between 0.5 to 40 Hz, and digitized at a rate of 500 Hz. The ground electrode was placed on the forehead. All electrode impedances were kept below 5 kΩ. EEG was recorded for at least 22 minutes during the VCPT task. The participants were sat in a comfortable chair in a dimly lit, acoustically isolated room. The participants were instructed to sit still without blinking or moving their eyes. WinEEG software was used to correct and preprocess artifacts. Artifact-contaminated epochs (eye blinks, rapid eye movements, slow head or body movements, and myographic artifacts) were automatically marked and excluded from further analysis. The exclusion thresholds were set as follows: (a) 100 μV for nonfiltered EEG, (b) 50 μV for slow waves in the 0–1 Hz band, and (c) 20 μV for fast waves filtered in the 20–35 Hz band. For EEG data analysis, not less than 30 artifact-free EEG epochs were used (around 60 s).
Data analysis
WinEEG software, was used for artifact correction and preprocessing. Artifact-contaminated epochs (eye blinks, rapid eye movements, slow head or body movements, and myographic artifacts) were automatically marked and excluded from further analysis. The exclusion thresholds were set as follows: (a) 100 μV for nonfiltered EEG, (b) 50 μV for slow waves in the 0–1 Hz band, and (c) 20 μV for fast waves filtered in the 20–35 Hz band. For EEG data analysis, not less than 30 artifact-free EEG epochs were used (around 60 s).
After applying the quick Fourier transform, the absolute power was computed for the theta (4–8 Hz) and beta1 (13–20 Hz) frequency bands in Fz and Cz. The TBR was computed in these regions, and the t-test was used to compare the groups’ mean. It should be mentioned that to get close to the normal distribution, we applied an independent t-test after calculating the logarithms for the amplitude numbers.
3. Results
Table 1 presents the demographic characteristics of the participants.
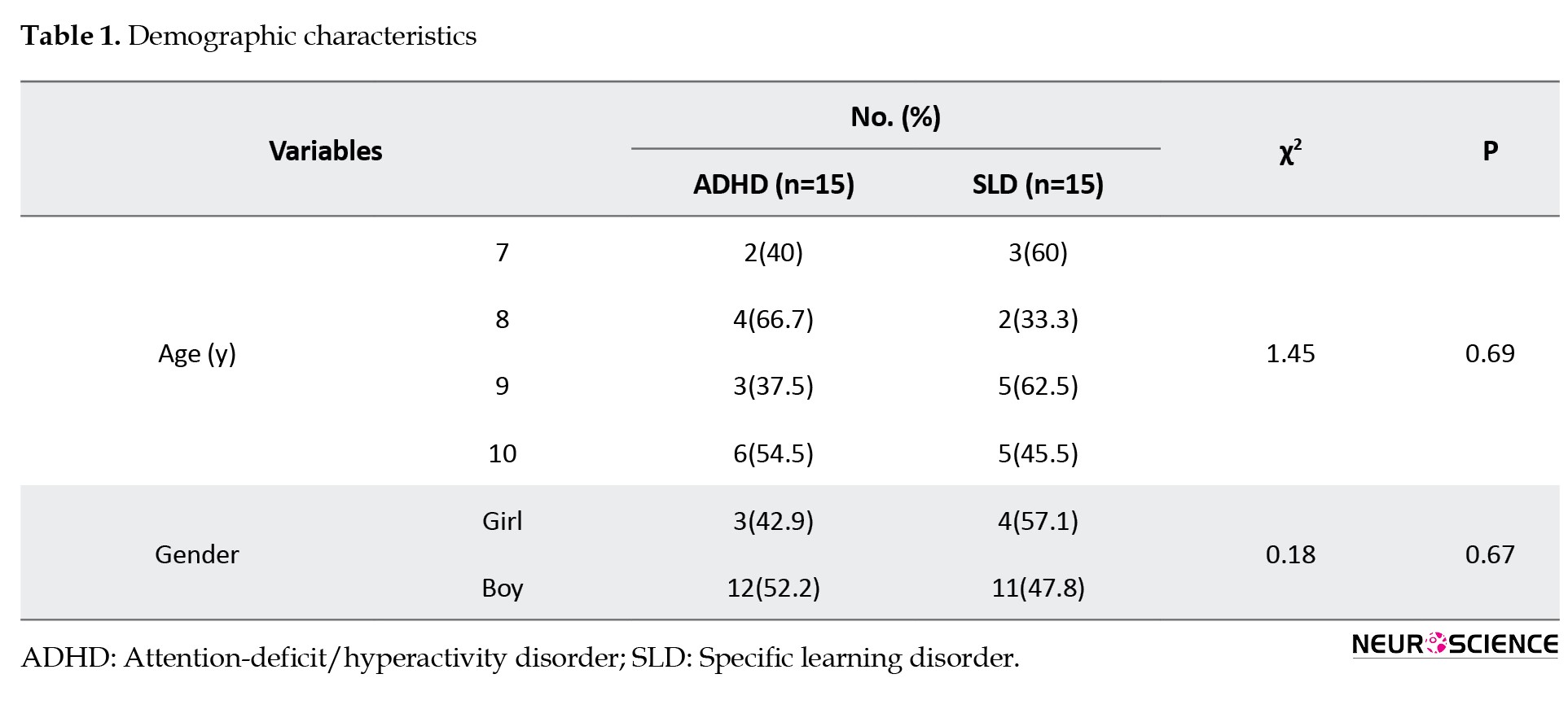
The participants’ ages ranged from 7 to 10 years. The chi-square test was performed to compare age and gender groupings. The chi-square test revealed no significant differences (P<0.05) between the groups regarding age and gender variables, as shown in
Table 1. Therefore, it can be argued that both groups of SLD and ADHD were matched in age and gender characteristics.
We compared the group’s performance in the VCPT task based on omission (t=0.021), commission (t=0.292), reaction time (t=-1.281), and variance in responses (t=1.091).
Table 2 shows no significant difference between the two groups regarding behavioral variables (P<0.05).
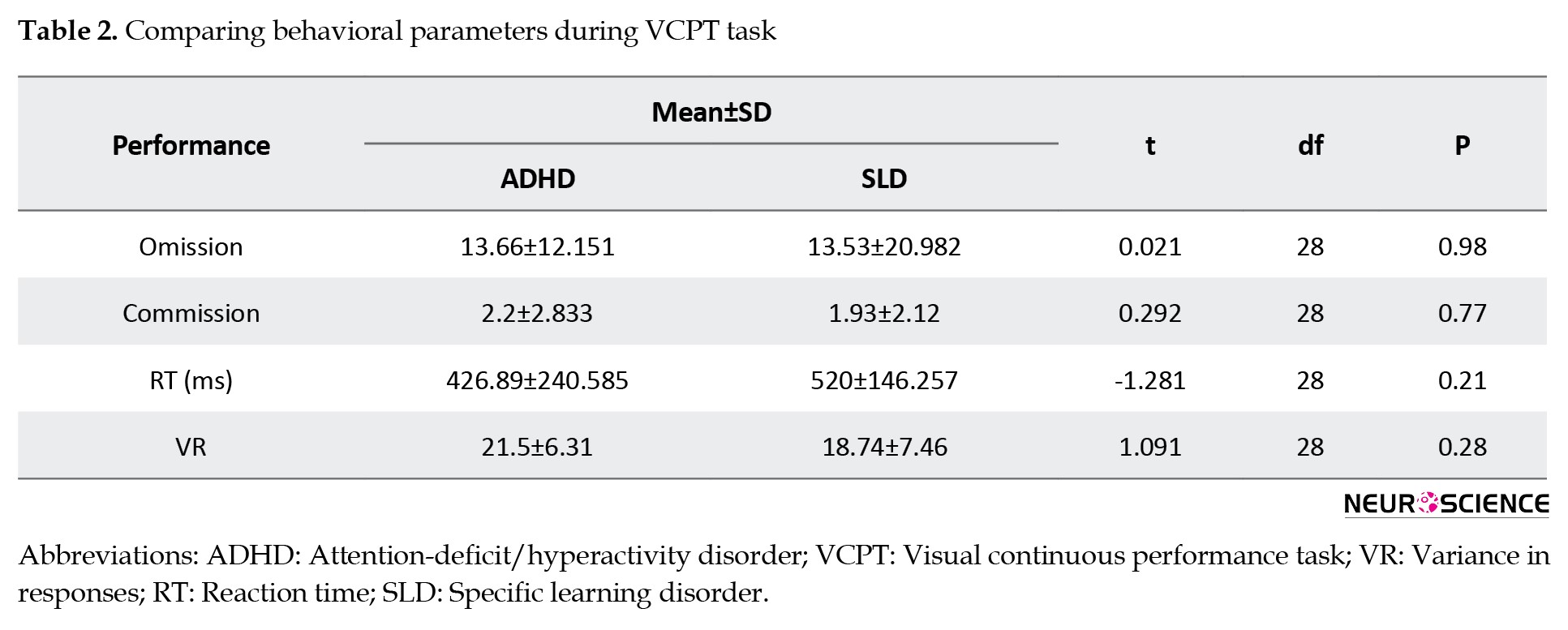
Based on
Table 3, the results of the independent t-test indicated no significant difference between the groups regarding TBR in Fz (t=-0.492, P=0.62) and Cz (t=0.406, P value=0.68) during the VCPT condition (P<0.05).
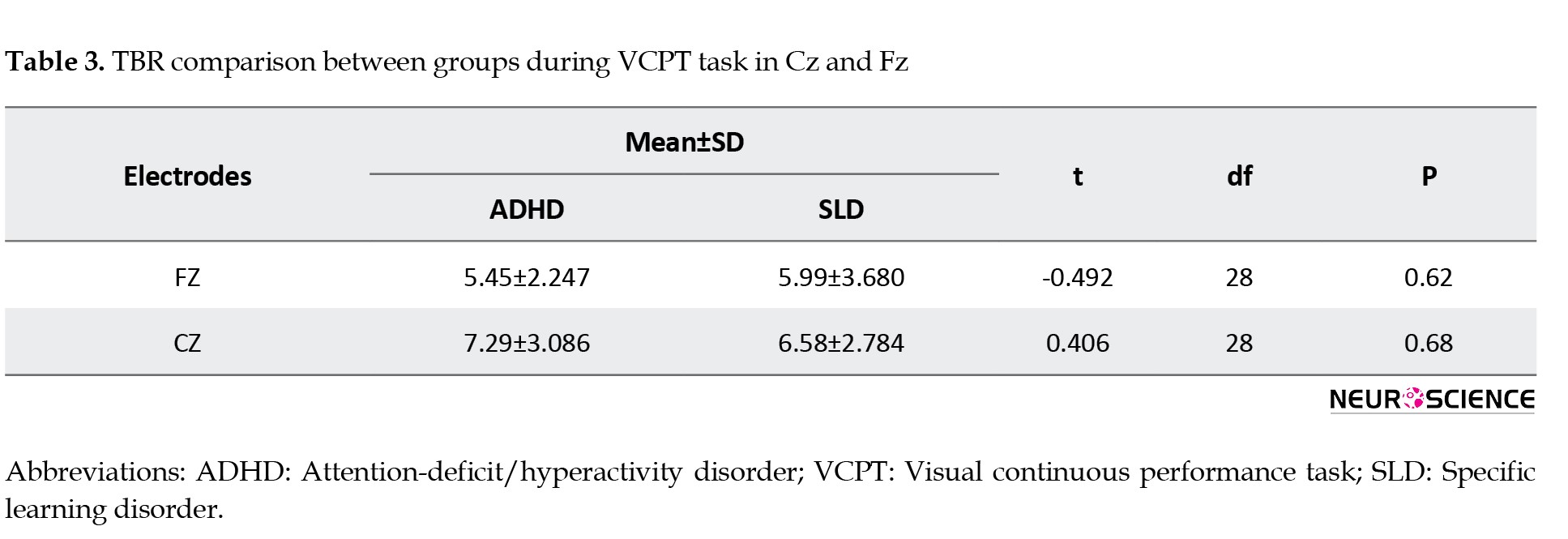
Therefore, no significant difference was found between SLD and ADHD children in terms of TBR in Cz and Fz sites during the VCPT task.
4. Discussion
In this study, TBR during a visual CPT was investigated in children with ADHD compared to children with SLD. EEG data were recorded on frontal and central sites. In addition, differences in the performance of these groups in VCPT were also calculated. Our analysis revealed significant distinctions in TBR and cognitive measures of CPT.
The ADHD group, compared to SLD, presented no difference in central and frontal TBR during attention tasks. There is no literature comparing the TBR of these groups while doing a cognitive performance task. However, the controversial issues of TBR differentiation power for people with LD and especially ADHD are still ongoing. Many studies have shown increased TBR or elevated slow waves in individuals with ADHD (e.g. Markovska-Simoska & Pop-Jordanova, 2017; Fonseca et al., 2008; Ogrim et al., 2012; Boutros et al., 2005; Chabot et al. 2001; Monastra et al., 2001) and learning disabilities (e.g. Jäncke et al., 2017; Jäncke & Alhamadi, 2016; Fernandez et al., 2003; Chabot et al. 2001). For example, Markovska-Simoska et al. (2017) reported 81% accuracy in the differentiation of unmedicated ADHD individuals from healthy controls using TBR power. Although TBR abnormalities have typically been found in ADHD populations, several studies failed to replicate the differences (e.g. Saad et al., 2015; Poil et al., 2014; Arns & Gordon, 2014; Arns et al., 2013; Barry et al., 2009; Murias et al., 2007).
Even if the TBR power to differentiate between ADHD and HC is assumed reliable, it might not be an effective biomarker unless it also differentiates ADHD from other mental conditions (especially neurodevelopmental disorders). In a leading investigation by Coolidge et al., QEEG was used as a diagnostic tool in children with different emotional and behavioral problems. Using TBR to diagnose ADHD among children with a vast range of psychological issues, Coolidge et al. (2007) found a sensitivity of 50% and a specificity of 36%, differentiating ADHD (as estimated by parents) from other psychological disorders. In the current study, differentiation and sensitivity/specificity measures were not calculated, but the results are consistent with the aforementioned study, showing that TBR inefficiency should be counted for differentiating ADHD from other mental health issues.
In most studies on this matter, the conventional, most regular approach of QEEG research (i.e. eyes-open or eyes-closed resting condition) (Klimesch, 1999) has been used. In this approach, we can examine the brain’s baseline (tonic) features in a resting state. By comparison, only scarce literature exists on QEEG under active conditions, mainly focused on brain dynamics while doing a task using an even-related (phasic) paradigm. P300 amplitude and latency in attention tasks (such as oddball, CPT, and go/no-go) are reported as a standard component to study aspects of processing in ADHD literature (e.g. Barry et al., 2003; Fallagater et al., 2004; Clarke et al., 2019) and to compare information processing and inhibition in individuals with ADHD and LD (e.g. Lubar et al., 1990; Buchman et al., 2011). Jonkman et al. (2000) came with reduced P300 amplitude to target stimuli in children with ADHD, while in new stimuli, P300 was normal. They claimed that it shows a deficit in attention allocation but not poorer cerebral processing capacity. On the other hand, tonic arousal, as presented by frontal TBR, is shown to be increased rather than phasic arousal (i.e. P300 component) in various attention tasks in healthy subjects (Howell et al., 2010). Our current research investigated TBR in ADHD and LD as a tonic arousal index in frontocentral areas during a task that requires both inhibition and attention allocation. Accordingly, though there was no difference in TBR in children with ADHD and LD during the task, there might still be distinctive TBR in the ADHD group in the default mode resting state. Several studies show that latency and amplitude in P300 might be a more effective biomarker index for investigating hypothetical differences between these disorders while doing cognitive tasks.
On the other hand, main executive functions have long been associated with the frontal cortex (Fiske & Holmboe, 2019). Many studies have acknowledged mid-frontal TBR as a marker for cognitive functions rather than the brain arousal index. According to the findings, theta oscillation might be related both to inhibitory control (Angelidis et al., 2018; Cavanagh & Frank, 2014; Putamen et al., 2014), attention (Guo et al., 2020; Onton et al., 2005), and processing speed (Zhang et al., 2017).
In a series of studies, (Barry et al., 2004, Barry et al., 2005, Barry et al., 2009) found no link between TBR and skin conductance level as a widely acceptable measure of arousal. Clarke et al. (2019) investigated the association between TBR and amplitude and latency of the P300 ERP component during an attentional task, showing TBR as linked with cognitive capacity in the normal group. Accumulating evidence on individuals with ADHD (Gou et al., 2020; Picken et al., 2020; Halawa et al., 2017; Markovska-Simoska & Pop-Jordanova, 2017; van Dongen-Boomsma et al., 2010) also confirms that frontal TBR is related to executive, most notably attentional, control. Given the nearly consistent results in the role of TBR in cognitive control, an elevated TBR index during cognitive performance tasks in groups with cognitive impairments is reasonably expected. Training cognitive abilities might decrease the index during rehabilitation, as Sari et al. (2015) have found about anxious people with executive dysfunctions.
In our findings, in line with QEEG results, no differences in attention, inhibitory control, and processing speed were found between the groups with LD and ADHD. Complying with our findings, many studies are showing executive dysfunctions in individuals with ADHD and SLD. As Barkley (1997) suggested, ADHD is claimed to emerge from a primary deficit in executive functions with a specific core of dysfunctional response inhibition, which has been investigated in several diagnostic and therapeutic studies (e.g. Baumeister et al., 2018; Azami et al., 2016; Buchmann, 2011; Martinussen et al., 2005; Cornoldi et al., 2001; Mariani & Barkley, 1997). Other investigations have shown the importance of inhibition and attention impairments associated with mathematics and reading underachievement (Cartwright et al., 2012; Merrell & Tymms, 2001). Executive functions in people with pure ADHD, pure LD, and combined LD+ADHD have been compared in several research, mostly seen similar difficulties in inhibitory control (Abou El Wafa et al., 2020; Ghamarigivi et al., 2009; Passolunghi et al., 2005) and regulation of attention resources (Martinussen & Tannock, 2006, Wu et al., 2002), while more severe impairment was observed in children with comorbidity (Purvis & Tannock, 2000; Huang et al., 2016).
5. Conclusion
Considering the findings, observing similar cerebral activations in frontocentral areas in these groups during a cognitive task may confirm the role of theta/beta oscillations in attention and inhibition.
Study Limitations
This study has a number of restrictions. The medication usage in subjects was not controlled, and we have no data that would determine if there was a difference between unmedicated vs medicated children. Resting state EEG and cognitive tasks in active conditions may demonstrate greater accuracy in identifying ADHD and LD. Also, including a larger sample size will result in more convincing results, especially with age considerations to contemplate maturational delay. These are challenges for future research.
Ethical Considerations
Compliance with ethical guidelines
All procedures were in accordance with the ethical standards of the responsible committee on human experimentation and with the Helsinki Declaration revised in 2008. Informed consent was obtained from legally authorized representatives (parents) of all subjects to be included in the study.
Funding
This research did not receive any grant from funding agencies in the public, commercial, or non-profit sectors.
Authors' contributions
Data collection and data analysis: Fatemeh Gholamali Nezhad: Writing the original draft: Fatemeh Gholamali Nezhad and Mahdieh Sadat Mirmohammad and Hanieh Ahmadi; Review and editing: Mahdieh Sadat Mirmohammad: Supervision: Reza Rostami.
Conflict of interest
All authors declared no conflict of interest.
Acknowledgments
The authors thank Martijn Arns for contributing to the study design. EEG data analysis was also done under his supervision.
References
Abou El Wafa, H. E., Ghobashy, S. A. E. L., & Hamza, A. M. (2020). A comparative study of executive functions among children with attention deficit and hyperactivity disorder and those with learning disabilities. Middle East Current Psychiatry, 27(1), 1-9. [DOI:10.1186/s43045-020-00071-8]
Angelidis, A., Hagenaars, M., van Son, D., van der Does, W., & Putman, P. (2018). Do not look away! Spontaneous frontal EEG theta/beta ratio as a marker for cognitive control over attention to mild and high threat. Biological Psychology, 135, 8-17. [DOI:10.1016/j.biopsycho.2018.03.002] [PMID]
Arns, M., & Gordon, E. (2014). Quantitative EEG (QEEG) in psychiatry: diagnostic or prognostic use? Clinical Neurophysiology, 125(8), 1504–1506. [DOI:10.1016/j.clinph.2014.01.014] [PMID]
Arns, M., Conners, C. K., & Kraemer, H. C. (2013). A decade of EEG theta/beta ratio research in ADHD: A meta-analysis. Journal of Attention Disorders, 17(5), 374–383.[DOI:10.1177/1087054712460087] [PMID]
Azami, S., Moghadas, A., Sohrabi-Esmrood, F., Nazifi, M., Mirmohamad, M., & Hemmati, F., et al. (2016). A pilot randomized controlled trial comparing computer-assisted cognitive rehabilitation, stimulant medication, and an active control in the treatment of ADHD. Child and Adolescent Mental Health, 21(4), 217–224. [DOI:10.1111/camh.12157] [PMID]
Barkley R. A. (1997). Behavioral inhibition, sustained attention, and executive functions: Constructing a unifying theory of ADHD. Psychological Bulletin, 121(1), 65–94. [DOI:10.1037/0033-2909.121.1.65] [PMID]
Barry, R. J., Clarke, A. R., & Johnstone, S. J. (2003). A review of electrophysiology in attention-deficit/hyperactivity disorder: I. Qualitative and quantitative electroencephalography. Clinical Neurophysiology, 114(2), 171–183. [DOI:10.1016/S1388-2457(02)00362-0] [PMID]
Barry, R. J., Clarke, A. R., Johnstone, S. J., McCarthy, R., & Selikowitz, M. (2009). Electroencephalogram theta/beta ratio and arousal in attention-deficit/hyperactivity disorder: Evidence of independent processes. Biological Psychiatry, 66(4), 398–401. [DOI:10.1016/j.biopsych.2009.04.027] [PMID]
Barry, R. J., Clarke, A. R., McCarthy, R., Selikowitz, M., Rushby, J. A., & Ploskova, E. (2004). EEG differences in children as a function of resting-state arousal level. Clinical Neurophysiology, 115(2), 402–408. [DOI:10.1016/S1388-2457(03)00343-2] [PMID]
Barry, R. J., Rushby, J. A., Wallace, M. J., Clarke, A. R., Johnstone, S. J., & Zlojutro, I. (2005). Caffeine effects on resting-state arousal. Clinical Neurophysiology, 116(11), 2693–2700. [DOI:10.1016/j.clinph.2005.08.008] [PMID]
Baumeister, S., Wolf, I., Holz, N., Boecker-Schlier, R., Adamo, N., & Holtmann, M., et al. (2018). Neurofeedback training effects on inhibitory brain activation in ADHD: A matter of learning? Neuroscience, 378, 89–99. [DOI:10.1016/j.neuroscience.2016.09.025] [PMID]
Boutros, N., Fraenkel, L., & Feingold, A. (2005). A four-step approach for developing diagnostic tests in psychiatry: EEG in ADHD as a test case. The Journal of Neuropsychiatry and Clinical Neurosciences, 17(4), 455–464. [DOI:10.1176/jnp.17.4.455] [PMID]
Buchmann, J., Gierow, W., Reis, O., & Haessler, F. (2011). Intelligence moderates impulsivity and attention in ADHD children: An ERP study using a go/nogo paradigm. The World Journal of Biological Psychiatry, 12(Suppl 1), 35–39. [DOI:10.3109/15622975.2011.600354] [PMID]
Butterworth, B., & Kovas, Y. (2013). Understanding neurocognitive developmental disorders can improve education for all. Science, 340(6130), 300–305. [DOI:10.1126/science.1231022] [PMID]
Cartwright, K. B. (2012). Insights from cognitive neuroscience: The importance of executive function for early reading development and education. Early Education & Development, 23(1), 24-36. [DOI:10.1080/10409289.2011.615025]
Cavanagh, J. F., & Frank, M. J. (2014). Frontal theta as a mechanism for cognitive control. Trends in Cognitive Sciences, 18(8), 414–421. [DOI:10.1016/j.tics.2014.04.012] [PMID]
Chabot, R. J., di Michele, F., Prichep, L., & John, E. R. (2001). The clinical role of computerized EEG in the evaluation and treatment of learning and attention disorders in children and adolescents. The Journal of Neuropsychiatry and Clinical Neurosciences, 13(2), 171–186. [DOI:10.1176/jnp.13.2.171] [PMID]
Clarke, A. R., Barry, R. J., Karamacoska, D., & Johnstone, S. J. (2019). The EEG theta/beta ratio: A marker of arousal or cognitive processing capacity? Applied Psychophysiology and Biofeedback, 44(2), 123–129. [DOI:10.1007/s10484-018-09428-6] [PMID]
Coolidge, F. L., Starkey, M. T., & Cahill, B. S. (2007). Comparison of a parent-rated DSM-IV measure of attention-deficit/hyperactivity disorder and quantitative EEG parameters in an outpatient sample of children. Journal of Clinical Neurophysiology, 24(4), 348–351. [DOI:10.1097/WNP.0b013e318067bcfc] [PMID]
Cornoldi, C., Marzocchi, G. M., Belotti, M., Caroli, M. G., Meo, T., & Braga, C. (2001). Working memory interference control deficit in children referred by teachers for ADHD symptoms. Child Neuropsychology, 7(4), 230–240. [DOI:10.1076/chin.7.4.230.8735] [PMID]
DuPaul, G. J., Gormley, M. J., & Laracy, S. D. (2013). Comorbidity of LD and ADHD: Implications of DSM-5 for assessment and treatment. Journal of Learning Disabilities, 46(1), 43–51.[DOI:10.1177/0022219412464351] [PMID]
Fernández, T., Herrera, W., Harmony, T., Díaz-Comas, L., Santiago, E., & Sánchez, L., et al. (2003). EEG and behavioral changes following neurofeedback treatment in learning disabled children. Clinical EEG, 34(3), 145–152. [DOI:10.1177/155005940303400308] [PMID]
Fiske, A., & Holmboe, K. (2019). Neural substrates of early executive function development. Developmental Review, 52, 42–62. [DOI:10.1016/j.dr.2019.100866] [PMID]
Fonseca, L. C., Tedrus, G. M., Moraes, C.d, Vicente Machado, A.d, Almeida, M. P., & Oliveira, D. O. (2008). Epileptiform abnormalities and quantitative EEG in children with attention-deficit/hyperactivity disorder. Arquivos de Neuro-Psiquiatria, 66(3A), 462–467. [DOI:10.1590/S0004-282X2008000400004] [PMID]
Getahun, D., Jacobsen, S. J., Fassett, M. J., Chen, W., Demissie, K., & Rhoads, G. G. (2013). Recent trends in childhood attention-deficit/hyperactivity disorder. JAMA Pediatrics, 167(3), 282–288. [DOI:10.1001/2013.jamapediatrics.401] [PMID]
Ghamarigivi, H. (2009). [Comparison of executive functions among children with attention deficit hyperactivity disorder, learning disability and normal children (Persian)]. Journal of Fundamentals of Mental Health, 11(44), 33-322. [DOI:10.22038/jfmh.2009.1216]
Guo, J., Luo, X., Li, B., Chang, Q., Sun, L., & Song, Y. (2020). Abnormal modulation of theta oscillations in children with attention-deficit/hyperactivity disorder. NeuroImage. Clinical, 27, 102314. [DOI:10.1016/j.nicl.2020.102314] [PMID]
Halawa, I. F., El Sayed, B. B., Amin, O. R., Meguid, N. A., & Abdel Kader, A. A. (2017). Frontal theta/beta ratio changes during TOVA in Egyptian ADHD children. Neurosciences, 22(4), 287–291.[DOI:10.17712/nsj.2017.4.20170067] [PMID]
Hendriksen, J. G., Keulers, E. H., Feron, F. J., Wassenberg, R., Jolles, J., & Vles, J. S. (2007). Subtypes of learning disabilities: neuropsychological and behavioural functioning of 495 children referred for multidisciplinary assessment. European child & Adolescent Psychiatry, 16(8), 517–524. [DOI:10.1007/s00787-007-0630-3] [PMID]
Howells, F. M., Stein, D. J., & Russell, V. A. (2010). Perceived mental effort correlates with changes in tonic arousal during attentional tasks. Behavioral and Brain Functions, 6, 39. [DOI:10.1186/1744-9081-6-39] [PMID]
Huang, F., Sun, L., Qian, Y., Liu, L., Ma, Q. G., & Yang, L., et al. (2016). Cognitive function of children and adolescents with attention deficit hyperactivity disorder and learning difficulties: A developmental perspective. Chinese Medical Journal, 129(16), 1922–1928. [DOI:10.4103/0366-6999.187861] [PMID]
Jäncke, L., & Alahmadi, N. (2016). Resting state EEG in children with learning disabilities: An independent component analysis approach. Clinical EEG and Neuroscience, 47(1), 24–36. [DOI:10.1177/1550059415612622] [PMID]
Jäncke, L., Saka, M. Y., Badawood, O., & Alhamadi, N. (2019). Resting-state electroencephalogram in learning-disabled children: Power and connectivity analyses. Neuroreport, 30(2), 95–101. [DOI:10.1097/WNR.0000000000001166] [PMID]
Jonkman, L. M., Kemner, C., Verbaten, M. N., Van Engeland, H., Camfferman, G., & Buitelaar, J. K., et al. (2000). Attentional capacity, a probe ERP study: Differences between children with attention-deficit hyperactivity disorder and normal control children and effects of methylphenidate. Psychophysiology, 37(3), 334–346. [DOI:10.1111/1469-8986.3730334] [PMID]
Klimesch, W. (1999). EEG alpha and theta oscillations reflect cognitive and memory performance: A review and analysis. Brain Research Reviews, 29(2-3), 169-195. [DOI:10.1016/S0165-0173(98)00056-3]
Lenartowicz, A., & Loo, S. K. (2014). Use of EEG to diagnose ADHD. Current Psychiatry Reports, 16(11), 498. [DOI:10.1007/s11920-014-0498-0] [PMID]
Lubar, J. F., Gross, D. M., Shively, M. S., & Man, C. A. (1990). Differences between normal, learning disabled and gifted children based upon on auditory evoked potential task. Journal of Psychophysiology, 4(3), 249–260. [Link]
Mahvashe, W. A., Javidnia, S., & Sadeghi, H. (2014). Assessment of the psychometric properties of the new version of Tehran-stanford-binet intelligence scale in children with dyslexia. Zahedan Journal of Research in Medical Sciences, 16(9), 26-29. [Link]
Mariani, M. A. & Barkley, R. R. (1997). Neuropsychological and academic function in preschool boys with attention deficit hyperactivity disorder. Developmental Neuropsychology, 13(1), 111-129. [DOI:10.1080/87565649709540671]
Markovska-Simoska, S., & Pop-Jordanova, N. (2017). Quantitative EEG in children and adults with attention deficit hyperactivity disorder: Comparison of absolute and relative power spectra and theta/beta ratio. Clinical EEG and Neuroscience, 48(1), 20–32. [DOI:10.1177/1550059416643824] [PMID]
Martinussen, R., & Tannock, R. (2006). Working memory impairments in children with attention-deficit hyperactivity disorder with and without comorbid language learning disorders. Journal of Clinical and Experimental Neuropsychology, 28(7), 1073–1094. [DOI:10.1080/13803390500205700] [PMID]
Martinussen, R., Hayden, J., Hogg-Johnson, S., & Tannock, R. (2005). A meta-analysis of working memory impairments in children with attention-deficit/hyperactivity disorder. Journal of the American Academy of Child and Adolescent Psychiatry, 44(4), 377–384. [DOI:10.1097/01.chi.0000153228.72591.73] [PMID]
Mayes, S. D., Calhoun, S. L., & Crowell, E. W. (2000). Learning disabilities and ADHD: Overlapping spectrumn disorders. Journal of Learning Disabilities, 33(5), 417–424. [DOI:10.1177/002221940003300502] [PMID]
Merrell, C., & Tymms, P. B. (2001). Inattention, hyperactivity and impulsiveness: Their impact on academic achievement and progress. The British Journal of Educational Psychology, 71(Pt 1), 43–56. [DOI:10.1348/000709901158389] [PMID]
Monastra, V. J., Lubar, J. F., & Linden, M. (2001). The development of a quantitative electroencephalographic scanning process for attention deficit-hyperactivity disorder: Reliability and validity studies. Neuropsychology, 15(1), 136–144. [DOI:10.1037/0894-4105.15.1.136] [PMID]
Mueller, A., Candrian, G., Kropotov, J. D., Ponomarev, V. A., & Baschera, G. M. (2010). Classification of ADHD patients on the basis of independent ERP components using a machine learning system. Nonlinear Biomedical Physics, 4(Suppl 1), S1. [DOI:10.1186/1753-4631-4-S1-S1] [PMID]
Murias, M., Swanson, J. M., & Srinivasan, R. (2007). Functional connectivity of frontal cortex in healthy and ADHD children reflected in EEG coherence. Cerebral Cortex, 17(8), 1788–1799. [DOI:10.1093/cercor/bhl089] [PMID]
Ogrim, G., Kropotov, J., & Hestad, K. (2012). The quantitative EEG theta/beta ratio in attention deficit/hyperactivity disorder and normal controls: Sensitivity, specificity, and behavioral correlates. Psychiatry Research, 198(3), 482–488. [DOI:10.1016/j.psychres.2011.12.041] [PMID]
Onton, J., Delorme, A., & Makeig, S. (2005). Frontal midline EEG dynamics during working memory. NeuroImage, 27(2), 341–356. [DOI:10.1016/j.neuroimage.2005.04.014] [PMID]
Passolunghi, M. C., Marzocchi, G. M., & Fiorillo, F. (2005). Selective effect of inhibition of literal or numerical irrelevant information in children with attention deficit hyperactivity disorder (ADHD) or arithmetic learning disorder (ALD). Developmental Neuropsychology, 28(3), 731–753. [DOI:10.1207/s15326942dn2803_1] [PMID]
Picken, C., Clarke, A. R., Barry, R. J., McCarthy, R., & Selikowitz, M. (2020). The theta/beta ratio as an index of cognitive processing in adults with the combined type of attention deficit hyperactivity disorder. Clinical EEG and Neuroscience, 51(3), 167–173. [DOI:10.1177/1550059419895142] [PMID]
Poil, S. S., Bollmann, S., Ghisleni, C., O'Gorman, R. L., Klaver, P., & Ball, J., et al. (2014). Age dependent electroencephalographic changes in attention-deficit/hyperactivity disorder (ADHD). Clinical Neurophysiology, 125(8), 1626–1638. [DOI:10.1016/j.clinph.2013.12.118] [PMID]
Purvis, K. L., & Tannock, R. (2000). Phonological processing, not inhibitory control, differentiates ADHD and reading disability. Journal of the American Academy of Child and Adolescent Psychiatry, 39(4), 485–494. [DOI:10.1097/00004583-200004000-00018] [PMID]
Putman, P., Verkuil, B., Arias-Garcia, E., Pantazi, I., & van Schie, C. (2014). EEG theta/beta ratio as a potential biomarker for attentional control and resilience against deleterious effects of stress on attention. Cognitive, Affective & Behavioral Neuroscience, 14(2), 782–791. [DOI:10.3758/s13415-013-0238-7] [PMID]
Sari, B. A., Koster, E. H., Pourtois, G., & Derakshan, N. (2016). Training working memory to improve attentional control in anxiety: A proof-of-principle study using behavioral and electrophysiological measures. Biological Psychology, 121(Pt B), 203–212. [DOI:10.1016/j.biopsycho.2015.09.008] [PMID]
Saad, J. F., Kohn, M. R., Clarke, S., Lagopoulos, J., & Hermens, D. F. (2018). Is the theta/beta EEG marker for ADHD inherently flawed? Journal of Attention Disorders, 22(9), 815–826. [DOI:10.1177/1087054715578270] [PMID]
Snyder, S. M., & Hall, J. R. (2006). A meta-analysis of quantitative EEG power associated with attention-deficit hyperactivity disorder. Journal of Clinical Neurophysiology, 23(5), 441–456. [Link]
Sonuga-Barke, E. J., Brandeis, D., Cortese, S., Daley, D., Ferrin, M., Holtmann, M., et al. (2013). Nonpharmacological interventions for ADHD: Systematic review and meta-analyses of randomized controlled trials of dietary and psychological treatments. The American Journal of Psychiatry, 170(3), 275–289. [DOI:10.1176/appi.ajp.2012.12070991] [PMID]
van Dongen-Boomsma, M., Lansbergen, M. M., Bekker, E. M., Kooij, J. J., & van der Molen, M., et al. (2010). Relation between resting EEG to cognitive performance and clinical symptoms in adults with attention-deficit/hyperactivity disorder. Neuroscience Letters, 469(1), 102–106. [DOI:10.1016/j.neulet.2009.11.053] [PMID]
Wu, K. K., Anderson, V., & Castiello, U. (2002). Neuropsychological evaluation of deficits in executive functioning for ADHD children with or without learning disabilities. Developmental Neuropsychology, 22(2), 501–531. [DOI:10.1207/S15326942DN2202_5] [PMID]
Zhang, D. W., Roodenrys, S., Li, H., Barry, R. J., Clarke, A. R., & Wu, Z., et al. (2017). Atypical interference control in children with AD/HD with elevated theta/beta ratio. Biological Psychology, 128, 82–88. [DOI:10.1016/j.biopsycho.2017.07.009] [PMID]