1. Introduction
Cognitive skills play a vital role in learning capacity and task performance. Attention, memory, perception, and logical reasoning represent various levels of cognitive functions. Studies on human cognition have revealed several biological, behavioral, and environmental factors contributing to the augmentation or deterioration of cognitive skills. One such factor is the indiscriminate use of smartphones, which adversely affects cognitive capacities, impacting physical and mental health (Shaffer, 1996). In recent years, smartphones have become embedded in almost every domain of human life, such as social networking, education, business, entertainment, etc. However, excessive use of smartphones can sometimes lead to addiction, which has emerged as a prevalent social problem that disrupts daily life. Smartphone addiction indicates the inability to control smartphone use despite adverse effects on users (Shaffer, 1996). Recent studies have shown that the prevalence of smartphone addiction among children and adolescents is rapidly increasing (Soni et al., 2017). The younger population is more addicted to social media platforms and tends to use social networking sites during their study or work hours (Chiang et al., 2019). Heavy media multitasking or switching between tasks may result in cognitive decline and poor academic outcomes (Abi-Jaoudeet al., 2020; van der Schuur, 2015; Uncapher et al., 2017). Furthermore, it has been implicated in anxiety, impatience, withdrawal, mood changes, and lack of concentration in tasks, in addition to physical health problems such as pain in the wrist, neck, and joints, nervous disturbances, and fatigue (Hou et al., 2019; Thomée et al., 2011; Van Deursen, 2015). Hence, the current study investigates smartphone use or media multitasking effects on adolescents’ cognitive performance.
Researchers have used several techniques for estimating the cognitive performance of individuals, including behavioral, subjective, and neurophysiological measures (Tattersall et al., 1996; Hart et al., 1988; Borghini et al., 2016). Behavioral measures rely on the subjects’ performance in experimental tasks, while subjective measures rely on self-reports and questionnaires (Tattersall et al., 1996; Hart et al., 1988). Neurophysiological techniques measure cognitive performance using various physiological signals such as brain activity, cardiac activity, skin conductance, and the like (Borghini et al., 2016; Mühl et al., 2014; Gevins & Smith, 2003). Neurophysiological measures have been demonstrated as effective tools for real-time monitoring, thereby enhancing individuals’ cognitive performance.
Electroencephalography (EEG), a very convenient and low-cost technique that records the electrical activity of the brain, is being widely used to measure various cognitive assessment factors such as attention (Peng et al., 2020), mental workload (Gevins & Smith, 2003), working memory (Missonnier et al., 2006). EEG reflects the neuronal changes occurring due to cognitive engagement or fatigue; hence, EEG is widely used for assessing the cognitive performance of individuals. EEG is composed of several rhythms based on the frequency: Delta (1-3 Hz), theta (4-7 Hz), alpha (8-12 Hz), beta (13-30 Hz), and gamma (31-50 Hz) (Britton et al., 2016; Noachtar et al., 1999). Several neurocognitive experiments have shown that attention’s cognitive state is linked with theta, alpha, beta, and gamma bands of EEG (Freeman et al., 1999; Liu et al., 2013; Peng et al., 2020). Attention is a complex neural phenomenon comprising different brain regions (Rosenberg et al., 2016; Posner and Petersen, 1990) coupled with several frequencies (Clayton et al., 2015). Researchers have used various EEG features based on temporal, power spectral density (Liu et al., 2013), wavelet transform (Djamal et al., 2016), and Hilbert-Huang transform (Peng et al., 2020) for deriving neuro markers that detect attention from neural signals. Gruzelier (2009) showed that the ratio of the alpha band to theta band reflects the performance enhancement index of individuals during cognitive tasks. Freeman et al. (1999) demonstrated that the ratio of the beta band to theta band indicates neural activity and is a potential biomarker for attentional assessment. Rabbi et al. (2009) reported that the ratio of the beta to (alpha + theta) is indicative of cognitive performance and attentional resource index. Ming et al. (2009) used sample entropy of EEG samples to discriminate mental states of attention and inattention. This study reported a higher sample entropy during attentive tasks compared to the mental state of inattention.
Cognitive workload (CWL) is another term used to measure task complexity while performing a task. It indicates the human ability to maintain focus and rational reasoning while processing multiple activities and facing various distractions (Recarte & Nunes, 2003). Several recent studies have shown that CWL or task complexity is positively correlated with theta activity over the frontal region of the brain (Gevins & Smith, 2003) and inversely correlated with the alpha band over the parietal region (Borghini et al., 2014; Gevins et al., 1997).
With the advent of the internet and smartphone technologies, social networking sites have become very popular among people of different ages and professions. The investigation of the impact of problematic smartphone use on learning performance and work efficacy has gained more attention in recent years (Abi-Jaoudeet al., 2020; Darcin et al., 2015). Several studies have revealed the regions of brain activation during the use of social networking sites. Neuroimaging studies based on functional magnetic resonance imaging (fMRI) demonstrate that the task of social networking activates a network of brain regions such as the dorsomedial prefrontal cortex, bilateral temporoparietal junction, anterior temporal lobes, inferior frontal gyri, and posterior cingulate cortex/precuneus (Schurz et al., 2014; Saxe et al., 2003; Wolf et al., 2010).
Numerous studies have investigated the effects of phone use or media multitasking on cognitive performance in adolescents. However, most were based on self-report questionnaire surveys, while a few utilized changes in physiological signals like brain activity and heart rate. Moreover, neuroimaging studies using fMRI revealed the adverse effects of media multitasking on cognitive functioning (Moisala et al., 2016). Some EEG-based studies have examined the variations in EEG frequency bands induced by electromagnetic fields due to mobile phone radiation (Arns et al., 2007; Croft et al., 2008; Krause et al., 2006; Parmar et al., 2019). Of these studies, only a few investigated the impact of phone usage on cognitive tasks (Krause et al., 2006; Parmar et al., 2019). Moreover, such works used limited EEG features such as average amplitudes, frequencies, etc. The changes in brain activity patterns during cognitive tasks induced by media multitasking (or switching between learning and social media use) have not been investigated in detail. The main objective of the current study was to investigate the effects of smartphone distractions or media multitasking on adolescents’ cognitive performance using a large set of EEG indices. To achieve this, we examined the patterns of smartphone use among adolescents using a questionnaire survey. Following this, the impact of smartphone distractions on cognitive performance was assessed using a diverse set of EEG-based cognitive performance indices such as band ratios, attention index, CWL, and sample entropy. It is hypothesized that uncontrolled use of smartphones could be negatively associated with mental health and task performance.
2. Materials and Methods
Survey on smartphone use
A self-report questionnaire was used to survey the smartphone use pattern among adolescents in South India. The questionnaire included data on demography, phone use duration, phone use frequency during study/work, the most-used feature on the phone, and performance outcomes in academics or jobs. Data on stress and sleep patterns among phone users were also collected. The respondents were divided into the low-user group and the high-user group based on the duration of phone use. The participants who used their smartphones for more than 3 hours per day were grouped into the high-user and others into the low-user.
Data used
The current study used EEG data of healthy subjects recorded using EBNeuro Galileo BE Plus LTM 128 channel EEG acquisition system during various mental tasks such as resting and reading before and after smartphone usage. Twenty-two healthy subjects (14 men and 8 women) aged 18-32 years (Mean±SD: 21.73±2.78 y) participated in the EEG experiment. The subjects included undergraduate students and research staff from the institute. The subjects were ruled out of any medical or psychiatric conditions, and they were divided into two groups: the phone-use group (experimental group) and the control group. There were 11 subjects in each group with the Mean±SD ages 21.0±1.48 (phone-use group) and 22.45±3.58 (control group) with 4 women in each group.
EEG recording
This work recorded EEG signals using the BE Plus LTM 128-channel EEG acquisition system. All the EEG channels were recorded with an averaged reference, and electrode impedance was kept lower than 5 kΩ. The subjects were comfortably seated in an electrically shielded room. For the experimental group, the recording consisted of four sessions: the resting or relaxation phase, the reading task phase (named pre-use task phase), the smartphone use phase, and the reading task phase following the smartphone use (named post-use task phase). EEG was continuously recorded from each subject during the rest state (3 minutes duration) and the remaining three states (5 minutes duration). During resting, the participants were instructed to remain idle for three minutes without moving. During the reading stage, they had to read a scientific article related to fundamental brain functions (Farnsworth, 2018) for 5 minutes. During the phase of smartphone use, they were instructed to use the social media platform “Facebook” for 5 minutes, during which they viewed their profile photos and posts, including the comments they received. After 5 minutes of smartphone use, the subjects were asked to read the remaining part of the article. After 5 minutes of smartphone use, the subjects were asked to read the remaining part of the article. The experiment was conducted similarly for the control group but without smartphone use. Instead, the subjects were instructed to sit in an idle state between the two reading phases. The participants were asked to answer the questions related to the reading content and to express their mental state and whether they had felt any state of inattentive or fatigue during each task. Accordingly, the subject’s mental state was verified based on the subject’s feedback in answering questions and self-expression of the emotional state they felt during the task.
Figure 1 illustrates the sequence of operations performed to assess cognitive performance using EEG-based indices.
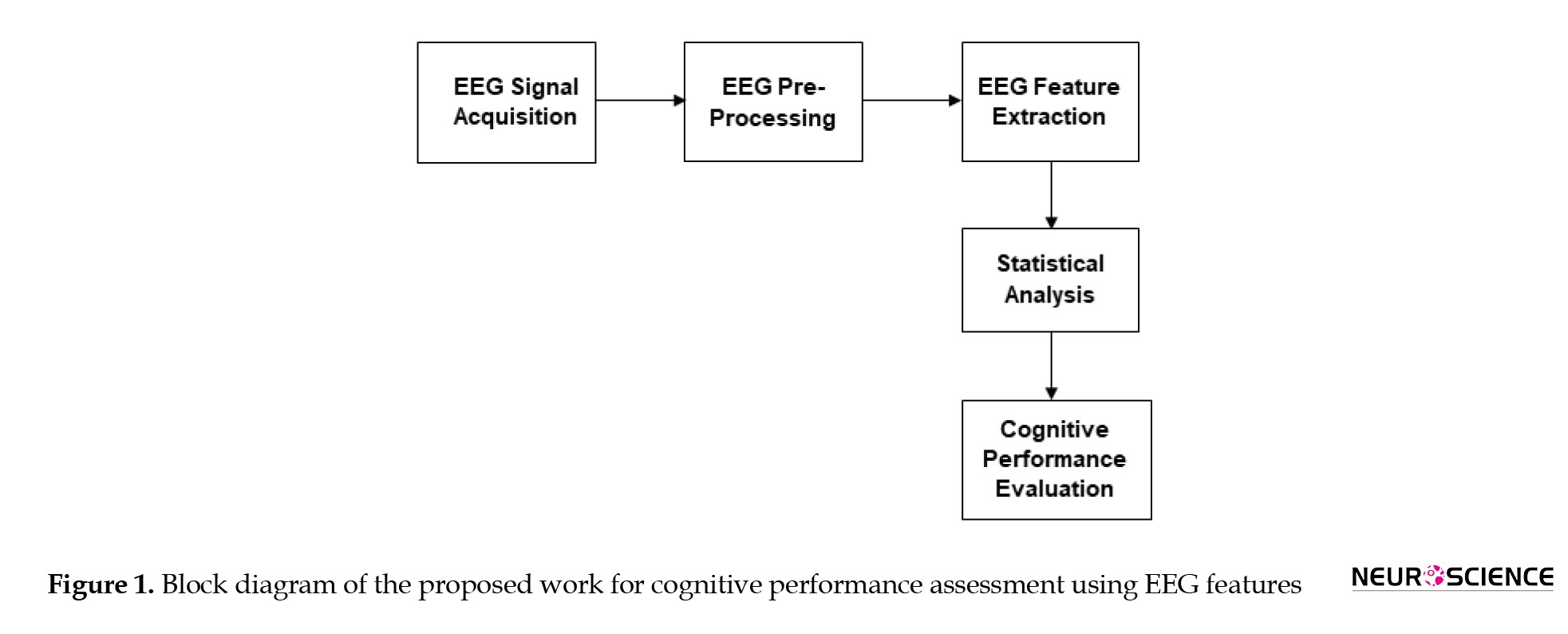
Sixty-four channels from different brain regions, including frontal (F), parietal (P), temporal (T), and occipital (O), were selected for EEG analysis. EEG data were online digitized with a sampling frequency of 128 Hz and exported to MATLAB-compatible format for further processing. Then, EEG was segmented into several epochs of 1-second duration, which had been reported as an optimal epoch duration to detect changes in neuronal activity during different mental states (Wang et al., 2014; Fraschini et al., 2016). The first 5 seconds in each trial were considered task preparation time for each subject, and those epochs were excluded from the analysis. EEG data were bandpass filtered between 1 Hz and 60 Hz, and an additional notch filtering was performed to eliminate 50 Hz power line noise interference. Amplitude thresholding was also performed to minimize movement artifacts, in which EEG samples with amplitudes greater than ±80 µV were excluded (Gotlib et al., 1998; Poppy and Speckens, 2015).
EEG feature extraction
EEG features were extracted from the pre-processed EEG in the frequency domain for interpreting brain activity. Wavelet transform was used to decompose EEG into various rhythms such as delta, theta, alpha, beta, and gamma. Wavelet transform is a very effective technique for the time-frequency analysis of non-stationary signals like EEG (Mallat, 1999; Polikar, 1999). It can detect any transient events occurring in the signal and decompose the given signal using a set of oscillating functions known as wavelets. Different families of wavelet functions ψa,b(t)' are formed as scaled and shifted versions of a unique mother wavelet' ψ(t)' according to
Equation 1.
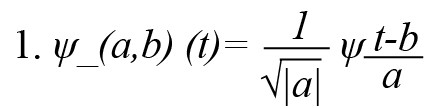
, where a, b ∈R,a≠0, 'a’ is the scaling parameter, ‘b’ is the shifting parameter, and ‘t’ is the time variable. Discrete wavelet transform (DWT) is a discrete version of the continuous wavelet transform, defined by assigning discrete values to wavelet parameters ‘a’ and ‘b’ (a=2-j and b=k 2-j, where j and k are integers representing the scale and translation). The current work performed a 4-level DWT decomposition to decompose the digitized EEG samples into different frequency components. The Daubechies-4 (db4) wavelet was chosen because it resembles the EEG waveform (Indiradevi et al., 2008). DWT decomposes the EEG signal to detail (high frequency) and approximation (low frequency) coefficients from which various EEG bands, ie, delta, theta, alpha, beta, and gamma, were selected (
Figure 2).
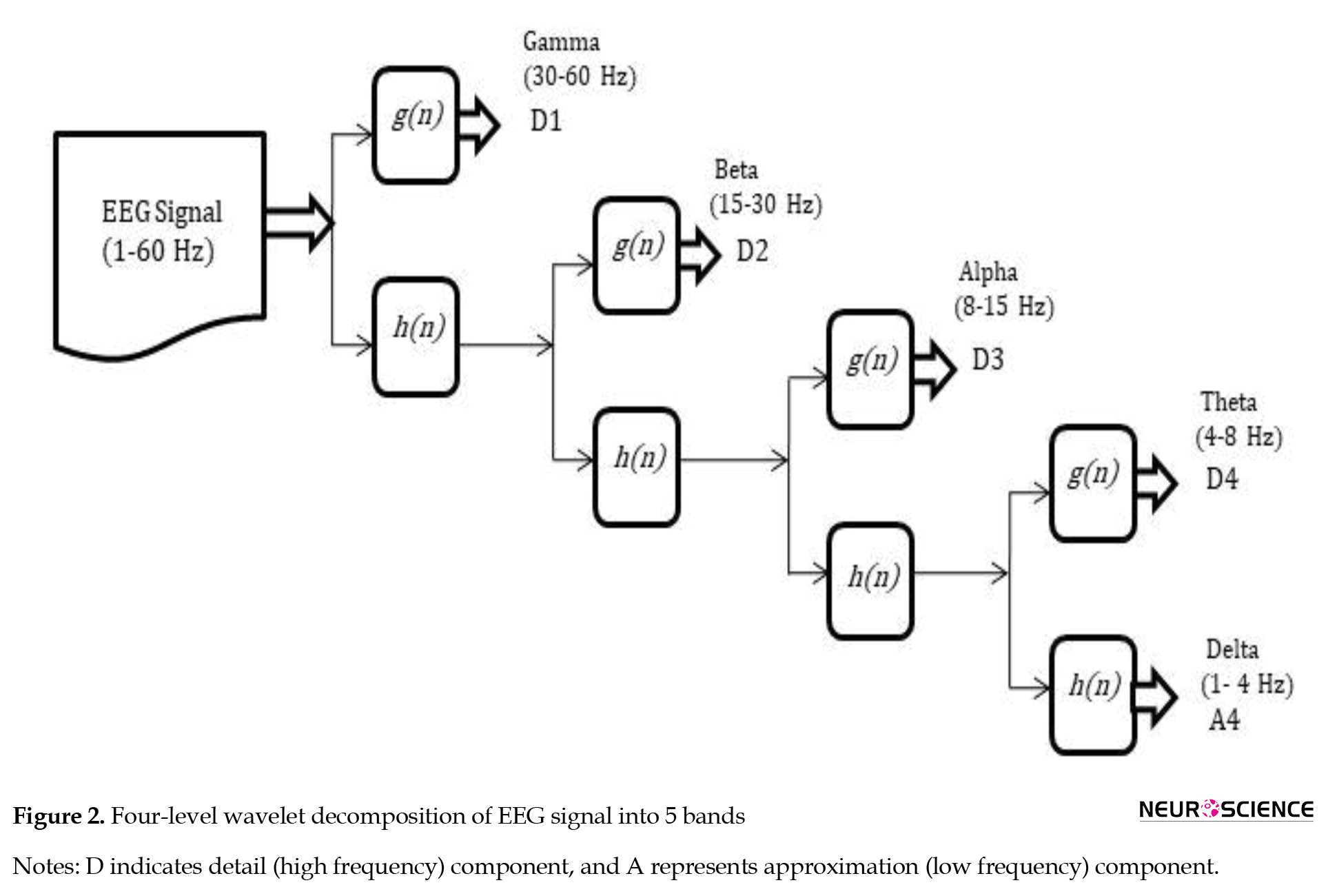
Based on the neuroscientific literature, various kinds of EEG features were extracted in this experiment. They included band ratios, attention index, and sample entropy of EEG samples. EEG band ratios have been reported as effective neuromarkers for recognizing the mental state of attentive tasks (Freeman et al., 1999; Gruzelier, 2009; Rabbi et al., 2009). The current experiment used different band ratios such as alpha-to-theta ratio (ATR), alpha-to-beta ratio (ABR), beta-to-theta ratio (BTR), and theta-to-gamma ratio (TGR). These were computed as the ratio of the absolute power of respective bands according to Equations
2-
5. Another ratio beta to (alpha + theta) ratio (BATR) was estimated as the ratio of theta absolute power to the sum of alpha absolute power and beta absolute power,
Equation 6.
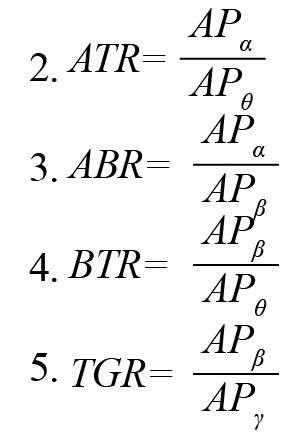
, where APθ, APα,APβ and APγ represent the absolute power of theta (θ), alpha (α), beta (β), and gamma (γ) bands, respectively.
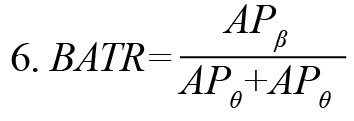
The attention index defined by a combination of band ratios (Suhail et al., 2021) was computed as
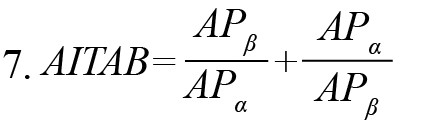
As EEG is a complex non-linear phenomenon, different entropies have been utilized to assess cognitive tasks’ mental states. Sample entropy is a powerful tool that measures the signal’s regularity. It was computed based on the algorithm proposed by Richman and Moorman (2000) with parameters m=2 and r=0.2×σ, where m is subseries length, r is similarity tolerance, and σ standard deviation of EEG samples.
CWL, a measure of task complexity, was estimated by the ratio of theta power across the frontal region to alpha power across the parietal region,
Equation 8 (Holm et al., 2009).

EEG features were normalized into a common scale [0 1] using the min-max normalization technique (Li et al., 2016). The normalized version of ith sample xi from a feature set X was computed using
Equation 9.
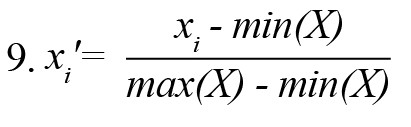
All the features were computed across two hemispheres (right and left) of different brain lobes (frontal, parietal, temporal, and occipital) by averaging feature values over the channels in respective lobes. For example, the feature BATR across the left frontal region (FL) was computed by averaging the values of BATR over F1, F3, F5, and F7 channels. In this way, all features were evaluated lobe-wise for each group.
Statistical analysis
The chi-square test was used to examine the impact of smartphone use (high-user and low-user groups) on task performance based on survey responses. The chi-square test is generally conducted to test statistical independence or association between two or more categorical variables. The Wilcoxon signed-rank test examined the significant differences in EEG patterns before and after smartphone use. This test is a non-parametric one used to compare related samples or matched pairs and is suitable for evaluating two different conditions of the same subjects (Scheff, 2016). The significance level was set to P<0.05 to examine the significant difference in performance indices between pre- and post-use tasks. The statistical analysis was performed using Matlab 2020a software.
3. Results
Questionnaire survey on smartphone use
The smartphone usage pattern among adolescents and its impact on task performance and health were examined using the survey. A total of 600 individuals, which included 341 men and 259 women, participated in this survey. Among the total participants of the survey, 310 (50.91%) belonged to the high-user group. Multiple parameters, including the most used feature in the phone, duration of phone use, phone use during studies/work, academic/work performance, sleep, and stress problems, were compared between high-user and low-user groups (
Table 1).
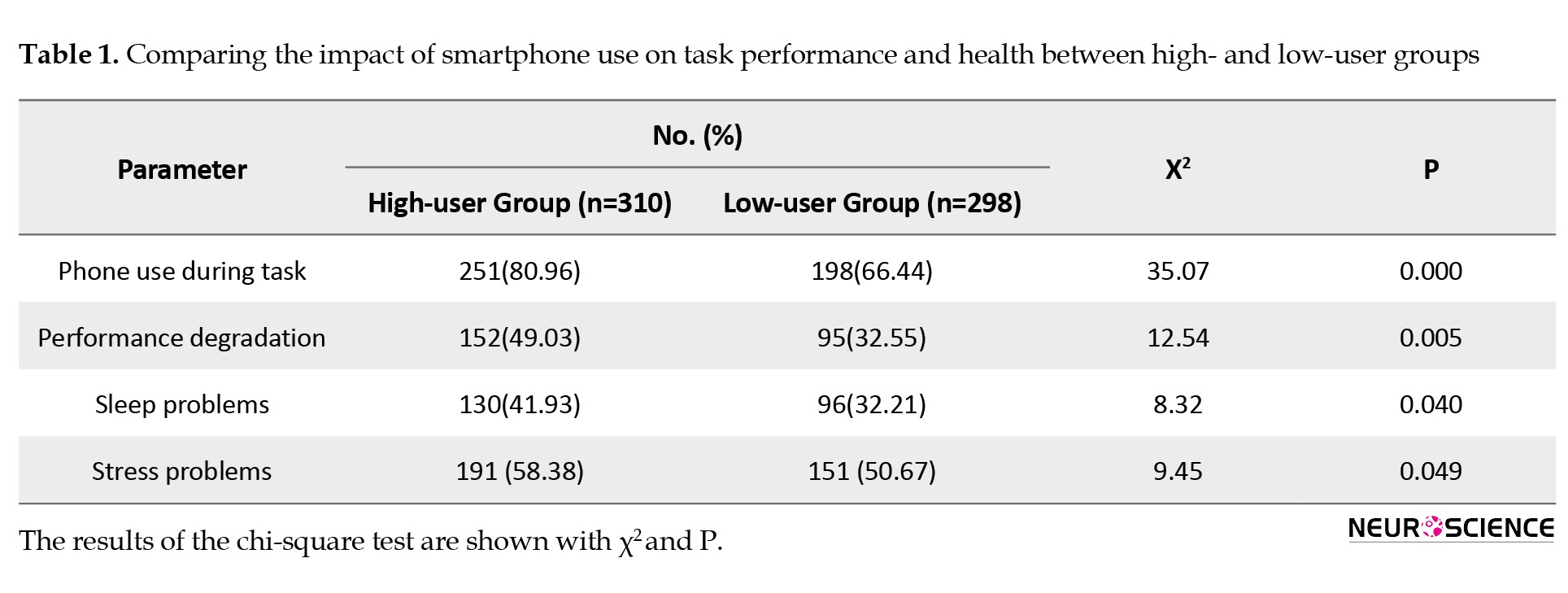
A significant difference (P=0.005) was observed in task performance between the high- and low-user groups. Performance degradation was more in the high-user group (49.03%) than in the low-user group (32.55%). Sleep problems (P=0.040) and mental stress (P=0.049) were also more prevalent in the high-user group.
EEG Experimental results
Following the survey, EEG rhythmic variations during the cognitive task before and after smartphone use were investigated in this experiment. Firstly, the changes in various EEG-based cognitive performance indices during rest and attentive states were examined for all subjects. Then, the variations in these EEG indices occurring due to phone use were evaluated by dividing the subjects into experimental (phone-use) and control groups. For the experimental group, EEG features were evaluated for the learning task before phone use (pre-use task) and after phone use (post-use task). For the control group, EEG features were evaluated for two phases of the learning task (phase-I and phase-II) with an idle state (without phone use) between the two phases. The variations in theta, alpha, beta, and gamma bands during the two learning tasks for each group are shown in
Figure 3.
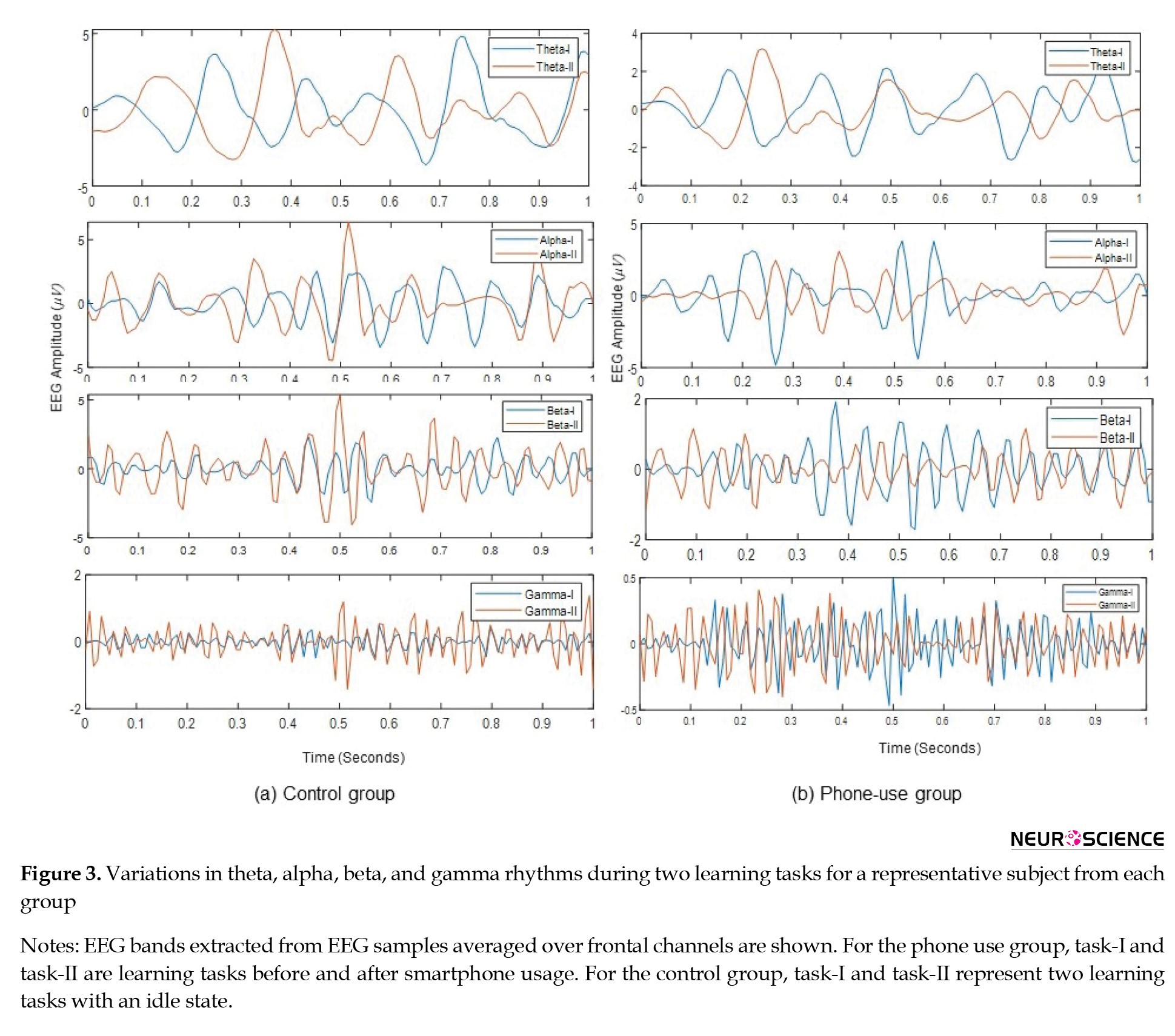
EEG bands extracted from EEG samples averaged over frontal channels are shown. For the phone use group, Task-I and Task-II are learning tasks before and after smartphone usage. For the control group, Task-I and Task-II represent two learning tasks with an idle state.
EEG variations during the state of attentiveness
The variations in different kinds of EEG features during rest and attentive states are shown in
Figure 4.
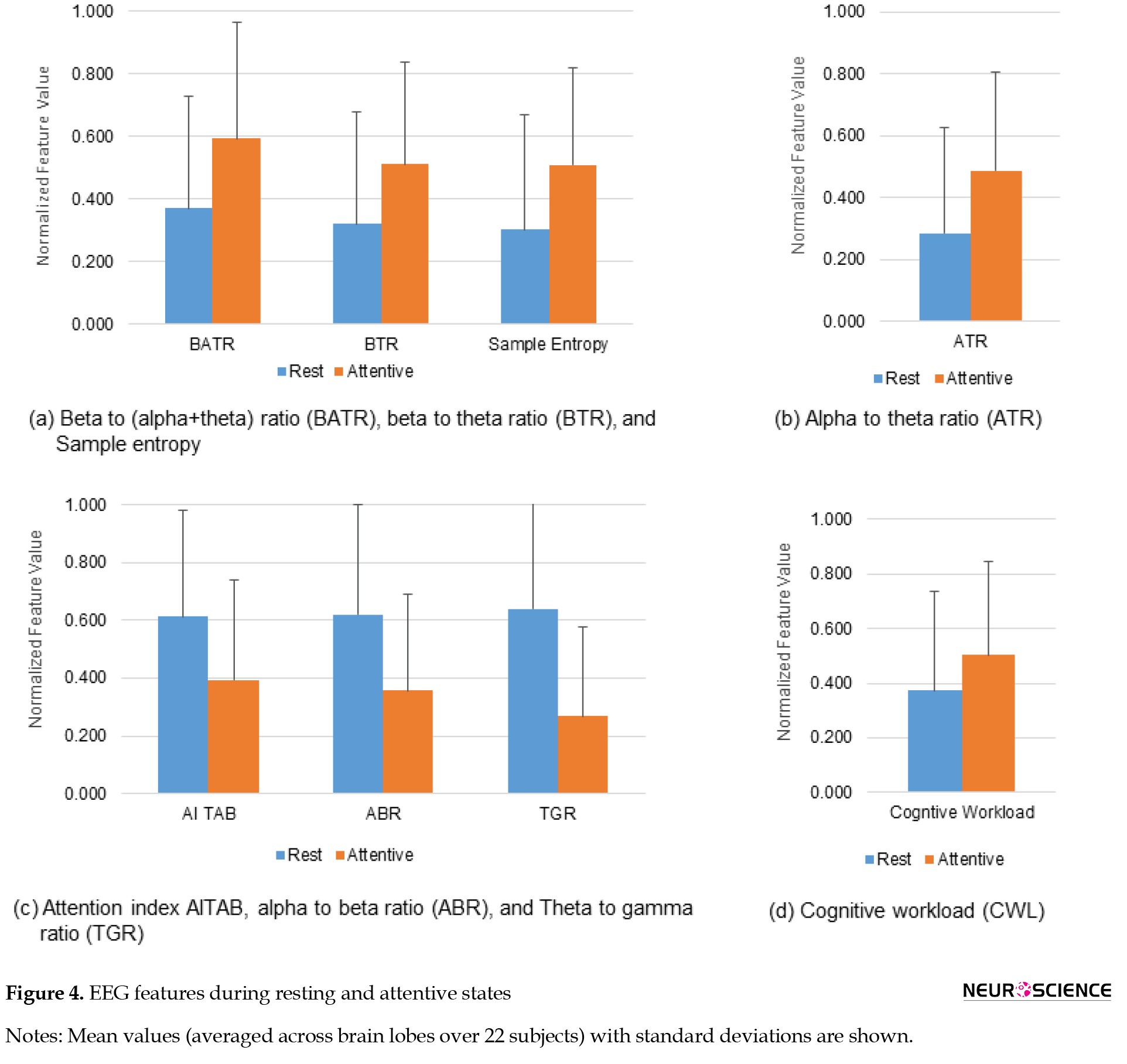
During the mental state of attention, EEG band ratios BATR (59.91%), ATR (71.35%), and BTR increased (59.97%), while ABR (42.39%) and TGR decreased (58.21%) across all lobes of the brain. A decrease in the attention index AITAB (35.79%) was also noticed during the attentive state. It observed an increase in sample entropy (68.56%) and CWL (35.08%) during the attentive state compared to the rest. The results signify that the increases of BATR, ATR, BTR, and sample entropy characterize the cognitive state of attention. The attentive state is also associated with ABR, TGR, and attention index decreases.
Effects of phone use on cognitive performance
The cognitive performances of the phone-use and control groups have been evaluated based on various EEG-based indices. The variations in EEG-based indices for the phone-use group and control group are shown in
Figure 5.
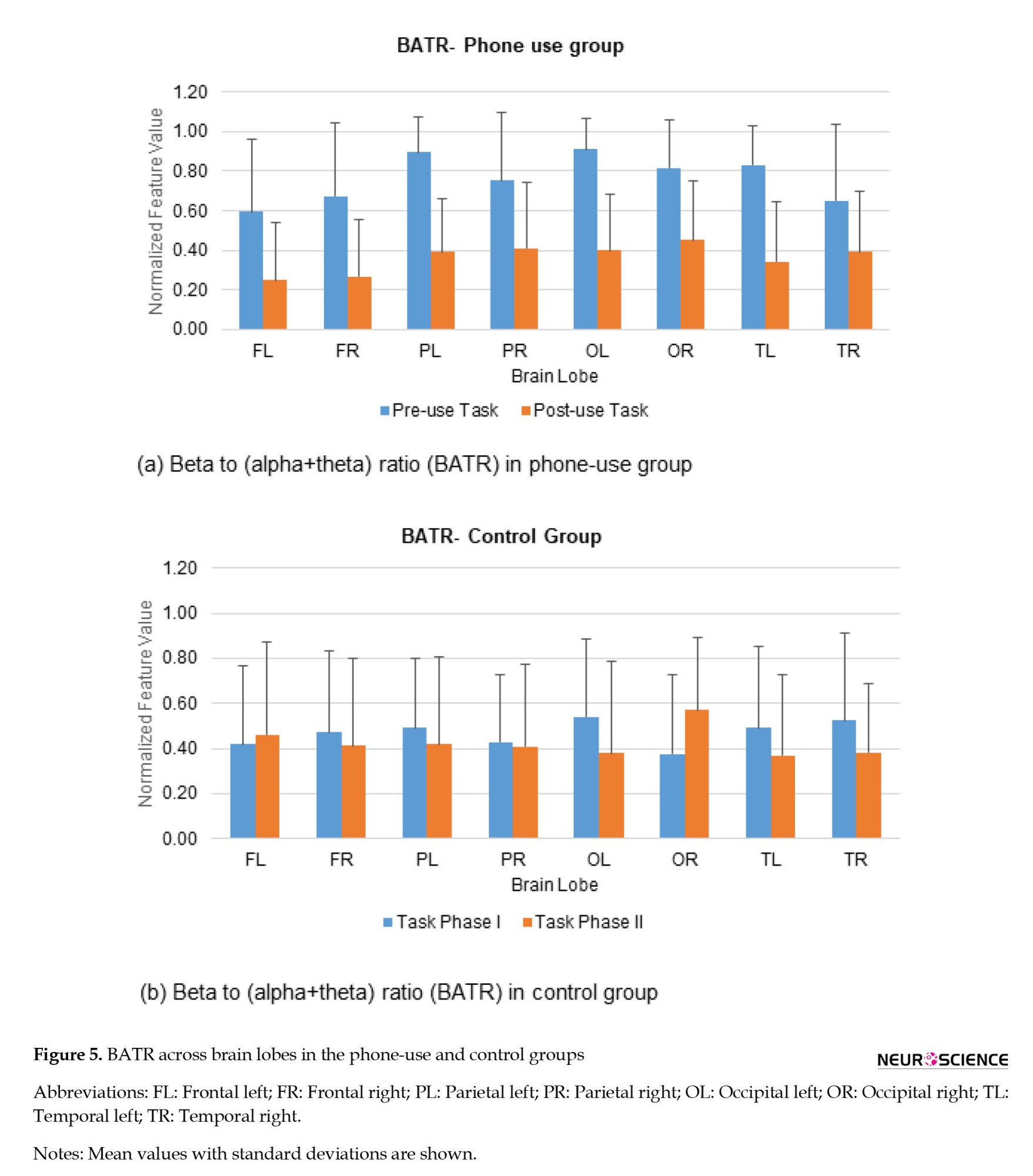
The ratio BATR decreased in the phone-use group. This ratio decreased in all brain lobes during the learning task after smartphone use. It has been demonstrated that BATR is associated with the attentional resource index, and a decrease in BATR represents a decline in cognitive performance (Rabbi et al., 2009). The mean values of BATR during the learning task before and after phone use for the experimental group are shown in
Figure 5. The decrease was larger in the right frontal (60.18%), left frontal (57.96%), and left occipital (56.02%) regions. The mean values of BATR during the learning task for the control group are shown in
Figure 5. For the control group, the ratio BATR increased during the learning task over the left frontal (10.67%) and right occipital (50.92%) regions while decreasing in all other lobes. As the frontal lobe is responsible for various cognitive functions like attention, memory, planning, problem-solving, etc. the changes in BATR across this region indicate variations in individuals’ cognitive task performance.
The variations in EEG-based cognitive performance indices for the phone-use and control groups are shown in
Figure 6.
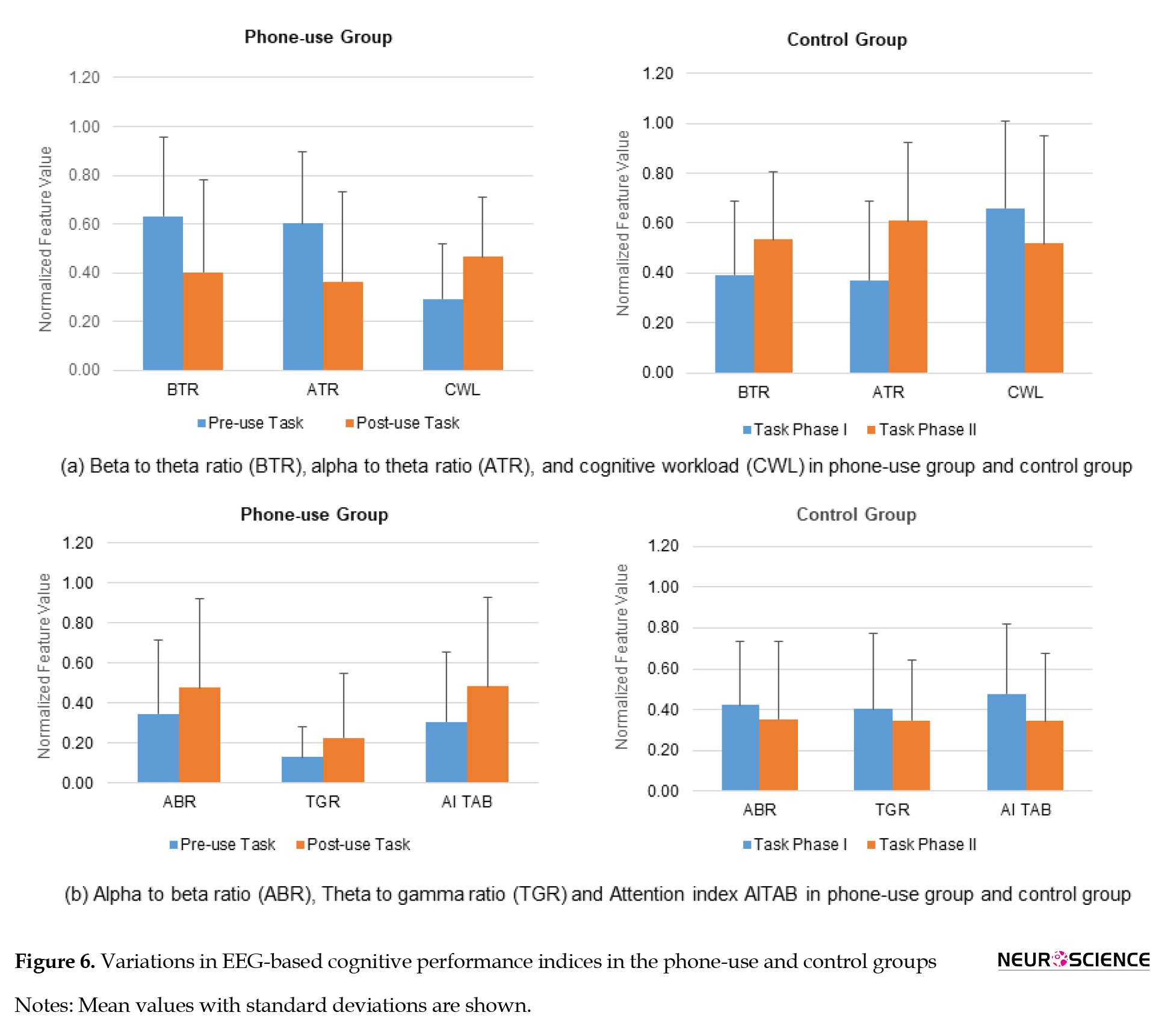
The ATR, representing the performance enhancement index (Gruzelier, 2009), decreased (39.57%) in the phone-use group while increased (64.37%) in the control group. The ABR increased in the phone-use group (37.79%) and decreased in the control group (16.37%). As the increase of beta band power and the decrease of alpha band power are associated with a higher level of certain cognitive skills, such as alertness, the decrease in ABR indicates a higher cognitive performance (Jap et al., 2009).
The BTR decreased (36.15%) in the phone-use group, while it increased (36.34%) in the control group. BTR decreased in all lobes following phone use during the learning task. The decreased BTR indicates a lower cognitive functioning performance (Freeman et al., 1999). For the control group, BTR increased across all brain regions. TGR increased in the phone-use group (73.04%) while decreased in the control group (14.22%). An increase in TGR was shown to be associated with poor cognitive functioning (Moretti et al., 2009). The increase in TGR was high in the phone-use group, indicating a cognitive decline, as shown in
Figure 6.
CWL, a task complexity measure (Gevins & Smith, 2003), increased by 58.80% during the learning task following phone use (
Figure 6). It decreased by 21.33% in the control group, indicating a lower mental workload. The current study also observed an increase (57.55%) in attention index AITAB during the learning task following phone use, as shown in
Figure 6. AITAB decreased by 28.04% for the control group in all lobes. It has been shown that an increase in attention index AITAB represents a lower attentive state (Suhail et al., 2021).
A decrease in sample entropy was observed during the learning task following phone use. The decrease was significantly larger in the right frontal (52.01%) and left occipital (47.83%) regions. It has been demonstrated that sample entropy increases during the attentive state (Ming et al., 2009). For the control group, sample entropy increased in frontal and left occipital regions (2.47%-4.88%) while decreasing in all other lobes (18.51%-36.48%), as indicated in
Figure 7.
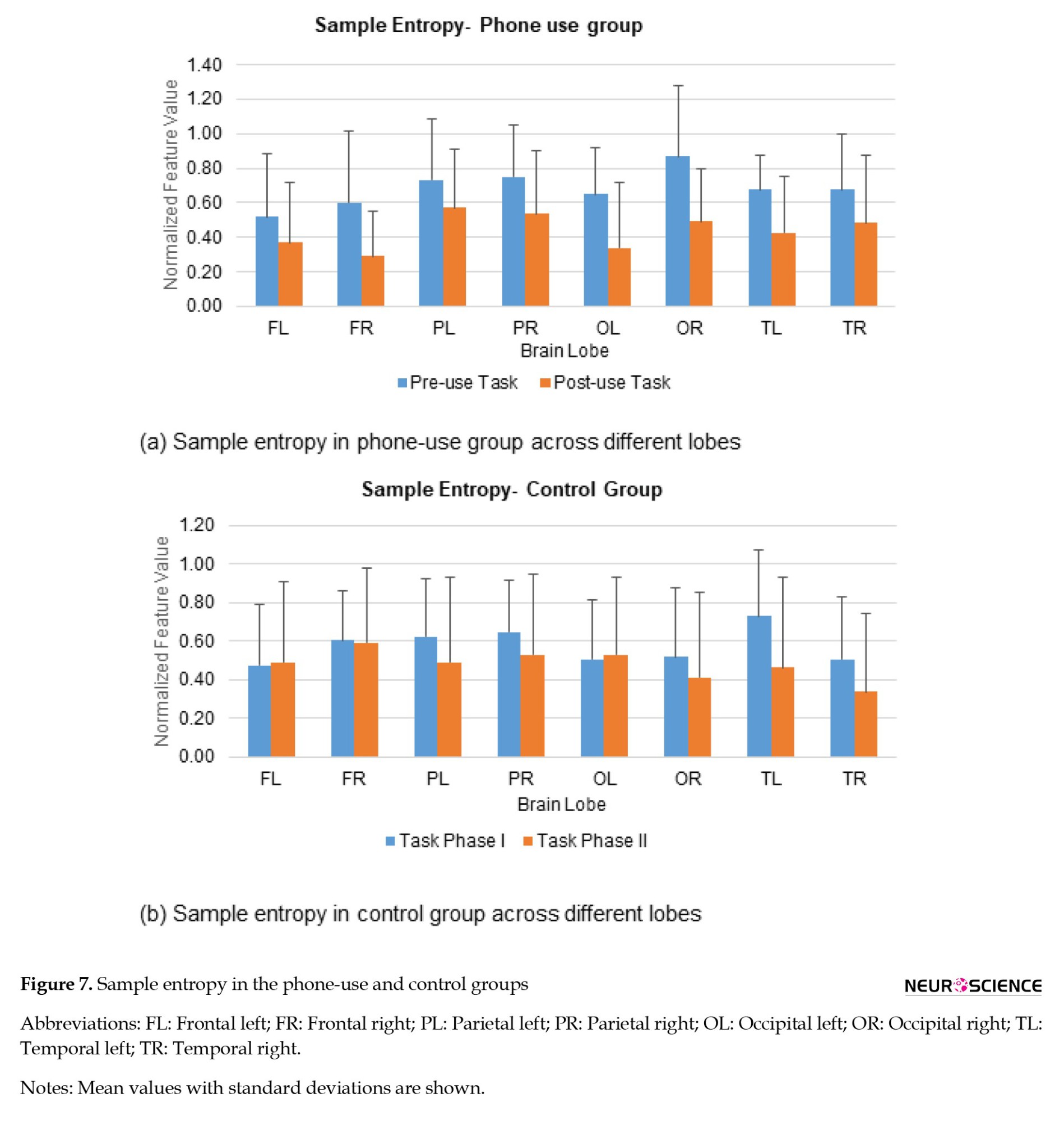
The statistical analysis revealed that BATR and CWL exhibited a statistically significant difference (P<0.05) in the phone-use group (between the learning tasks before and after smartphone use). For the control group, the features exhibited no significant differences. The evaluation of various cognitive performance indices between the phone-use group and control group suggests that smartphone use (or distractions) during cognitive tasks adversely affects the cognitive performance of individuals.
4. Discussion
The present study examined the patterns of smartphone use among young people and the impact of smartphone usage on cognitive performance. Several studies have addressed the adverse effects of heavy media multitasking on the brain’s cognitive functioning. Most relied on self-report questionnaire surveys (Abi-Jaoudeet al., 2020; Uncapher et al., 2017), while a few utilized neuroimaging techniques like fMRI (Moisala et al., 2016). The current study adopted two techniques: a questionnaire survey and an EEG-based analysis to measure smartphone use’s effects on cognitive performance. Compared to the fMRI technique, EEG provides a very high temporal resolution in the order of milliseconds so that any minute variation in brain waves can be captured using EEG. In this work, the patterns of smartphone usage among different groups of people, such as students and working professionals, were investigated using a questionnaire survey. The survey responses indicated that 71.87% of respondents frequently used their smartphones (other than for study/work purposes) during their studies or working hours. Among these multitaskers, 55.01% expressed that their academic/job performance was degraded by smartphone use. Excessive use of smartphones has also been implicated in stress and sleep problems. The results are consistent with previous studies (Abi-Jaoudeet al., 2020; Hou et al., 2019) that showed the adverse effects of heavy media multitasking or excessive use of smartphones on academic performance and mental/physical health. In addition, EEG spectral analysis also indicated a significant difference in cognitive performance indices during tasks following smartphone use. The study used various EEG-based cognitive performance indices, including band ratios, sample entropy, CWL, and attention index. All these features were first evaluated during resting and attentive states. The increases in ATR, BATR, and BTR were observed during the attentive state, consistent with the neuroscientific literature (Freeman et al., 1999; Gruzelier, 2009; Rabbi et al., 2009).
The EEG-based cognitive performance indices analysis in the phone-use and control groups signified that multitasking or switching between learning and phone use negatively affects cognitive performance. In a neuroimaging study using fMRI, Moisala et al. (2016) demonstrated that media multitasking is associated with behavioral distractibility and poor task performance in adolescents. Various mental and physical ill-effects such as headache, mental fatigue, and sleep problems were reported due to cell phone use in an EEG-based study (Parmar et al., 2019). However, EEG-based performance indices have not been widely explored to analyze the impact of smartphone distractions on cognitive performance. Various EEG features representing attentional resource index, performance enhancement index, CWL, and so on were utilized in this study. The experimental results of EEG analysis revealed a decrease in the BATR in the phone-use group, indicating a lower cognitive performance and attentional resource index (Rabbi et al., 2009). The phone-use group has also observed a decrease in the ATR (performance enhancement index) and BTR (attentional control). The variations in these indices occurring due to phone distractions or multitasking indicated a significant decline in cognitive performance.
The current study’s findings indicate that media multitasking during learning or working hours can negatively affect the brain’s cognitive functioning. The current study considered only one type of cognitive task and one smartphone application, such as social media, which is a limitation of this work. Future work will investigate the impact of various smartphone applications, like social media and gaming, on cognitive tasks such as working memory, attentive tasks, and so on. It is also important to focus on developing neurofeedback-based recovery methods for maintaining and enhancing the cognitive performance of individuals with poor cognitive capabilities.
5. Conclusion
The present study investigated the acute impacts of smartphone distractions on cognitive task performance using a questionnaire survey and a set of EEG-based performance indices. Multiple EEG features, such as band ratios, sample entropy, and attention index, were used in this experiment. The survey responses indicated that overuse of smartphones is related to declines in learning performance and work efficacy. Furthermore, the experimental results of EEG analysis showed that smartphone use (or media multitasking during learning) induces significant differences in cognitive performance indices. Taken together, the findings emphasize the need for controlling the use of smartphones during study/work hours. The current study focused on the impact of a social networking site on a learning task. Future work will focus on the effects of various smartphone applications on different cognitive tasks. The relevance of neurofeedback techniques in improving cognitive capabilities deserves special mention in this context.
Ethical Considerations
Compliance with ethical guidelines
The study was approved by the Institutional Ethics Committee of the Institute for Communicative and Cognitive Neurosciences, Shoranur, India, duly constituted according to the guidelines of the Indian Council of Medical Research. The procedure was explained in detail, and written informed consent was obtained from all the participants.
Funding
This research was supported by the research project funded by Cognitive Science Research Initiative, Department of Science and Technology, Government of India (CODE: SR/CSRI/135/2014[G]).
Authors' contributions
All authors equally contributed to preparing this article.
Conflict of interest
The authors declared no conflict of interest.
Acknowledgments
The authors are deeply grateful to Ananthakrishnan C G., Sumitha K P, and Rahna Parakkal at the Institute for Communicative and Cognitive Neuroscience, Shoranur, India, for their valuable support in recording EEG data.
References
Abi-Jaoude, E., Naylor, K. T., & Pignatiello, A. (2020). Smartphones, social media use and youth mental health. CMAJ, 192(6), E136–E141. [DOI:10.1503/cmaj.190434] [PMID]
Arns, M., Van Luijtelaar, G., Sumich, A., Hamilton, R., & Gordon, E. (2007). Electroencephalographic, personality, and executive function measures associated with frequent mobile phone use. The International Journal of Neuroscience, 117(9), 1341–1360. [DOI:10.1080/00207450600936882] [PMID]
Borghini, G., Aricò, P., Graziani, I., Salinari, S., Sun, Y., & Taya, F., et al. (2016). Quantitative assessment of the training improvement in a motor-cognitive task by using EEG, ECG and EOG signals. Brain Topography, 29(1), 149–161. [DOI:10.1007/s10548-015-0425-7] [PMID]
Borghini, G., Astolfi, L., Vecchiato, G., Mattia, D., & Babiloni, F. (2014). Measuring neurophysiological signals in aircraft pilots and car drivers for the assessment of mental workload, fatigue and drowsiness. Neuroscience and Biobehavioral Reviews, 44, 58–75. [DOI:10.1016/j.neubiorev.2012.10.003] [PMID]
Britton, J. W., Frey, L. C., Hopp, J. L., Korb, P., Koubeissi, M. Z., & Lievens, W. E., et al. (2016). Electroencephalography (EEG): An introductory text and atlas of normal and abnormal findings in adults, children, and infants. Chicago: American Epilepsy Society; 2016. [DOI:10.5698/978-0-9979756-0-4]
Chiang, J. T., Chang, F. C., Lee, K. W., & Hsu, S. Y. (2019). Transitions in smartphone addiction proneness among children: The effect of gender and use patterns. Plos One, 14(5), e0217235. [DOI:10.1371/journal.pone.0217235] [PMID]
Clayton, M. S., Yeung, N., & Cohen Kadosh, R. (2015). The roles of cortical oscillations in sustained attention. Trends in Cognitive Sciences, 19(4), 188–195. [DOI:10.1016/j.tics.2015.02.004] [PMID]
Croft, R. J., Hamblin, D. L., Spong, J., Wood, A. W., McKenzie, R. J., & Stough, C. (2008). The effect of mobile phone electromagnetic fields on the alpha rhythm of human electroencephalogram. Bioelectromagnetics, 29(1), 1–10. [DOI:10.1002/bem.20352] [PMID]
Darcin, A. E., Noyan, C., Nurmedov, S., Yilmaz, O., & Dilbaz, N. (2015). Smartphone addiction in relation with social anxiety and loneliness among university students in Turkey. European Psychiatry, 30(Supplement 1), 28-31. [DOI:10.1016/s0924-9338(15)30398-9]
Djamal, E. C., Pangestu, D. P., & Dewi, D. A. (2016). EEG-based recognition of attention state using wavelet and support vector machine. Paper presented at: 2016 International Seminar on Intelligent Technology and Its Applications (ISITIA), Lombok, Indonesia, 30 July 2016. [DOI:10.1109/isitia.2016.7828648]
Farnsworth, B. (2018). What is EEG (Electroencephalography) and How Does it Work? [Internet]. Retrieved from: [Link]
Fraschini, M., Demuru, M., Crobe, A., Marrosu, F., Stam, C. J., & Hillebrand, A. (2016). The effect of epoch length on estimated EEG functional connectivity and brain network organisation. Journal of Neural Engineering, 13(3), 036015. [DOI:10.1088/1741-2560/13/3/036015] [PMID]
Freeman, F. G., Mikulka, P. J., Prinzel, L. J., & Scerbo, M. W. (1999). Evaluation of an adaptive automation system using three EEG indices with a visual tracking task. Biological Psychology, 50(1), 61–76. [DOI:10.1016/s0301-0511(99)00002-2] [PMID]
Gevins, A., & Smith, M. E. (2003). Neurophysiological measures of cognitive workload during human-computer interaction. Theoretical Issues in Ergonomics Science, 4(1-2), 113-131. [DOI:10.1080/14639220210159717]
Gevins, A., Smith, M. E., McEvoy, L., & Yu, D. (1997). High-resolution EEG mapping of cortical activation related to working memory: Effects of task difficulty, type of processing, and practice. Cerebral cortex, 7(4), 374–385. [DOI:10.1093/cercor/7.4.374] [PMID]
Gotlib, I. H. (1998). EEG alpha asymmetry, depression, and cognitive functioning. Cognition & Emotion, 12(3), 449-478. [DOI:10.1080/026999398379673]
Gruzelier J. (2009). A theory of alpha/theta neurofeedback, creative performance enhancement, long distance functional connectivity and psychological integration. Cognitive Processing, 10(Suppl 1), S101–S109. [DOI:10.1007/s10339-008-0248-5] [PMID]
Hart, S. G., & Staveland, L. E. (1988). Development of NASA-TLX (task load index): Results of empirical and theoretical research. Advances in Psychology, 52, 139-183. [DOI:10.1016/s0166-4115(08)62386-9]
Holm, A., Lukander, K., Korpela, J., Sallinen, M., & Müller, K. M. (2009). Estimating brain load from the EEG. TheScientificWorldJournal, 9, 639–651. [DOI:10.1100/tsw.2009.83] [PMID]
Hou, Y., Xiong, D., Jiang, T., Song, L., & Wang, Q. (2019). Social media addiction: Its impact, mediation, and intervention. Cyberpsychology, 13(1), 1-17. [DOI:10.5817/cp2019-1-4]
Indiradevi, K. P., Elias, E., Sathidevi, P. S., Dinesh Nayak, S., & Radhakrishnan, K. (2008). A multi-level wavelet approach for automatic detection of epileptic spikes in the electroencephalogram. Computers in Biology and Medicine, 38(7), 805–816. [DOI:10.1016/j.compbiomed.2008.04.010] [PMID]
Jap, B. T., Lal, S., Fischer, P., & Bekiaris, E. (2009). Using EEG spectral components to assess algorithms for detecting fatigue. Expert Systems With Applications, 36(2), 2352-2359. [DOI:10.1016/j.eswa.2007.12.043]
Krause, C. M., Björnberg, C. H., Pesonen, M., Hulten, A., Liesivuori, T., & Koivisto, M., et al. (2006). Mobile phone effects on children's event-related oscillatory EEG during an auditory memory task. International Journal of Radiation Biology, 82(6), 443–450. [DOI:10.1080/09553000600840922] [PMID]
Li, K. G., Shapiai, M. I., Adam, A., & Ibrahim, Z. (2016). Feature scaling for EEG human concentration using particle swarm optimization. Paper presented at: 2016 8th International Conference on Information Technology and Electrical Engineering (ICITEE), Yogyakarta, Indonesia, 6 October 2016. [DOI:10.1109/iciteed.2016.7863292]
Liu, N. H., Chiang, C. Y., & Chu, H. C. (2013). Recognizing the degree of human attention using EEG signals from mobile sensors. Sensors, 13(8), 10273–10286. [DOI:10.3390/s130810273] [PMID]
Mallat, S. (1999). VI-Wavelet zoom, In S. Mallat (Ed.), A wavelet tour of signal processing (pp. 163-219). Cambridge: Academic Press. [DOI:10.1016/B978-012466606-1/50008-8]
Ming, D., Zhang, M., Xi, Y., Qi, H., Hu, Y., & Luk, K. D. K. (2009). Multiscale entropy analysis of attention ralated EEG based on motor imaginary potential. Paper presented at: 2009 IEEE International Conference on Computational Intelligence for Measurement Systems and Applications, Hong Kong, China, 13 May 2009. [DOI:10.1109/cimsa.2009.5069911]
Missonnier, P., Deiber, M. P., Gold, G., Millet, P., Gex-Fabry Pun, M., & Fazio-Costa, L., et al. (2006). Frontal theta event-related synchronization: Comparison of directed attention and working memory load effects. Journal of Neural Transmission, 113(10), 1477–1486. [DOI:10.1007/s00702-005-0443-9] [PMID]
Moisala, M., Salmela, V., Hietajärvi, L., Salo, E., Carlson, S., Salonen, O., & Lonka, K., et al. (2016). Media multitasking is associated with distractibility and increased prefrontal activity in adolescents and young adults. NeuroImage, 134, 113–121. [DOI:10.1016/j.neuroimage.2016.04.011] [PMID]
Moretti, D. V., Fracassi, C., Pievani, M., Geroldi, C., Binetti, G., & Zanetti, O., et al. (2009). Increase of theta/gamma ratio is associated with memory impairment. Clinical Neurophysiology, 120(2), 295–303. [DOI:10.1016/j.clinph.2008.11.012] [PMID]
Mühl, C., Jeunet, C., & Lotte, F. (2014). EEG-based workload estimation across affective contexts. Frontiers in Neuroscience, 8, 114. [DOI:10.3389/fnins.2014.00114] [PMID]
Noachtar, S., Binnie, C., Ebersole, J., Mauguière, F., Sakamoto, A., & Westmoreland, B. (1999). A glossary of terms most commonly used by clinical electroencephalographers and proposal for the report form for the EEG findings. The International Federation of Clinical Neurophysiology. Electroencephalography and clinical neurophysiology. Supplement, 52, 21–41. [PMID]
Parmar, K., Tandon, R., Kumar, N., & Garg, R. K. (2019). Variations in electroencephalography with mobile phone usage in medical students. Neurology India, 67(1), 235–241.[DOI:10.4103/0028-3886.253610] [PMID]
Peng, C. J., Chen, Y. C., Chen, C. C., Chen, S. J., Cagneau, B., & Chassagne, L. (2020). An EEG-based attentiveness recognition system using hilbert-huang transform and support vector machine. Journal of Medical and Biological Engineering, 40(2), 230-238. [DOI:10.1007/s40846-019-00500-y]
Polikar, R. (1999). The story of wavelets. In R. Polikar (Ed.), Physics and modern topics in mechanical and electrical engineering (pp. 192-197). Marathon Beach: World Scientific and Engineering Academy and Society. [Link]
Schoenberg, P. L., & Speckens, A. E. (2015). Multi-dimensional modulations of α and γ cortical dynamics following mindfulness-based cognitive therapy in major depressive disorder. Cognitive Neurodynamics, 9(1), 13–29. [DOI:10.1007/s11571-014-9308-y] [PMID]
Posner, M. I., & Petersen, S. E. (1990). The attention system of the human brain. Annual Review of Neuroscience, 13, 25–42.[DOI:10.1146/annurev.ne.13.030190.000325] [PMID]
Rabbi, A. F., Ivanca, K., Putnam, A. V., Musa, A., Thaden, C. B., & Fazel-Rezai, R. (2009). Human performance evaluation based on EEG signal analysis: A prospective review. Annual International Conference of the IEEE Engineering in Medicine and Biology Society, 2009, 1879–1882. [DOI:10.1109/iembs.2009.5333877] [PMID]
Recarte, M. A., & Nunes, L. M. (2003). Mental workload while driving: Effects on visual search, discrimination, and decision making. Journal of Experimental Psychology, 9(2), 119–137. [DOI:10.1037/1076-898x.9.2.119] [PMID]
Richman, J. S., & Moorman, J. R. (2000). Physiological time-series analysis using approximate entropy and sample entropy. American Journal of Physiology. Heart and Circulatory Physiology, 278(6), H2039–H2049. [DOI:10.1152/ajpheart.2000.278.6.h2039] [PMID]
Rosenberg, M. D., Finn, E. S., Scheinost, D., Papademetris, X., Shen, X., & Constable, R. T., et al. (2016). A neuromarker of sustained attention from whole-brain functional connectivity. Nature, 19(1), 165–171. [DOI:10.1038/nn.4179] [PMID]
Saxe, R., & Kanwisher, N. (2003). People thinking about thinking people. The role of the temporo-parietal junction in "theory of mind". NeuroImage, 19(4), 1835–1842. [DOI:10.1016/s1053-8119(03)00230-1] [PMID]
Schurz, M., Maliske, L., & Kanske, P. (2020). Cross-network interactions in social cognition: A review of findings on task related brain activation and connectivity. Cortex, 130, 142–157. [DOI:10.1016/j.cortex.2020.05.006] [PMID]
Shaffer H. J. (1996). Understanding the means and objects of addiction: Technology, the internet, and gambling. Journal of Gambling Studies, 12(4), 461–469. [DOI:10.1007/bf01539189] [PMID]
Scheff, S. W. (2016). Nonparametric statistics. In S. W. Scheff (Ed.), Fundamental statistical principles for the neurobiologist: A survival guide (pp. 157.182). Amsterdam: Elsevier. [DOI:10.1016/B978-0-12-804753-8.00008-7]
Soni, R., Upadhyay, R., & Jain, M. (2017). Prevalence of smart phone addiction, sleep quality and associated behaviour problems in adolescents. International Journal of Research in Medical Sciences, 5(2), 515-519. [DOI:10.18203/2320-6012.ijrms20170142]
Suhail, T. A., Indiradevi, K. P., Suhara, E. M., Suresh, P. A., & Anitha, A. (2021). Electroencephalography based detection of cognitive state during learning tasks: An extensive approach. Cognition Brain Behavior, 25(2), 157-178. [DOI:10.24193/cbb.2021.25.08]
Tattersall, A. J., & Foord, P. S. (1996). An experimental evaluation of instantaneous self-assessment as a measure of workload. Ergonomics, 39(5), 740–748. [DOI:10.1080/00140139608964495] [PMID]
Thomée, S., Härenstam, A., & Hagberg, M. (2012). Computer use and stress, sleep disturbances, and symptoms of depression among young adults-A prospective cohort study. BMC Psychiatry, 12, 176. [DOI:10.1186/1471-244x-12-176] [PMID]
Uncapher, M. R., Lin, L., Rosen, L. D., Kirkorian, H. L., Baron, N. S., & Bailey, K., et al. (2017). Media multitasking and cognitive, psychological, neural, and learning differences. Pediatrics, 140(Suppl 2), S62–S66. [DOI:10.1542/peds.2016-1758d] [PMID]
Van Der Schuur, W. A., Baumgartner, S. E., Sumter, S. R., & Valkenburg, P. M. (2015). The consequences of media multitasking for youth: A review. Computers in Human Behavior, 53, 204-215. [DOI:10.1016/j.chb.2015.06.035]
Van Deursen, A. J., Bolle, C. L., Hegner, S. M., & Kommers, P. A. (2015). Modeling habitual and addictive smartphone behavior: The role of smartphone usage types, emotional intelligence, social stress, self-regulation, age, and gender. Computers in Human Behavior, 45, 411-420. [DOI:10.1016/j.chb.2014.12.039]
Wang, X. W., Nie, D., & Lu, B. L. (2014). Emotional state classification from EEG data using machine learning approach. Neurocomputing, 129, 94-106. [DOI:10.1016/j.neucom.2013.06.046]
Wolf, I., Dziobek, I., & Heekeren, H. R. (2010). Neural correlates of social cognition in naturalistic settings: A model-free analysis approach. NeuroImage, 49(1), 894–904. [DOI:10.1016/j.neuroimage.2009.08.060] [PMID]