1. Introduction
utism spectrum disorder (ASD) as a neurodevelopmental disorder is characterized by deficits in communication and reciprocal social interaction and restricted, repetitive, or stereotyped patterns of behavior (
McAlonan et al., 2008). Whereas Asperger syndrome (AS) is a distinct type of ASD that shares most of the clinical features of classical ASD but has significant language delay (
Gilchrist et al., 2001). There are also behavioral differences between ASD and AS, like performance intelligence quotient (IQ) (
Szatmari, et al., 1995), motor performance (
Volkmar, et al., 1998), emotion perception (
Mazefsky & Oswald, 2007), empathy (
Montgomery et al., 2016), sensory experience (
Bogdashina, 2016), executive function, the severity of repetitive behavior (
Ozonoff, et al., 2000), and development of the theory of mind (
Montgomery et al., 2016). Differences in cognitive, language, school functioning, and comorbidity were also found between AS and ASD (
de Giambattista et al., 2019;
Bi, et al., 2018). Neuroimaging studies have linked these traits to structural abnormalities in the brain. For example, Recent studies have reported brain structural abnormalities in the frontal, temporal, parietal, and striothalamic networks in ASD (
Ecker et al., 2017). Increased gray matter (GM) in angular gyrus (
Liu et al., 2017) and regions, including default mode network (
Uddin et al., 2011), as well as less GM in the right paracingulate sulcus, left inferior frontal gyrus (
Abell et al., 1999) and cerebellum (
D’Mello, et al., 2016) has also been reported in ASD. GM volume reduction in the striatum and amygdala/hippocampus has been frequently reported in ASD (
Van Rooij et al., 2018). One of the recent meta-analyses of voxel-based morphometry studies in over 900 ASD patients, found GM volume decrease in the medial prefrontal cortex (mPFC) and posterior insula and an increase in left anterior temporal, right inferior temporoparietal, left dorsolateral prefrontal (DLPFC), and precentral cortices (
Carlisi et al., 2017). Other studies considered the relationship between white matter (WM) and GM. For example, Cauda found positive concordance of WM and GM in the left hemisphere and negative concordance in the right hemisphere in ASD (
Cauda et al., 2014).
Studies on Asperger syndrome have also shown GM abnormality in the cingulate gyrus (
Kwon, et al., 2004), cerebellum, putamen, precuneus, and amygdala (Kevin & McAlonan, 2011). Although GM abnormalities have been reported in ASD and AS individuals, much less is known about the differentiation between them (
Khosrowabadi, et al., 2015;
Sadeghi et al., 2017b). Faridi and Khosrowabadi reviewed some of the important factors, including the effect of age, gender, and IQ on Asperger syndrome. In their study, the effect of each factor on behavioral, chemical, and brain structural changes is discussed (
Faridi & Khosrowabadi, 2017).
The grey matter changes are region-specific (
Stacey et al., 2017;
Kobayashi et al., 2020). Therefore, covariation of regional changes could introduce another measure known as structural covariance (SC) (
Mechelli, et al.e, 2005). The SC is mainly based on the phenomenon that inter-individual differences in a brain region often covariate with other brain regions that simultaneously fluctuate (
Evans, 2013). Pathological structural covariance has been demonstrated in cases of ASD. For example, large-scale disruption of the frontotemporal SC network in an individual with ASD which play a central role in language and communication has been demonstrated (
Sharda, et al., 2016). Other studies have reported a disrupted structural correlation between brain regions that are responsible for social functions in autism (
McAlonan et al., 2005;
Dziobek, et al., 2010). Reduced local and increased long-ranged functional connectivity of the thalamus (
Tomasi & Volkow, 2019), insula (
Guo et al., 2019), and amygdala (
Guo et al., 2016) have also been identified. Moreover, decreased inter-hemispheric and enhanced intra-hemispheric structural covariation have been shown in recent studies (
Duan et al., 2020). One structural covariance study of sensory networks demonstrated decreased structural covariation between sensory-related cortical structures, especially between the left and right cerebral hemispheres, but increased structural covariance of the structure in the right cerebral hemisphere in individuals with ASD (
Cardon, et al., 2017).
However, it needs to be investigated whether the SC varies between ASD and AS. So, this study hypothesized that structural grey matter changes vary in ASD, AS, and healthy control (HC) groups. To test the hypothesis, grey matter changes were estimated from MRI scans in ASD, AS and HC groups. After the calculation of regional GM density, the covariation of GM changes between pairs of brain regions was calculated. Subsequently, statistical analysis was performed to highlight the structural covariance changes among the groups.
2. Materials and Methods
Study participants
A total of 42 subjects with a DSM-IV-TR diagnosis of autism, including 16 AS (Mean±SD age=24.31±15.810 years, IQ=111.31±11.241) and 26 ASD (Mean±SD age=24.67±8.347 years, IQ=110.26±12.09) with 28 HC (mean ± SD age=22.13± 8.579 years, IQ=115.92±12.66) participants were studied. All subjects were male and right-hand dominant. ASD and HC subjects were selected from the dataset of USM (University of Utah School of Medicine) and AS subjects were selected from the dataset of LMU (Ludwig Maximilian University Munich). A summary of the demographic characteristics of each group is provided in
Table 1.
.jpg)
Informed written consent was obtained for all participants and the procedure was approved by the human investigation Review Board at the University of Utah School of Medicine and Ludwig Maximilian University Munich. Estimates of full IQ above 92 and absence of other chronic medical conditions were required for all subjects. Additionally, these measures are all correlated with the Wechsler Abbreviated Scale of Intelligence.
Data acquisition
All participants underwent a T1-weighted high-resolution MRI scanning using a 3 Tesla Siemens scanner system (SIMENS MAGNETOM TrioTim syngo MR B17) with the following protocol: T1=900 ms; Flip angle=9º; TR=2300 ms; TE=2.91 ms; Slices=160; Orientation=Sagittal; Slice thickness=1.20 mm; and voxel size=1.0×1.0×1.2 mm. All imaging data used are publicly available at http://fcon_1000.projects.nitrc.org/indi/abide/abide_I.html.
Magnetic resonance imaging (MRI) data processing
Standard preprocessing was performed on MRI data using the FMRIB software library (FSL: http://www.fmrib.ox.ac.uk/fsl) (
Jenkinson, et al., 2012) and analysis of functional neuroimaging (AFNI: http://afni.nimh.nih.gov/afni) (
Cox, 1996). All participants’ structural images were checked for scanner and individual-based motion artifacts. Then, images were deobliqued before reorientation to FSL-friendly space. Subsequently, individual images were affine registered to Montreal Neurological Institute and Hospital (MNI) space and then corrected for bias-field in homogeneities. Next, MRI images were segmented into different tissue types through the FSL FMRIB’s Automated Segmentation Tool (FAST) by partial volume modeling. All images were then spatially normalized. Afterward, the MRI grey matter segment of each subject was parcellated into 116 regions of interest (ROI) using MNI-normalized automated anatomical labelling (AAL) atlas. Therefore, 116 ROIs were acquired for each participant that present the average GM density at the specified region of the brain. Finally, a region-wise statistical analysis was performed across different groups (
Figure 1).
Statistical analysis
Differential pattern of brain anatomical changes in AS, ASD, and HC groups
In this stage, the grey matter density of each ROI for each group individually was statistically compared to the same ROI in another group using a 2-sample t test. The comparison was performed for each pair of groups separately. Since the comparisons were performed for each ROI separately, a correction was also performed for multiple comparison effects. Statistical analyses were performed using the statistical toolbox of MATLAB 2013a software. A significance level of P<0.005, corrected for false discovery rate (FDR), was considered for presenting the results.
Construction of structural connectivity network (SCN)
The covariance of GM density between the brain regions was calculated for each group separately. GM density of all 116 anatomical regions for each individual was used to construct the structural connectivity network (SCN). For each group, an association matrix (N×116, N=number of samples) was implied to generate the SCN (R=116×116) by computing the Pearson correlation (ri,j) between the vector of GM density in region i and region j across participants. The extracted SCN of the AS group was then statistically compared to the SCNs of ASD and HC groups.
Comparing between SCNs of AS, ASD, and HC groups
The SCNs were extracted based on similarities of the regional GM density. The correlation coefficients of each group were compared to another using the ‘cocor’ software package (version 1.1, Fisher’s Z test 1925) written in the R 3.2.5 programming language. In this research, the correlation coefficients (r, p considering the sample size) of each group was calculated using MATLAB Software. Then, statistical analysis was conducted between each pair of groups in R statistical software by transferring r values to z values using Fisher’s z test and P<0.005 were considered significant results.
3. Results
As presented in
Table 1, there were no significant differences between the groups in age and IQ scores. The regional grey matter density and structural covariance network in AS, ASD, and HC groups was computed based on the subjects’ MRI data. Subsequently, differences between the groups were calculated by statistical comparison between their measures. Significant differences in the regional GM density between the groups (P<0.05, false discovery rate [FDR] corrected) are presented in
Tables 2 to
4, and
Figure 2.
.jpg)
In addition, structural covariance networks of AS, ASD, and HC groups are presented in
Figure 3 and their significant differences are shown in
Figure 4.
.jpg)
.jpg)
Details of results presented in
Figure 3 are presented in supplementary material in
Tables S1 to S3.
.jpg)
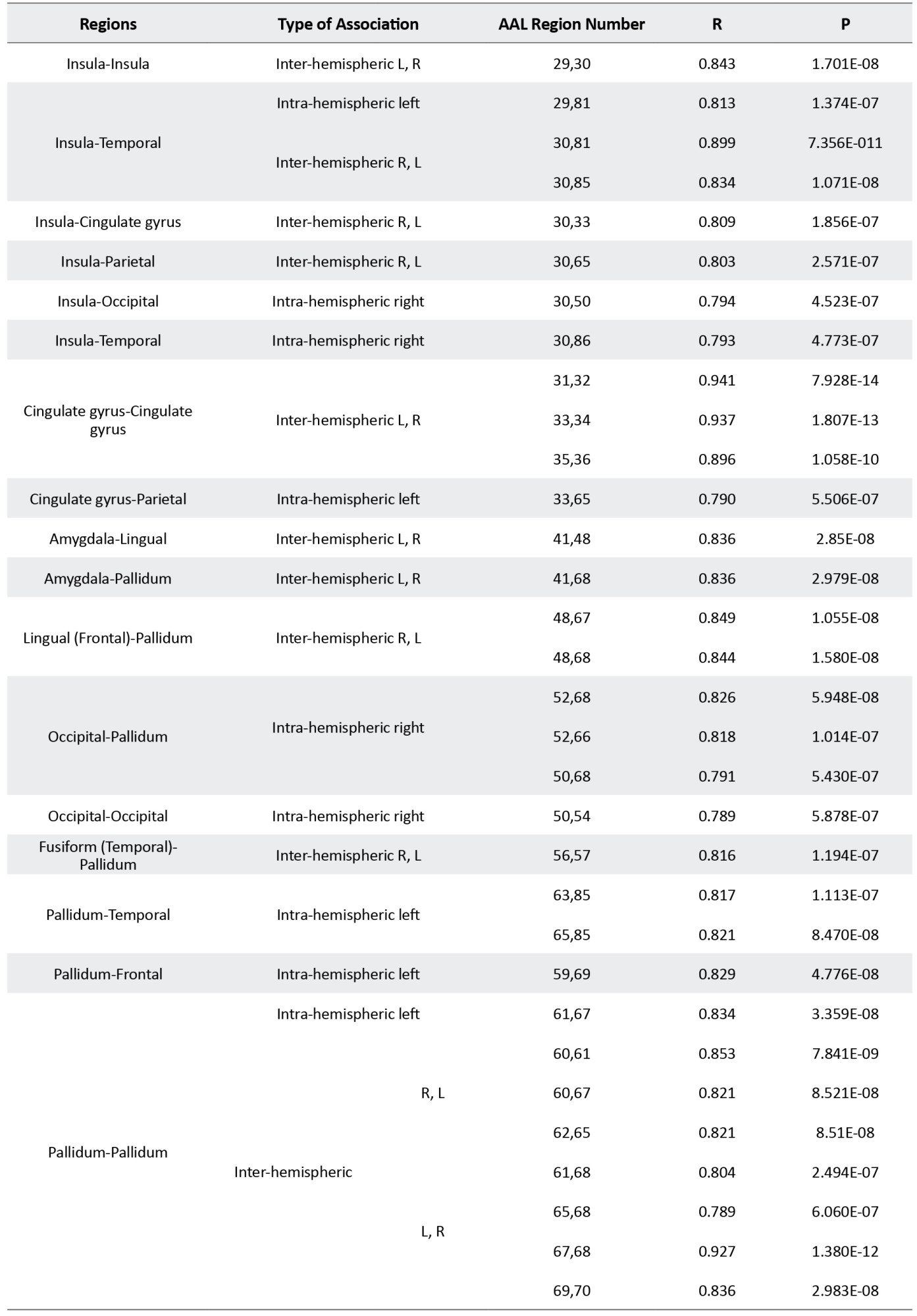

.jpg)

.jpg)
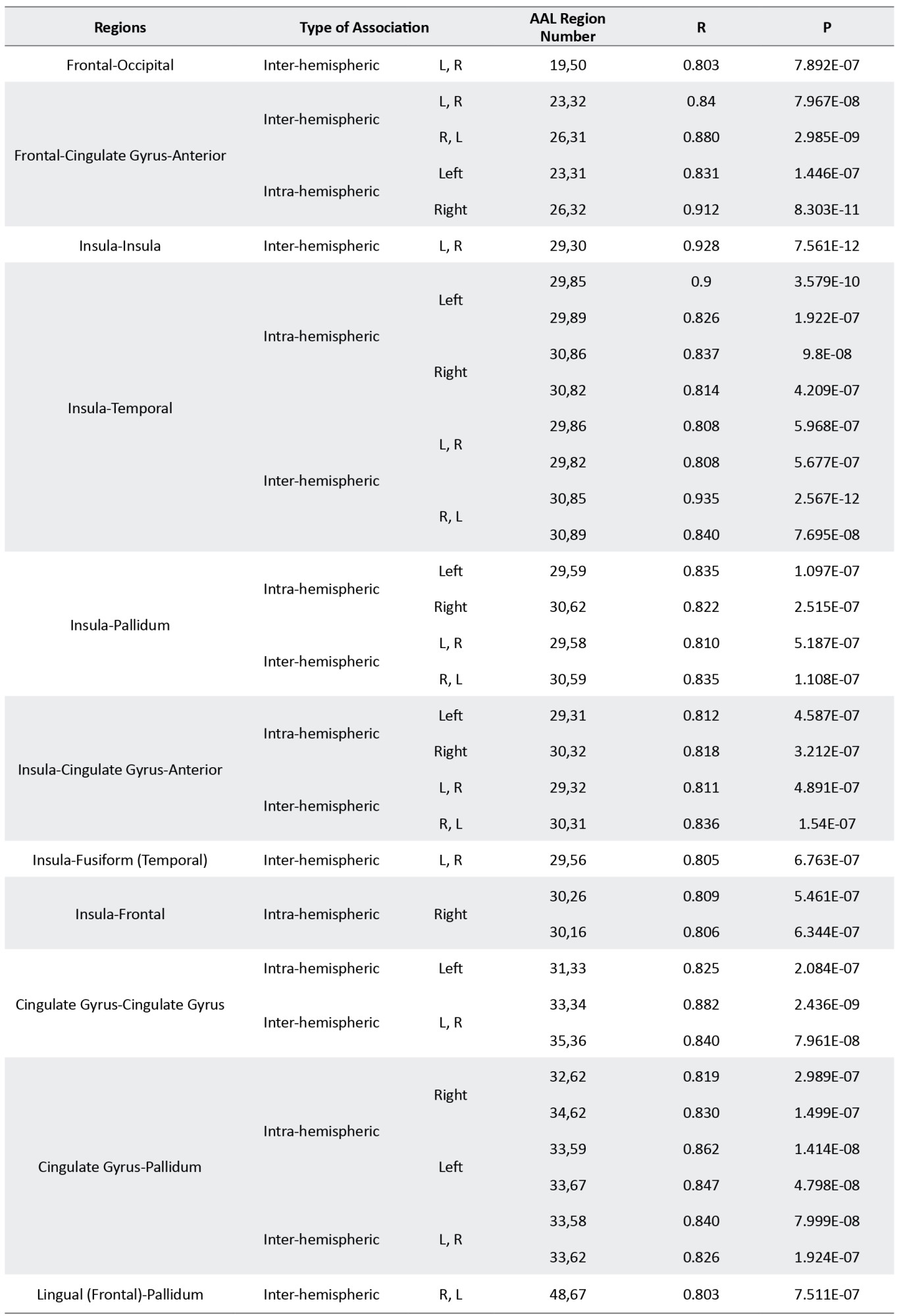
Differential pattern of grey matter density in AS, ASD, and HC groups
ASD group compared to HCs showed an increase in GM density at the precentral and vermis regions (
Table 2,
Figure 2A).
.jpg)
Moreover, AS groups compared to HCs showed raised GM density at the frontal medial orbital and anterior cingulum bilaterally and superior orbitofrontal, middle orbitofrontal, and inferior triangle frontal at the right hemisphere. While decreased GM density was found in the hippocampus, parahippocampal, cuneus, fusiform, caudate, putamen, pallidum, thalamus, heschle, cerebellum crus 1, cerebellum 3, 4-5, 6, 7b, 8, 9 bilaterally, amygdala and cerebellum crus 2 at the right side, olfactory, calcarine, lingual, middle occipital, postcentral at the left side and vermis 3, 4-5, 7, 8, 9 (
Table 3,
Figure 2B).
.jpg)
.jpg)
In addition, AS group compared to the ASD group showed increased GM density at the medial orbitofrontal and anterior cingulum bilaterally, and frontal superior orbital and frontal inferior triangle on the right hemisphere. In contrast, decreased GM density was also found at the olfactory, hippocampus, para-hippocampal, amygdala, cuneus, fusiform, caudate, putamen, pallidum, thalamus, heschle, cerebellum crus 1, cerebellum 3, 4-5, 6, 8, 9 bilaterally, and insula, calcarine, lingual, middle occipital, postcentral at the left side, and cerebellum crus 2, cerebellum 7b at the right side, as well as vermis 3, 4-5, 6,7, 8, 9 (
Table 4,
Figure 2C).
.jpg)

In
Figure 2, increased GM density is shown in red, and decreased GM density is in blue.
Structural covariance network in AS, ASD, and HC
The structural covariance network based on regional GM density in each group is presented in
Figure 3 and their statistical differences are shown in
Figure 4. In
Figure 3, the red color denotes a positive correlation between regions, while the blue color indicates a negative association. Interestingly, all 3 groups mainly showed a significant positive association between the regional GM densities (P<0.05, FEW corrected).
Differential pattern of SCNs in AS, ASD, and HC groups
The Asperger syndrome group as compared to ASD had increased inter-regional correlation at the posterior fossa and excessive intra-regional correlation at frontotemporal and frontoparietal associations. Nevertheless, the correlation between GM density at the posterior fossa and other regions (frontal, temporal, parietal, occipital, insula) was decreased (
Figure 4A). Similar pattern but with the more severe degree was observed in AS individuals versus the HC group (
Figure 4B). Moreover, compared to HC, ASD showed an increased inter-hemispheric correlation of GM density at posterior fossa and frontal regions. In addition, increased intra-hemispheric correlation between GM density of the temporal region and parietal, insula, and posterior fossa regions were observed. However, inter-hemispheric associations between GM density at the temporal region and other brain regions were decreased (
Figure 4C).
4. Discussion
In this study, the alteration of regional grey matter density in autistic and Asperger individuals compared to healthy controls was investigated. In addition, covariation of regional GM density in pairs of 116 anatomically separated regions was also calculated and the group differences were identified. Our analysis revealed significant changes in the regional GM density, as well as the pattern of structural covariance between the 3 above-mentioned groups.
The ASD group compared to the HC group presented higher GM density at the precentral gyrus and vermis. This result is consistent with previous MRI studies (
Hyde, et al., 2010;
Courchesne, et al.n, 1988). The precentral gyrus is believed to be involved in motor control, and the vermis has crucial importance in emotion regulation and emotion processing paradigms, as well as the oculomotor control that all are disrupted in the ASD group (
Kato & Izumiyama, 2015;
Beauregard et al., 1998;
Laidi et al., 2017). The increased GM density at the precentral gyrus in the ASD group might be due to excessive gyrification that has been reported in several studies (
Ecker et al., 2016;
Yang, et al., 2016;
Ecker et al., 2016;
Kohli, 2017). However, the increased GM density at the vermis may be corroborated by the idea of hypoplasia and loss of Purkinje neurons in this region (
Courchesne et al., 1988).
On the other hand, the AS group showed decreased GM density at limbic regions (hippocampus, parahippocampus, amygdala, hypothalamus, thalamus), basal ganglia (putamen, caudate, pallidum), language region (lingual, parietal), heschle, fusiform, and cerebellum compared to HC group. Significant decreased GM density in the limbic regions which plays an important role in sensory-motor gating (
Koch & Bubser, 1994) could infer the weakness of AS group in inhibiting repetitive behaviors (
Hollander et al., 2005). Thalamus is the gateway to the cortex, and almost all sensory information is routed to the cortex through different areas of the thalamus (
Jones, 2009) and abnormal thalamus was associated with social deficits, sensory deficits, and restricted repetitive behavior (
Zuo, et al., 2019). The amygdala and hippocampus are the key components of the medial temporal lobe and are involved in emotional perception and regulation (
Groen, et al., 2010). In addition, abnormal morphology in striatal structures or basal ganglia was reported to be associated with restricted and repetitive behavior (
Van Rooij et al., 2018). These regions are also involved in the modulation of habit learning, action selection and performance (
Jin & Costa, 2015).
Decreased GM density at lingual regions in AS group fits well with the language deficiency in AS individuals. In addition, reduced GM density at the fusiform in AS individuals also support the deficit of this group in face processing (
Rossion et al., 2003). Moreover, decreased GM density at the cerebellum and vermis, can also be a reason for the weakness of AS individuals in emotion regulation and social cognition (
Van Overwalle & Mariën, 2016), eye avoidance (
Laidi et al., 2017), and social and affective processing (
Riva et al., 2013).
Our study represented increased GM density in AS group compared to the HC group at the medial frontal and cingulate. These regions are mainly involved in social orienting and monitoring self-related information (
Mundy, 2003) which are weak in AS and ASD individuals. This contradiction between the increase in GM density and behavioral deficits could be answered by the mechanism of the dopaminergic system. The dopaminergic activity in the medial prefrontal cortex is lower in autistic individuals (
Ernst, et al., 1997). Therefore, the increased GM density in this region could be a compensatory mechanism to balance the drop in dopamine activity. Moreover, at the medial prefrontal cortex, AS patients showed higher GM density than the ASD group. This fact may imply the better performance of AS patients in social orientation and self-reflection than ASD patients (
Ashwin, et al., 2007;
Cauda, Geda, et al., 2011). Moreover, the medial orbitofrontal and anterior cingulum are also involved in expressing fear or anxiety (
Etkin, et al., 2011), which seems to be disrupted in children with autism and Asperger syndrome (
Kim, et al, 2000).
In addition to structural abnormalities in AS and ASD in this study, just as mentioned, our findings also represented a differential pattern between ASD and AS individuals which is still controversial (
McAlonan et al., 2008;
Via, et al, 2011).
In our study, the AS group compared to ASD showed higher GM density at the medial orbitofrontal and the anterior cingulum and lessened GM density in several brain regions, including olfactory, insula, hippocamp, hippocampal gyrus, amygdala, calcarine, cuneus, lingual, occipital, fusiform, postcentral, caudate, putamen, thalamus, heschle, and cerebellum. Interestingly, the AS group, despite less severe behavioral and cognitive performance than ASD, presented more structural alteration (
Courchesne, et al., 2005). Different patterns of changes in GM density in AS group may suggest a compensatory mechanism to maintain a better level of behavioral and cognitive performance by more alteration of the brain GM structure.
The brain structural network has a complex topology that could be better studied using a structural covariance network (
Romero-Garcia et al., 2017). Structural covariance analysis is an effective approach for mapping the inter-regional anatomical association (
Bethlehem, et al., 2017). Evidence from brain connectivity suggests that behavior affected by ASD is subscribed by deficits in distributed brain networks rather than single regions of the brain (
Cardon et al., 2017).
In this study, the regional correlations in the SCNs were mainly positive, and more correlations were observed between regions in the right hemisphere. This lateralized pattern of SCN has been reported in previous studies as well (
Tomasi & Volkow, 2012), (
Kikuchi et al., 2013;
Nielsen et al., 2014).
In the healthy control group, GM density similarities were observed between frontal-insula, frontal-cingulate, and insula-cingulate verified by previous studies (
Soriano-Mas et al., 2013;
Taylor, et al., 2009). Our findings emphasize the idea that functionally related regions are structurally associated (
Damoiseaux & Greicius, 2009;
Nair et al., 2014;
Sui, etal., 2014). For instance, a correlation between GM densities at the insula and temporal region or amygdala and parietal region might be due to high functional connectivity between these regions (
Paulus, et al., 2005;
Bonda, et al., 1996). The correlations observed between GM densities at the amygdala and lingual region nicely fit with former studies relating the amygdala to language ability in normally developing children (
Ortiz-Mantilla, et al., 2010). Interestingly, the GM density similarities were observed between more spatially local regions (inter-lobar). This finding may indicate that closed regions have associative activities in the brain (
Allen, et al., 2002;
Alexander-Bloch et al., 2012;
Eyler et al., 2011; He, Chen, & Evans, 2007). Inter-regional structural covariance reflects the synchronism of their developmental changes, which may be related to the formation and reconstruction of axon connections during development and is regulated by intrinsic nutrition, neurodevelopmental factors, and genetic factors (
Duan et al., 2020). Moreover, hyper-synchronous developmental coordination and maturation between these regions might be linked to the disruption of functional segregation and segregated network properties such as clustering and modularity (
Chen, et al., 2008;
Alexander- Bloch et al., 2013).
In contrast, the distant regions’ similarities, for example, similarities at the occipital insula could reveal the brain integration network (
Vértes et al., 2012).
In the Asperger syndrome group, fewer regional GM similarities were observed compared to ASD and HC groups. Decreased level of anatomical similarities between brain regions in AS group might be the compensatory mechanism (
Butz, et al., 2009) to maintain a desired level of activity (
Rudie et al., 2013), by using functional plasticity and altering synaptic strength.
In the ASD group, our findings showed regional over-anatomical similarities, compared to HC group that has been reported in numerous studies (
Assaf et al., 2010;
Kana, et al., 2011; (
Rudie et al., 2013;
Kennedy & Courchesne, 2008;
Nair et al., 2014;
Rudie et al., 2011;
Dickstein et al., 2013;
Kennedy & Courchesne, 2008). Local overconnectivity (GM density similarities) in SCN of ASD individuals were mainly observed at the frontal and posterior fossa in our study which has been reported in previous studies (
Courchesne & Pierce, 2005;
Keown et al., 2013;
Sahyoun, et al. 2010). A distal pattern of occipitofrontal functional connectivity is related to the symptom severity of ASD (
Jao Keehn et al., 2019). Previous studies suggested excessive connectivity within the frontal lobe, whereas connectivity between the frontal cortex and other systems is poorly synchronized in ASD, which may reflect impairment of the fundamental frontal function of integrating information from widespread and diverse systems (
Courchesne et al., 2007).
Overconnectivity in SCN of ASD was also observed between the insula and cingulate regions. Local overconnectivity at the frontal regions in ASD can be taken as attenuation of cortical differentiation in frontal regions in ASD individuals by enlargement of frontal regions (
Sadeghi et al., 2017a) and reducing the mini-columns (
Buxhoeveden et al., 2006) and widening them (
McKavanagh, et al., 2015;
Vissers, Cohen, & Geurts, 2012). The GM density at the insula showed increased similarities with other brain regions in ASDs. Insula is part of the salience network (
Nelson et al., 2010) that is involved in many cognitive functions, including subjective feelings (
Ebisch et al., 2011), processing vestibular/auditory information (
Cauda, D’agata, et al., 2011), emotions (
Seminowicz & Davis, 2006), language and mood stability (
Flynn, 1999). Because ASD individuals are weak at the above-mentioned functions, we think that attenuation of the insula’s anatomical links with other parts of the brain leads to less efficient function (
Vissers et al., 2012). A similar hypothesis may be applied to our findings in cingulate gyrus that mainly involves the understanding of other’s emotional experiences (
Saarela et al., 2006), emotional salience monitoring, general environmental monitoring, response selection, and body orientation (
Taylor et al., 2009) that could be linked to repetitive and restricted behavior in autism (
Vissers et al., 2012).
5. Conclusions
This study shows the potential of using a pattern of regional grey matter density to differentiate various ASD subtypes. Our findings also indicate the structural covariance network could be used for the discrimination of AS from ASD and HC groups. Less regional GM density together with fewer similar regions in terms of GM density in AS group may suggest a compensatory mechanism to overcome the behavioral dysfunctions in autism. The attenuated structural differentiation could be a reason for less efficient information segregation and integration which lead to cognitive dysfunctions in autism. Our findings provide another insight into understanding the pathology of autism and we hope to be used for intervention purposes.
Study limitation and strength
This study has some limitations. The study was limited to male subjects, and the number of participants varied in each group. Therefore, caution should be emphasized against generalizing the results across different age and gender groups, and the severity of the disease should be considered as well. It should be noted that we only investigated the alteration of the regional GM density and their inter-regional covariations in this study. For sure, using other imaging modalities such as diffusion tensor imaging could improve our understanding of the SCNs by a direct assessment of the structural connectivity.
Ethical Considerations
Compliance with ethical guidelines
All ethical principles are considered in this article. The participants were informed of the purpose of the research and its implementation stages. They were also assured about the confidentiality of their information and were free to leave the study whenever they wished and if desired, the research results would be available to them. A written consent has obtained from the subjects. Principles of the Helsinki convention was also observed.
Funding
This research did not receive any grant from funding agencies in the public commercial, or non-profit sectors.
Authors' contributions
Conceptualization and supervision: Reza Khosrowabadi; Methodology: Afrooz Seyedebrahimi; Investigating and writing-original draft: Farnaz Faridi; Review and edit: All authors; Data collection and analysis: Afrooz Seyedebrahimi and Reza Khosrowabadi.
Conflict of interest
The authors declare no conflict of interest.
Acknowledgments
We would like to thank the Autism Brain Imaging Data Exchange (ABIDE) for generously sharing the data.
References
Abell, F., Krams, M., Ashburner, J., Passingham, R., Friston, K., & Frackowiak, R., et al. (1999). The neuroanatomy of autism: A voxel‐based whole brain analysis of structural scans. Neuroreport, 10(8), 1647-1651. [PMID]
Alexander-Bloch, A. F., Vértes, P. E., Stidd, R., Lalonde, F., Clasen, L., & Rapoport, J., et al. (2013). The anatomical distance of functional connections predicts brain network topology in health and schizophrenia. Cerebral Cortex (New York, N.Y. : 1991), 23(1), 127–138. [PMID] [PMCID]
Alexander-Bloch, A. F., Vértes, P. E., Stidd, R., Lalonde, F., Clasen, L., & Rapoport, J., et al. (2013). The anatomical distance of functional connections predicts brain network topology in health and schizophrenia. Cerebral Cortex, 23(1), 127-138. [PMID] [PMCID]
Allen, J. S., Damasio, H., & Grabowski, T. J. (2002). Normal neuroanatomical variation in the human brain: An MRI-volumetric study. American Journal of Physical Anthropology, 118(4), 341–358. [PMID]
Ashwin, C., Baron-Cohen, S., Wheelwright, S., O'Riordan, M., & Bullmore, E. T. (2007). Differential activation of the amygdala and the ‘social brain’during fearful face-processing in Asperger Syndrome. Neuropsychologia, 45(1), 2-14. [DOI:10.1016/j.neuropsychologia.2006.04.014] [PMID]
Assaf, M., Jagannathan, K., Calhoun, V. D., Miller, L., Stevens, M. C., & Sahl, R., et al. (2010). Abnormal functional connectivity of default mode sub-networks in autism spectrum disorder patients. NeuroImage, 53(1), 247-256. [PMID] [PMCID]
Beauregard, M., Leroux, J. M., Bergman, S., Arzoumanian, Y., Beaudoin, G., & Bourgouin, P., et al. (1998). The functional neuroanatomy of major depression: An fMRI study using an emotional activation paradigm. Neuroreport, 9(14), 3253-3258. [PMID]
Bethlehem, R. A. I., Romero-Garcia, R., Mak, E., Bullmore, E. T., & Baron-Cohen, S. (2017). Structural covariance networks in children with autism or ADHD. Cerebral Cortex, 27(8), 4267-4276. [PMID] [PMCID]
Bi, X. A., Wang, Y., Shu, Q., Sun, Q., & Xu, Q. (2018). Classification of autism spectrum disorder using random support vector machine cluster. Frontiers in Genetics, 9, 18. [DOI:10.3389/fgene.2018.00018] [PMID] [PMCID]
Bogdashina, O. B. (2016). Synaesthesia in autism. Autism and Developmental Disorders, 14(3), 21-31. [Link]
Bonda, E., Petrides, M., Ostry, D., & Evans, A. (1996). Specific involvement of human parietal systems and the amygdala in the perception of biological motion. The Journal of Neuroscience: The Official Journal of the Society for Neuroscience, 16(11), 3737–3744. [PMID] [PMCID]
Butz, M., Wörgötter, F., & van Ooyen, A. (2009). Activity-dependent structural plasticity. Brain Research Reviews, 60(2), 287-305. [DOI:10.1016/j.brainresrev.2008.12.023] [PMID]
Buxhoeveden, D. P., Semendeferi, K., Buckwalter, J., Schenker, N., Switzer, R., & Courchesne, E. (2006). Reduced minicolumns in the frontal cortex of patients with autism. Neuropathology and Applied Neurobiology, 32(5), 483-491. [DOI:10.1111/j.1365-2990.2006.00745.x] [PMID]
Cardon, G. J., Hepburn, S., & Rojas, D. C. (2017). Structural covariance of sensory networks, the cerebellum, and amygdala in autism spectrum disorder. Frontiers in Neurology, 8, 615. [PMID] [PMCID]
Carlisi, C. O., Norman, L. J., Lukito, S. S., Radua, J., Mataix-Cols, D., & Rubia, K. (2017). Comparative multimodal meta-analysis of structural and functional brain abnormalities in autism spectrum disorder and obsessive-compulsive disorder. Biological Psychiatry, 82(2), 83-102. [DOI:10.1016/j.biopsych.2016.10.006] [PMID]
Cauda, F., Costa, T., Palermo, S., D'Agata, F., Diano, M., & Bianco, F., et al. (2014). Concordance of white matter and gray matter abnormalities in autism spectrum disorders: A voxel‐based meta‐analysis study. Human Brain Mapping, 35(5), 2073-2098. [PMID] [PMCID]
Cauda, F., D'Agata, F., Sacco, K., Duca, S., Geminiani, G., & Vercelli, A. (2011). Functional connectivity of the insula in the resting brain. NeuroImage, 55(1), 8-23. [DOI:10.1016/j.neuroimage.2010.11.049] [PMID]
Cauda, F., Geda, E., Sacco, K., D'Agata, F., Duca, S., & Geminiani, G., et al. (2011). Grey matter abnormality in autism spectrum disorder: an activation likelihood estimation meta-analysis study. Journal of Neurology, Neurosurgery, and Psychiatry, 82(12), 1304–1313. [PMID]
Chen, Z. J., He, Y., Rosa-Neto, P., Germann, J., & Evans, A. C. (2008). Revealing modular architecture of human brain structural networks by using cortical thickness from MRI. Cerebral Cortex, 18(10), 2374-2381. [PMID] [PMCID]
Courchesne, E., & Pierce, K. (2005). Why the frontal cortex in autism might be talking only to itself: Local over-connectivity but long-distance disconnection. Current Opinion in Neurobiology, 15(2), 225-230. [PMID]
Courchesne, E., Pierce, K., Schumann, C. M., Redcay, E., Buckwalter, J. A., & Kennedy, D. P., et al. (2007). Mapping early brain development in autism. Neuron, 56(2), 399-413. [PMID]
Courchesne, E., Redcay, E., Morgan, J. T., & Kennedy, D. P. (2005). Autism at the beginning: Microstructural and growth abnormalities underlying the cognitive and behavioral phenotype of autism. Development and Psychopathology, 17(3), 577-597. [PMID]
Courchesne, E., Yeung-Courchesne, R., Press, G. A., Hesselink, J. R., & Jernigan, T. L. (1988). Hypoplasia of cerebellar vermal lobules VI and VII in autism. The New England Journal of Medicine, 318(21), 1349–1354. [PMID]
Cox, R. W. (1996). AFNI: software for analysis and visualization of functional magnetic resonance neuroimages. Computers and Biomedical Research, 29(3), 162-173. [DOI:10.1006/cbmr.1996.0014] [PMID]
D'Mello, A. M., Moore, D. M., Crocetti, D., Mostofsky, S. H., & Stoodley, C. J. (2016). Cerebellar gray matter differentiates children with early language delay in autism. Autism Research, 9(11), 1191–1204. [PMID]
Damoiseaux, J. S., & Greicius, M. D. (2009). Greater than the sum of its parts: A review of studies combining structural connectivity and resting-state functional connectivity. Brain Structure and Function, 213(6), 525-533. [DOI:10.1007/s00429-009-0208-6] [PMID]
de Giambattista, C., Ventura, P., Trerotoli, P., Margari, M., Palumbi, R., & Margari, L. (2019). Subtyping the autism spectrum disorder: Comparison of children with high functioning autism and Asperger syndrome. Journal of Autism and Developmental Disorders, 49(1), 138- 150. [PMID]
Dickstein, D. P., Pescosolido, M. F., Reidy, B. L., Galvan, T., Kim, K. L., & Seymour, K. E., et al. (2013). Developmental meta-analysis of the functional neural correlates of autism spectrum disorders. Journal of the American Academy of Child & Adolescent Psychiatry, 52(3), 279- 289. e216. [PMID] [PMCID]
Duan, X., Wang, R., Xiao, J., Li, Y., Huang, X., & Guo, X., et al. (2020). Subcortical structural covariance in young children with autism spectrum disorder. Progress in Neuro-Psychopharmacology and Biological Psychiatry, 99, 109874. [PMID]
Dziobek, I., Bahnemann, M., Convit, A., & Heekeren, H. R. (2010). The role of the fusiform-amygdala system in the pathophysiology of autism. Archives of General Psychiatry, 67(4), 397-405. [PMID]
Ebisch, S. J., Gallese, V., Willems, R. M., Mantini, D., Groen, W. B., & Romani, G. L., et al. (2011). Altered intrinsic functional connectivity of anterior and posterior insula regions in high‐functioning participants with autism spectrum disorder. Human Brain Mapping, 32(7), 1013-1028. [PMID] [PMCID]
Ecker, C., Andrews, D., Dell’Acqua, F., Daly, E., Murphy, C., Catani, M., et al. (2016). Relationship between cortical gyrification, white matter connectivity, and autism spectrum disorder. Cerebral Cortex, 26(7), 3297-3309. [DOI:10.1093/cercor/bhw098] [PMID] [PMCID]
Ecker, C., Schmeisser, M. J., Loth, E., & Murphy, D. G. (2017). Neuroanatomy and neuropathology of autism spectrum disorder in humans. In M. Schmeisser, & T. Boeckers (eds.), Advances in anatomy, embryology and cell biology (pp. 27-48): Cham: Springer. [DOI:10.1007/978-3-319-52498-6_2]
Ernst, M., Zametkin, A. J., Matochik, J. A., Pascualvaca, D., & Cohen, R. M. (1997). Low medial prefrontal dopaminergic activity in autistic children. The Lancet, 350(9078), 638. [DOI:10.1016/S0140-6736(05)63326-0] [PMID]
Etkin, A., Egner, T., & Kalisch, R. (2011). Emotional processing in anterior cingulate and medial prefrontal cortex. Trends in Cognitive Sciences, 15(2), 85-93. [PMID] [PMCID]
Evans, A. C. (2013). Networks of anatomical covariance. Neuroimage, 80, 489-504. [DOI:10.1016/j.neuroimage.2013.05.054] [PMID]
Eyler, L. T., Prom-Wormley, E., Fennema-Notestine, C., Panizzon, M. S., Neale, M. C., & Jernigan, T. L., et al. (2011). Genetic patterns of correlation among subcortical volumes in humans: Results from a magnetic resonance imaging twin study. Human Brain Mapping, 32(4), 641- 653. [DOI:10.1002/hbm.21054] [PMID] [PMCID]
Faridi, F., & Khosrowabadi, R. (2017). Behavioral, cognitive and neural markers of Asperger syndrome. Basic and Clinical Neuroscience, 8(5), 349–359. [PMID] [PMCID]
Flynn, F. G. (1999). Anatomy of the insula functional and clinical correlates. Aphasiology, 13(1), 55- 78. [DOI:10.1080/026870399402325]
Gilchrist, A., Green, J., Cox, A., Burton, D., Rutter, M., & Le Couteur, A. (2001). Development and current functioning in adolescents with Asperger syndrome: A comparative study. Journal of Child Psychology and Psychiatry, 42(2), 227-240. [DOI:10.1111/1469-7610.00714] [PMID]
Groen, W., Teluij, M., Buitelaar, J., & Tendolkar, I. (2010). Amygdala and hippocampus enlargement during adolescence in autism. Journal of the American Academy of Child & Adolescent Psychiatry, 49(6), 552-560. [PMID]
Guo, X., Duan, X., Long, Z., Chen, H., Wang, Y., & Zheng, J., et al. (2016). Decreased amygdala functional connectivity in adolescents with autism: A resting-state fMRI study. Psychiatry Research: Neuroimaging, 257, 47-56. [DOI:10.1016/j.pscychresns.2016.10.005] [PMID]
Guo, X., Duan, X., Suckling, J., Chen, H., Liao, W., & Cui, Q., et al. (2019). Partially impaired functional connectivity states between right anterior insula and default mode network in autism spectrum disorder. Human Brain Mapping, 40(4), 1264-1275. [PMID] [PMCID]
He, Y., Chen, Z. J., & Evans, A. C. (2007). Small-world anatomical networks in the human brain revealed by cortical thickness from MRI. Cerebral Cortex, 17(10), 2407-2419. [PMID]
Hollander, E., Anagnostou, E., Chaplin, W., Esposito, K., Haznedar, M. M., & Licalzi, E., et al. (2005). Striatal volume on magnetic resonance imaging and repetitive behaviors in autism. Biological Psychiatry, 58(3), 226-232. [DOI:10.1016/j.biopsych.2005.03.040] [PMID]
Hyde, K. L., Samson, F., Evans, A. C., & Mottron, L. (2010).Neuroanatomical differences in brain areas implicated in perceptual and other core features of autism revealed by cortical thickness analysis and voxel‐based morphometry. Human Brain Mapping, 31(4), 556-566. [DOI:10.1002/hbm.20887] [PMID] [PMCID]
Jao Keehn, R. J., Nair, S., Pueschel, E. B., Linke, A. C., Fishman, I., & Müller, R. A. (2019). Atypical local and distal patterns of occipito-frontal functional connectivity are related to symptom severity in autism. Cerebral Cortex, 29(8), 3319-3330. [PMID] [PMCID]
Jenkinson, M., Beckmann, C. F., Behrens, T. E., Woolrich, M. W., & Smith, S. M. (2012). FSL. Neuroimage, 62(2), 782-790. [DOI:10.1016/j.neuroimage.2011.09.015] [PMID]
Jin, X., & Costa, R. M. (2015). Shaping action sequences in basal ganglia circuits. Current Opinion in Neurobiology, 33, 188-196. [PMID] [PMCID]
Jones, E. G. (2009). Synchrony in the interconnected circuitry of the thalamus and cerebral cortex. Annals of the New York Academy of Sciences, 1157, 10-23. [DOI:10.1111/j.1749-6632.2009.04534.x] [PMID]
Kana, R. K., Libero, L. E., & Moore, M. S. (2011). Disrupted cortical connectivity theory as an explanatory model for autism spectrum disorders. Physics of Life Reviews, 8(4), 410-437. [DOI:10.1016/j.plrev.2011.10.001] [PMID]
Kato, H., & Izumiyama, M. (2015). Impaired motor control due to proprioceptive sensory loss in a patient with cerebral infarction localized to the postcentral gyrus. Journal of Rehabilitation Medicine, 47(2), 187-190. [DOI:10.2340/16501977-1900] [PMID]
Kennedy, D. P., & Courchesne, E. (2008). The intrinsic functional organization of the brain is altered in autism. NeuroImage, 39(4), 1877-1885. [DOI:10.1016/j.neuroimage.2007.10.052] [PMID]
Keown, C. L., Shih, P., Nair, A., Peterson, N., Mulvey, M. E., & Müller, R. A. (2013). Local functional overconnectivity in posterior brain regions is associated with symptom severity in autism spectrum disorders. Cell Reports, 5(3), 567-572. [DOI:10.1016/j.celrep.2013.10.003] [PMID] [PMCID]
Yu, K. K., Cheung, C., Chua, S. E., & McAlonan, G. M. (2011). Can Asperger syndrome be distinguished from autism? An anatomic likelihood meta-analysis of MRI studies. Journal of Psychiatry & Neuroscience: JPN, 36(6), 412-421. [DOI:10.1503/jpn.100138] [PMID] [PMCID]
Khosrowabadi, R., Quek, C., Ang, K. K., Wahab, A., & Chen, S.-H. A. (2015). Dynamic screening of autistic children in various mental states using pattern of connectivity between brain regions. Applied Soft Computing, 32, 335-346. [DOI:10.1016/j.asoc.2015.03.030]
Kikuchi, M., Shitamichi, K., Yoshimura, Y., Ueno, S., Hiraishi, H., & Hirosawa, T., et al. (2013). Altered brain connectivity in 3-to 7-year-old children with autism spectrum disorder. NeuroImage. Clinical, 2, 394–401. [PMID]
Kim, J. A., Szatmari, P., Bryson, S. E., Streiner, D. L., & Wilson, F. J. (2000). The prevalence of anxiety and mood problems among children with autism and Asperger syndrome. Autism, 4(2), 117- 132. [DOI:10.1177/1362361300004002002]
Kobayashi, A., Yokota, S., Takeuchi, H., Asano, K., Asano, M., & Sassa, Y., et al. (2020). Increased grey matter volume of the right superior temporal gyrus in healthy children with autistic cognitive style: A VBM study. Brain and Cognition, 139, 105514. [DOI:10.1016/j.bandc.2019.105514] [PMID]
Koch, M., & Bubser, M. (1994). Deficient sensorimotor gating after 6‐hydroxydopamine lesion of the rat medial prefrontal cortex is reversed by haloperidol. European Journal of Neuroscience, 6(12), 1837-1845. [DOI:10.1111/j.1460-9568.1994.tb00576.x] [PMID]
Kohli, J. S. (2017). Cerebral gyrification and the cortical architecture of Autism Spectrum Disorder (ASD). San Diego: San Diego State University.
Kwon, H., Ow, A. W., Pedatella, K. E., Lotspeich, L. J., & Reiss, A. L. (2004). Voxel-based morphometry elucidates structural neuroanatomy of high-functioning autism and Asperger syndrome. Developmental Medicine & Child Neurology, 46(11), 760-764. [PMID]
Laidi, C., Boisgontier, J., Chakravarty, M. M., Hotier, S., d'Albis, M. A., & Mangin, J. F., et al. (2017). Cerebellar anatomical alterations and attention to eyes in autism. Scientific Reports, 7(1), 12008. [PMID] [PMCID]
Liu, J., Yao, L., Zhang, W., Xiao, Y., Liu, L., & Gao, X., et al. (2017). Gray matter abnormalities in pediatric autism spectrum disorder: A meta-analysis with signed differential mapping. European Child & Adolescent Psychiatry, 26(8), 933–945. [DOI:10.1007/s00787-017-0964-4] [PMID]
Mazefsky, C. A., & Oswald, D. P. (2007). Emotion perception in Asperger’s syndrome and high- functioning autism: The importance of diagnostic criteria and cue intensity. Journal of Autism and Developmental Disorders, 37(6), 1086-1095. [PMID]
McAlonan, G. M., Cheung, V., Cheung, C., Suckling, J., Lam, G. Y., & Tai, K. S., et al. (2005). Mapping the brain in autism. A voxel-based MRI study of volumetric differences and intercorrelations in autism. Brain, 128(Pt 2), 268–276. [DOI:10.1093/brain/awh332] [PMID]
McAlonan, G. M., Suckling, J., Wong, N., Cheung, V., Lienenkaemper, N., & Cheung, C., et al. (2008). Distinct patterns of grey matter abnormality in high‐functioning autism and Asperger’s syndrome. Journal of Child Psychology and Psychiatry, and Allied Disciplines, 49(12), 1287–1295. [DOI:10.1111/j.1469-7610.2008.01933.x] [PMID]
McKavanagh, R., Buckley, E., & Chance, S. A. (2015). Wider minicolumns in autism: A neural basis for altered processing? Brain, 138(Pt 7), 2034–2045. [DOI:10.1093/brain/awv110] [PMID]
Mechelli, A., Friston, K. J., Frackowiak, R. S., & Price, C. J. (2005). Structural covariance in the human cortex. The Journal of Neuroscience : The Official Journal of The Society for Neuroscience, 25(36), 8303–8310. [DOI:10.1523/JNEUROSCI.0357-05.2005] [PMID]
Montgomery, C. B., Allison, C., Lai, M. C., Cassidy, S., Langdon, P. E., & Baron-Cohen, S. (2016). Do adults with high functioning autism or Asperger Syndrome differ in empathy and emotion recognition? Journal of Autism and Developmental Disorders, 46(6), 1931-1940. [DOI:10.1007/s10803-016-2698-4] [PMID] [PMCID]
Mundy, P. (2003). Annotation: The neural basis of social impairments in autism: the role of the dorsal medial‐frontal cortex and anterior cingulate system. Journal of Child Psychology and Psychiatry, 44(6), 793-809. [DOI:10.1111/1469-7610.00165] [PMID]
Nair, A., Keown, C. L., Datko, M., Shih, P., Keehn, B., & Müller, R. A. (2014). Impact of methodological variables on functional connectivity findings in autism spectrum disorders. Human Brain Mapping, 35(8), 4035-4048. [DOI:10.1002/hbm.22456] [PMID] [PMCID]
Nelson, S. M., Dosenbach, N. U., Cohen, A. L., Wheeler, M. E., Schlaggar, B. L., & Petersen, S. E. (2010). Role of the anterior insula in task-level control and focal attention. Brain Structure and Function, 214(5-6), 669-680. [DOI:10.1007/s00429-010-0260-2] [PMID] [PMCID]
Nielsen, J. A., Zielinski, B. A., Fletcher, P. T., Alexander, A. L., Lange, N., & Bigler, E. D.,et al. (2014). Abnormal lateralization of functional connectivity between language and default mode regions in autism. Molecular Autism, 5(1), 8. [PMID]
Ortiz-Mantilla, S., Choe, M. S., Flax, J., Grant, P. E., & Benasich, A. A. (2010). Associations between the size of the amygdala in infancy and language abilities during the preschool years in normally developing children. NeuroImage, 49(3), 2791-2799. [DOI:10.1016/j.neuroimage.2009.10.029] [PMID]
Ozonoff, S., South, M., & Miller, J. N. (2000). DSM-IV-defined Asperger syndrome: Cognitive, behavioral and early history differentiation from high-functioning autism. Autism, 4(1), 29- 46. [DOI:10.1177/1362361300041003]
Paulus, M. P., Feinstein, J. S., Leland, D., & Simmons, A. N. (2005). Superior temporal gyrus and insula provide response and outcome-dependent information during assessment and action selection in a decision-making situation. NeuroImage, 25(2), 607-615. [PMID]
Riva, D., Annunziata, S., Contarino, V., Erbetta, A., Aquino, D., & Bulgheroni, S. (2013). Gray matter reduction in the vermis and CRUS-II is associated with social and interaction deficits in low-functioning children with autistic spectrum disorders: A VBM-DARTEL Study. Cerebellum (London, England), 12(5), 676–685. [DOI:10.1007/s12311-013-0469-8] [PMID]
Romero-Garcia, R., Whitaker, K. J., Váša, F., Seidlitz, J., Shinn, M., & Fonagy, P., et al. (2018). Structural covariance networks are coupled to expression of genes enriched in supragranular layers of the human cortex. NeuroImage, 171, 256–267. [DOI:10.1101/163758]
Rossion, B., Caldara, R., Seghier, M., Schuller, A. M., Lazeyras, F., & Mayer, E. (2003). A network of occipito‐temporal face‐sensitive areas besides the right middle fusiform gyrus is necessary for normal face processing. Brain, 126(11), 2381-2395. [DOI:10.1093/brain/awg241] [PMID]
Rudie, J. D., Brown, J. A., Beck-Pancer, D., Hernandez, L. M., Dennis, E. L., & Thompson, P. M., et al. (2012). Altered functional and structural brain network organization in autism. NeuroImage. Clinical, 2, 79–94. [PMID] [PMCID]
Rudie, J. D., Shehzad, Z., Hernandez, L. M., Colich, N. L., Bookheimer, S. Y., & Iacoboni, M., et al. (2012). Reduced functional integration and segregation of distributed neural systems underlying social and emotional information processing in autism spectrum disorders. Cerebral Cortex, 22(5), 1025-1037. [PMID] [PMCID]
Saarela, M. V., Hlushchuk, Y., Williams, A. C., Schürmann, M., Kalso, E., & Hari, R. (2007). The compassionate brain: Humans detect intensity of pain from another’s face. Cerebral Cortex, 17(1), 230-237. [DOI:10.1093/cercor/bhj141] [PMID]
Sadeghi, M., Khosrowabadi, R., Bakouie, F., Mahdavi, H., Eslahchi, C., & Pouretemad, H. (2017). Screening of autism based on task-free fMRI using graph theoretical approach. Psychiatry Research: Neuroimaging, 263, 48-56. [DOI:10.1016/j.pscychresns.2017.02.004] [PMID]
Sadeghi, M., Khosrowabadi, R., Bakouie, F., Mahdavi, H., Eslahchi, C., & Pouretemad, H. (2017). Screening of autism based on task-free fMRI using graph theoretical approach. Psychiatry Research. Neuroimaging, 263, 48–56. [DOI:10.1016/j.pscychresns.2017.02.004] [PMID]
Sahyoun, C. P., Belliveau, J. W., Soulières, I., Schwartz, S., & Mody, M. (2010). Neuroimaging of the functional and structural networks underlying visuospatial vs. linguistic reasoning in high- functioning autism. Neuropsychologia, 48(1), 86–95. [PMID]
Seminowicz, D. A., & Davis, K. D. (2007). Interactions of pain intensity and cognitive load: The brain stays on task. Cerebral Cortex, 17(6), 1412-1422. [DOI:10.1093/cercor/bhl052] [PMID]
Sharda, M., Khundrakpam, B. S., Evans, A. C., & Singh, N. C. (2016). Disruption of structural covariance networks for language in autism is modulated by verbal ability. Brain Structure and Function, 221(2), 1017-1032. [DOI:10.1007/s00429-014-0953-z] [PMID]
Soriano-Mas, C., Harrison, B. J., Pujol, J., López-Solà, M., Hernández-Ribas, R., & Alonso, P., et al. (2013). Structural covariance of the neostriatum with regional gray matter volumes. Brain Structure and Function, 218(3), 697-709. [DOI:10.1007/s00429-012-0422-5] [PMID]
Stacey, D., Redlich, R., Büschel, A., Opel, N., Grotegerd, D., & Zaremba, D., et al. (2017). TNF receptors 1 and 2 exert distinct region‐specific effects on striatal and hippocampal grey matter volumes (VBM) in healthy adults. Genes, Brain and Behavior, 16(3), 352-360. [DOI:10.1111/gbb.12318] [PMID]
Sui, J., Huster, R., Yu, Q., Segall, J. M., & Calhoun, V. D. (2014). Function-structure associations of the brain: Evidence from multimodal connectivity and covariance studies. NeuroImage, 102 Pt 1, 11–23. [PMID] [PMCID]
Szatmari, P., Archer, L., Fisman, S., Streiner, D. L., & Wilson, F. (1995). Asperger’s syndrome and autism: Differences in behavior, cognition, and adaptive functioning. Journal of the American Academy of Child & Adolescent Psychiatry, 34(12), 1662-1671. [PMID]
Taylor, K. S., Seminowicz, D. A., & Davis, K. D. (2009). Two systems of resting state connectivity between the insula and cingulate cortex. Human Brain Mapping, 30(9), 2731-2745. [PMID] [PMCID]
Tomasi, D., & Volkow, N. D. (2012). Laterality patterns of brain functional connectivity: Gender effects. Cerebral Cortex, 22(6), 1455-1462. [PMID]
Tomasi, D., & Volkow, N. D. (2019). Reduced local and increased long-range functional connectivity of the thalamus in autism spectrum disorder. Cerebral Cortex, 29(2), 573-585. [DOI:10.1093/cercor/bhx340] [PMID] [PMCID]
Uddin, L. Q., Menon, V., Young, C. B., Ryali, S., Chen, T., & Khouzam, A., et al.(2011). Multivariate searchlight classification of structural magnetic resonance imaging in children and adolescents with autism. Biological Psychiatry, 70(9), 833-841. [PMID]
Van Overwalle, F., & Mariën, P. (2016). Functional connectivity between the cerebrum and cerebellum in social cognition: A multi-study analysis. NeuroImage, 124(Pt A), 248–255. [PMID]
van Rooij, D., Anagnostou, E., Arango, C., Auzias, G., Behrmann, M., & Busatto, G. F., et al. (2018). Cortical and subcortical brain morphometry differences between patients with autism spectrum disorder and healthy individuals across the lifespan: results from the ENIGMA ASD Working Group. The American Journal of Psychiatry, 175(4), 359–369. [PMID] [PMCID]
Vértes, P. E., Alexander-Bloch, A. F., Gogtay, N., Giedd, J. N., Rapoport, J. L., & Bullmore, E. T. (2012). Simple models of human brain functional networks. Proceedings of the National Academy of Sciences, 109(15), 5868-5873. [PMID] [PMCID]
Via, E., Radua, J., Cardoner, N., Happé, F., & Mataix-Cols, D. (2011). Meta-analysis of gray matter abnormalities in autism spectrum disorder: Should Asperger disorder be subsumed under a broader umbrella of autistic spectrum disorder? Archives of General Psychiatry, 68(4), 409- 418. [PMID]
Vissers, M. E., Cohen, M. X., & Geurts, H. M. (2012). Brain connectivity and high functioning autism: A promising path of research that needs refined models, methodological convergence, and stronger behavioral links. Neuroscience & Biobehavioral Reviews, 36(1), 604-625. [DOI:10.1016/j.neubiorev.2011.09.003] [PMID]
Volkmar, F. R., Klin, A., & Pauls, D. (1998). Nosological and genetic aspects of Asperger syndrome. Journal of Autism and Developmental Disorders, 28(5), 457-463. [Link]
Yang, D. Y., Beam, D., Pelphrey, K. A., Abdullahi, S., & Jou, R. J. (2016). Cortical morphological markers in children with autism: A structural magnetic resonance imaging study of thickness, area, volume, and gyrification. Molecular Autism, 7, 11. [DOI:10.1186/s13229-016-0076-x] [PMID] [PMCID]
Zuo, C., Wang, D., Tao, F., & Wang, Y. (2019). Changes in the development of subcortical structures in autism spectrum disorder. Neuroreport, 30(16), 1062–1067. [PMID]